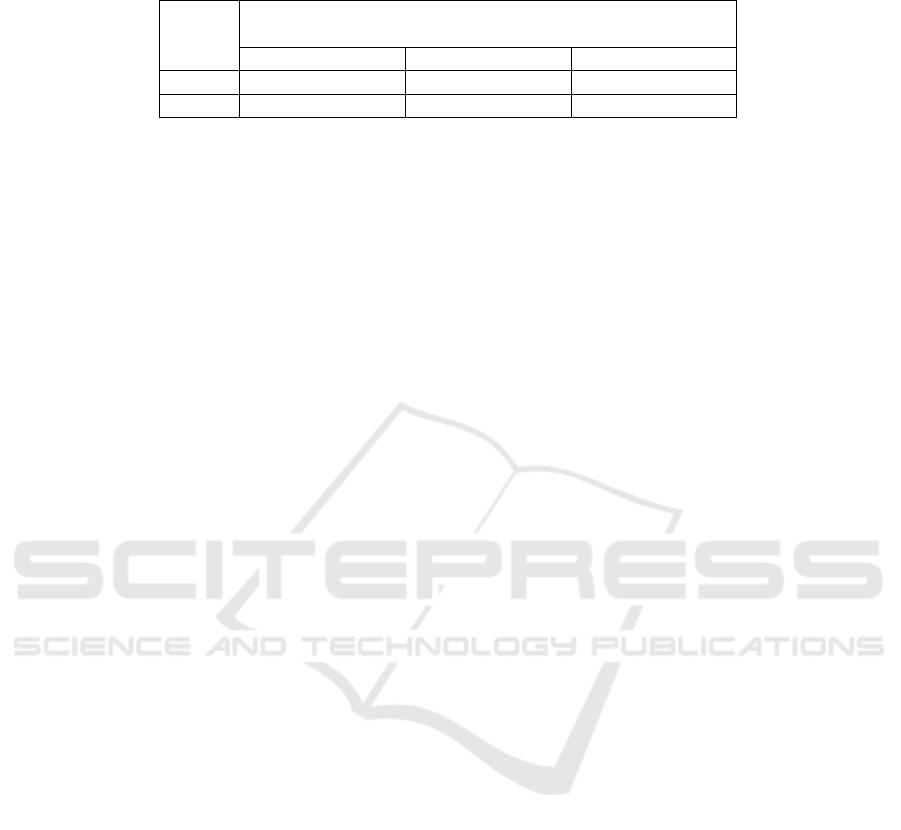
Table 2: Qualitative evaluation of the correctness of the feedback depending on the type of input and the respective category
of the message.
Input
type
Category
AnswerQuestion SentimentAnalysis DoActionRequest
Text 91% 87% 79%
Voice 88% 85% 71%
ACKNOWLEDGMENTS
This research was supported by European Union’s
Horizon Europe research and innovation programme
under grant agreement no. 101070455, project DYN-
ABIC. We also thank our game development industry
partners from Amber, Ubisoft, and Electronic Arts for
their feedback.
REFERENCES
Allouch, M., Azaria, A., and Azoulay, R. (2021). Conver-
sational agents: Goals, technologies, vision and chal-
lenges. Sensors, 21(24).
Baltezarevic, R., Baltezarevic, B., and Baltezarevic, V.
(2018). The video gaming industry (from play to rev-
enue). International Review, pages 71–76.
Banerjee, S. and Lavie, A. (2005). METEOR: An automatic
metric for MT evaluation with improved correlation
with human judgments. In Proceedings of the ACL
Workshop on Intrinsic and Extrinsic Evaluation Mea-
sures for Machine Translation and/or Summarization,
pages 65–72, Ann Arbor, Michigan. Association for
Computational Linguistics.
