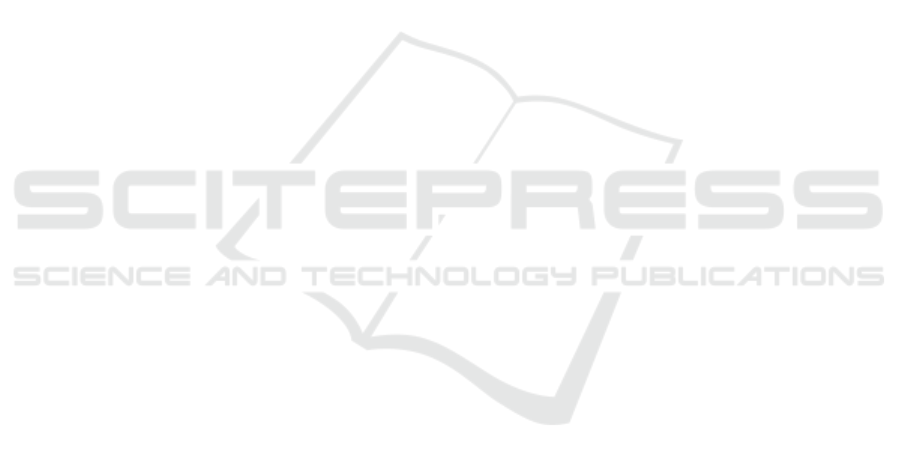
REFERENCES
Aljedaani, W., Rustam, F., Mkaouer, M. W., Ghallab, A.,
Rupapara, V., Washington, P. B., Lee, E., and Ashraf,
I. (2022). Sentiment analysis on twitter data inte-
grating textblob and deep learning models: the case
of us airline industry. Knowledge-Based Systems,
255:109780.
Dien, T. T., Thanh-Hai, N., and Thai-Nghe, N. (2022). An
approach for learning resource recommendation using
deep matrix factorization. Journal of Information and
Telecommunication, 6(4):1–18.
Fraihat, S. and Shambour, Q. (2015). A framework of se-
mantic recommender system for e-learning. Journal
of Software, 10(3):317–330.
Guo, J., Ji, W., Yuan, J., and Wang, X. (2022). Multi-
channel orthogonal decomposition attention network
for sequential recommendation. In Pacific-Asia Con-
ference on Knowledge Discovery and Data Mining,
pages 288–300. Springer.
Guo, T., Wen, Y., Wang, F., and Hou, J. (2019). Learning re-
source recommendation based on generalized matrix
factorization and long short-term memory model. In
2019 IEEE International Conference on Cloud Com-
puting Technology and Science (CloudCom), pages
217–222. IEEE.
Hermawan, D. (2021). The rise of e-learning in covid-19
pandemic in private university: challenges and oppor-
tunities. IJORER: International Journal of Recent Ed-
ucational Research, 2(1):86–95.
Kaminskas, M. and Bridge, D. (2016). Diversity, serendip-
ity, novelty, and coverage: a survey and empiri-
cal analysis of beyond-accuracy objectives in recom-
mender systems. ACM Transactions on Interactive In-
telligent Systems (TiiS), 7(1):1–42.
Kotkov, D., Veijalainen, J., and Wang, S. (2020). How does
serendipity affect diversity in recommender systems?
a serendipity-oriented greedy algorithm. Computing,
102(2):393–411.
Kotkov, D., Wang, S., and Veijalainen, J. (2016). A survey
of serendipity in recommender systems. Knowledge-
Based Systems, 111:180–192.
Kundu, S. S., Sarkar, D., Jana, P., and Kole, D. K. (2021).
Personalization in education using recommendation
system: An overview. Computational Intelligence in
Digital Pedagogy, pages 85–111.
Li, X., Jiang, W., Chen, W., Wu, J., and Wang, G. (2019).
Haes: A new hybrid approach for movie recommen-
dation with elastic serendipity. In Proceedings of the
28th ACM International Conference on Information
and Knowledge Management, pages 1503–1512.
Menk, A., Sebastia, L., and Ferreira, R. (2017). Cu-
rumim: A serendipitous recommender system for
tourism based on human curiosity. In 2017 IEEE 29th
International Conference on Tools with Artificial In-
telligence (ICTAI), pages 788–795. IEEE.
Mihaescu, M. C. and Popescu, P. S. (2021). Review on
publicly available datasets for educational data min-
ing. Wiley Interdisciplinary Reviews: Data Mining
and Knowledge Discovery, 11(3):e1403.
Nguyen, T. T., Hui, P.-M., Harper, F. M., Terveen, L., and
Konstan, J. A. (2014). Exploring the filter bubble: the
effect of using recommender systems on content di-
versity. In Proceedings of the 23rd international con-
ference on World wide web, pages 677–686.
Pardos, Z. A. and Jiang, W. (2020). Designing for serendip-
ity in a university course recommendation system. In
Proceedings of the tenth international conference on
learning analytics & knowledge, pages 350–359.
Peinelt, N., Nguyen, D., and Liakata, M. (2020). tbert:
Topic models and bert joining forces for semantic sim-
ilarity detection. In Proceedings of the 58th annual
meeting of the association for computational linguis-
tics, pages 7047–7055.
Taramigkou, M., Bothos, E., Christidis, K., Apostolou, D.,
and Mentzas, G. (2013). Escape the bubble: Guided
exploration of music preferences for serendipity and
novelty. In Proceedings of the 7th ACM conference on
Recommender systems, pages 335–338.
Troudi, A., Ghorbel, L., Amel Zayani, C., Jamoussi, S., and
Amous, I. (2021). Mder: Multi-dimensional event rec-
ommendation in social media context. The Computer
Journal, 64(3):369–382.
Vora, M., Barvaliya, H., Balar, P., and Jagtap, N. (2020).
E-learning systems and moocs-a review. Int. J. Res.
Appl. Sci. Eng. Technol, 8(9):636–641.
Zhang, M., Yang, Y., Abbas, R., Deng, K., Li, J., and Zhang,
B. (2021). Snpr: A serendipity-oriented next poi rec-
ommendation model. In Proceedings of the 30th ACM
International Conference on Information & Knowl-
edge Management, pages 2568–2577.
Zhang, Q., Wang, S., Lu, W., Feng, C., Peng, X., and
Wang, Q. (2022). Rethinking adjacent dependency in
session-based recommendations. In Pacific-Asia Con-
ference on Knowledge Discovery and Data Mining,
pages 301–313. Springer.
Ziarani, R. J. and Ravanmehr, R. (2021a). Deep neural
network approach for a serendipity-oriented recom-
mendation system. Expert Systems with Applications,
185:115660.
Ziarani, R. J. and Ravanmehr, R. (2021b). Serendipity
in recommender systems: a systematic literature re-
view. Journal of Computer Science and Technology,
36(2):375–396.
EKM 2023 - 6th Special Session on Educational Knowledge Management
462