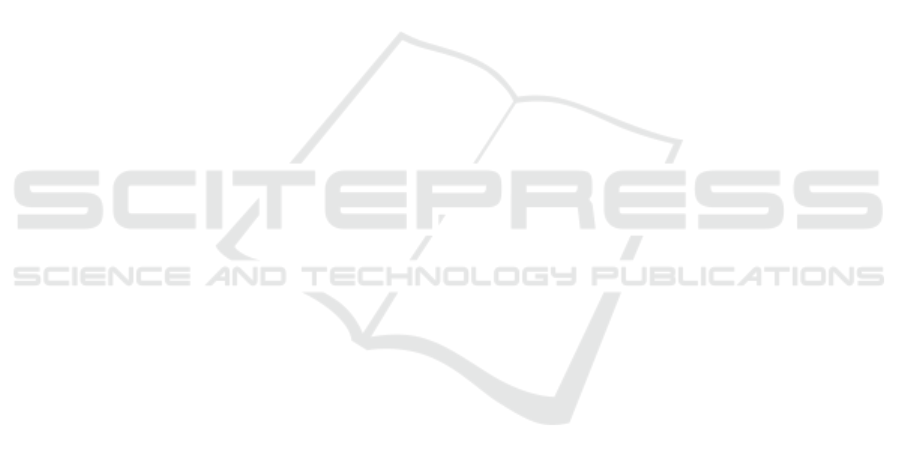
REFERENCES
Adake, N. (2022). Trust the process: An investigation into
astrophotography. In AIAA Southeastern Regional
Student Conference.
Aliakhmet, K. and James, A. P. (2019). Temporal g-
neighbor filtering for analog domain noise reduction
in astronomical videos. IEEE Trans. on Circuits and
Systems II: Express Briefs, 66(5):868–872.
Bracken, C. (2017). The deep-sky imaging primer. Deep-
sky Publishing.
Bradley, L., Sipocz, B., Robitaille, T., Tollerud, E., Deil, C.,
Vin
´
ıcius, Z., Barbary, K., G
¨
unther, H. M., Bostroem,
A., Droettboom, M., et al. (2016). Photutils: Photom-
etry tools. Astrophysics Source Code Library, pages
ascl–1609.
Feurer, M. and Hutter, F. (2019). Hyperparameter optimiza-
tion. In Automated Machine Learning, pages 3–33.
Springer, Cham.
He, K., Zhang, X., Ren, S., and Sun, J. (2016). Deep resid-
ual learning for image recognition. In IEEE CVPR
2016, pages 770–778.
Hogg, D. W., Blanton, M., Lang, D., Mierle, K., and
Roweis, S. (2008). Automated astrometry. In As-
tronomical Data Analysis Software and Systems XVII,
volume 394, page 27.
Hutter, F., Kotthoff, L., and Vanschoren, J. (2019). Au-
tomated machine learning: methods, systems, chal-
lenges. Springer Nature.
Jin, H., Chollet, F., Song, Q., and Hu, X. (2023). Autok-
eras: An automl library for deep learning. Journal of
Machine Learning Research, 24(6):1–6.
Jin, Z. and Finkel, H. (2020). Analyzing deep learning
model inferences for image classification using Open-
VINO. In IPDPSW 2020, pages 908–911.
Jung, A. (2019). Imgaug documentation. Readthedocs. io,
Jun, 25.
Kim, J., Nguyen, A.-D., and Lee, S. (2019). Deep cnn-
based blind image quality predictor. IEEE Trans. on
Neural Networks and Learning Systems, 30(1):11–24.
Leung, H. W. and Bovy, J. (2018). Deep learning of
multi-element abundances from high-resolution spec-
troscopic data. Monthly Notices of the Royal Astro-
nomical Society.
Mittal, A., Moorthy, A. K., and Bovik, A. C. (2012).
No-reference image quality assessment in the spa-
tial domain. IEEE Trans. on image processing,
21(12):4695–4708.
Olson, R. S. and Moore, J. H. (2016). TPOT: A tree-
based pipeline optimization tool for automating ma-
chine learning. In Workshop on automatic machine
learning, pages 66–74. PMLR.
Parisot, O., Bruneau, P., Hitzelberger, P., Krebs, G., and
Destruel, C. (2022). Improving accessibility for deep
sky observation. ERCIM News, 2022(130).
Parisot, O., Hitzelberger, P., Bruneau, P., Krebs, G., De-
struel, C., and Vandame, B. (2023). MILAN Sky Sur-
vey, a dataset of raw deep sky images captured during
one year with a Stellina automated telescope. Data in
Brief, 48:109133.
Parisot, O. and Tamisier, T. (2022). Applying genetic algo-
rithm and image quality assessment for reproducible
processing of low-light images. In IMPROVE 2022,
pages 189–194.
Raschka, S. (2018). Model evaluation, model selection, and
algorithm selection in machine learning.
Ravi, A. (2020). Nebula images.
Redfern, G. I. (2020). Astrophotography is Easy!: Basics
for Beginners. Springer.
Rigby, J., Perrin, M., McElwain, M., Kimble, R., Fried-
man, S., Lallo, M., Doyon, R., Feinberg, L., Fer-
ruit, P., Glasse, A., et al. (2022). Characterization of
jwst science performance from commissioning. arXiv
preprint arXiv:2207.05632.
Shorten, C. and Khoshgoftaar, T. M. (2019). A survey on
image data augmentation for deep learning. Journal
of big data, 6(1):1–48.
Talebi, H. and Milanfar, P. (2018). Nima: Neural im-
age assessment. IEEE Trans. on Image Processing,
27(8):3998–4011.
Tan, M. and Le, Q. (2019). Efficientnet: Rethinking model
scaling for convolutional neural networks. In ICML,
pages 6105–6114. PMLR.
Teimoorinia, H., Kavelaars, J., Gwyn, S., Durand, D., Rol-
ston, K., and Ouellette, A. (2020). Assessment of as-
tronomical images using combined machine-learning
models. The Astronomical Journal, 159(4):170.
Zack, M., Gannon, A., and McRoberts, J. (2018). Software
and apps to help the suburban astronomer. In Stargaz-
ing Under Suburban Skies, pages 341–350. Springer.
Zelaya, C. V. G. (2019). Towards explaining the effects
of data preprocessing on machine learning. In ICDE
2019, pages 2086–2090. IEEE.
Zhai, G. and Min, X. (2020). Perceptual image quality as-
sessment: a survey. Science China Information Sci-
ences, 63(11):1–52.
DATA 2023 - 12th International Conference on Data Science, Technology and Applications
286