Brown, T., Mann, B., Ryder, N., Subbiah, M., Kaplan, J. D.,
Dhariwal, P., Neelakantan, A., Shyam, P., Sastry, G.,
Askell, A., Agarwal, S., Herbert-Voss, A., Krueger,
G., Henighan, T., Child, R., Ramesh, A., Ziegler, D.,
Wu, J., Winter, C., Hesse, C., Chen, M., Sigler, E.,
Litwin, M., Gray, S., Chess, B., Clark, J., Berner,
C., McCandlish, S., Radford, A., Sutskever, I., and
Amodei, D. (2020). Language models are few-shot
learners. In Larochelle, H., Ranzato, M., Hadsell, R.,
Balcan, M., and Lin, H., editors, Advances in Neu-
ral Information Processing Systems, volume 33, pages
1877–1901. Curran Associates, Inc.
Burtsev, M., Seliverstov, A., Airapetyan, R., Arkhipov,
M., Baymurzina, D., Bushkov, N., Gureenkova, O.,
Khakhulin, T., Kuratov, Y., Kuznetsov, D., Litinsky,
A., Logacheva, V., Lymar, A., Malykh, V., Petrov, M.,
Polulyakh, V., Pugachev, L., Sorokin, A., Vikhreva,
M., and Zaynutdinov, M. (2018). DeepPavlov: Open-
source library for dialogue systems. In Proceedings of
ACL 2018, System Demonstrations, pages 122–127,
Melbourne, Australia. Association for Computational
Linguistics.
Chatterjee, S. and Dietz, L. (2022). Bert-er: Query-specific
bert entity representations for entity ranking. In Pro-
ceedings of the 45th International ACM SIGIR Con-
ference on Research and Development in Information
Retrieval, SIGIR ’22, page 1466–1477.
Chen, A., Stanovsky, G., Singh, S., and Gardner, M.
(2019a). Evaluating question answering evaluation. In
Proceedings of the 2nd Workshop on Machine Read-
ing for Question Answering, pages 119–124, Hong
Kong, China. Association for Computational Linguis-
tics.
Chen, Q., Zhuo, Z., and Wang, W. (2019b). Bert for
joint intent classification and slot filling. ArXiv,
abs/1902.10909.
Devlin, J., Chang, M.-W., Lee, K., and Toutanova, K.
(2018). Bert: Pre-training of deep bidirectional trans-
formers for language understanding. arXiv preprint
arXiv:1810.04805.
Dingler, T., Kwasnicka, D., Wei, J., Gong, E., and Olden-
burg, B. (2021). The use and promise of conversa-
tional agents in digital health. Yearbook of Medical
Informatics, 30:191–199.
Hu, Y., Jing, X., Ko, Y., and Rayz, J. T. (2020). Mis-
spelling correction with pre-trained contextual lan-
guage model. 2020 IEEE 19th International Confer-
ence on Cognitive Informatics & Cognitive Comput-
ing (ICCI*CC), pages 144–149.
Kingma, D. P. and Ba, J. (2014). Adam: A method for
stochastic optimization.
Laranjo, L., Dunn, A. G., Tong, H. L., Kocaballi, A. B.,
Chen, J., Bashir, R., Surian, D., Gallego, B., Magrabi,
F., Lau, A. Y. S., and Coiera, E. (2018). Conversa-
tional agents in healthcare: a systematic review. Jour-
nal of the American Medical Informatics Association,
25(9):1248–1258.
Liu, Y., Ott, M., Goyal, N., Du, J., Joshi, M., Chen, D.,
Levy, O., Lewis, M., Zettlemoyer, L., and Stoyanov,
V. (2019). Roberta: A robustly optimized bert pre-
training approach. ArXiv, abs/1907.11692.
Miller, F. P., Vandome, A. F., and McBrewster, J. (2009).
Levenshtein Distance: Information Theory, Computer
Science, String (Computer Science), String Metric,
Damerau Levenshtein Distance, Spell Checker, Ham-
ming Distance. Alpha Press.
Munday, P. (2017). Duolingo. gamified learning through
translation. Journal of Spanish Language Teaching,
4(2):194–198.
Ogilvie, L., Prescott, J., and Carson, J. (2022). The use of
chatbots as supportive agents for people seeking help
with substance use disorder: A systematic review. Eu-
ropean Addiction Research, 28(6):405–418.
Conversational Agents for Simulation Applications and Video Games
35