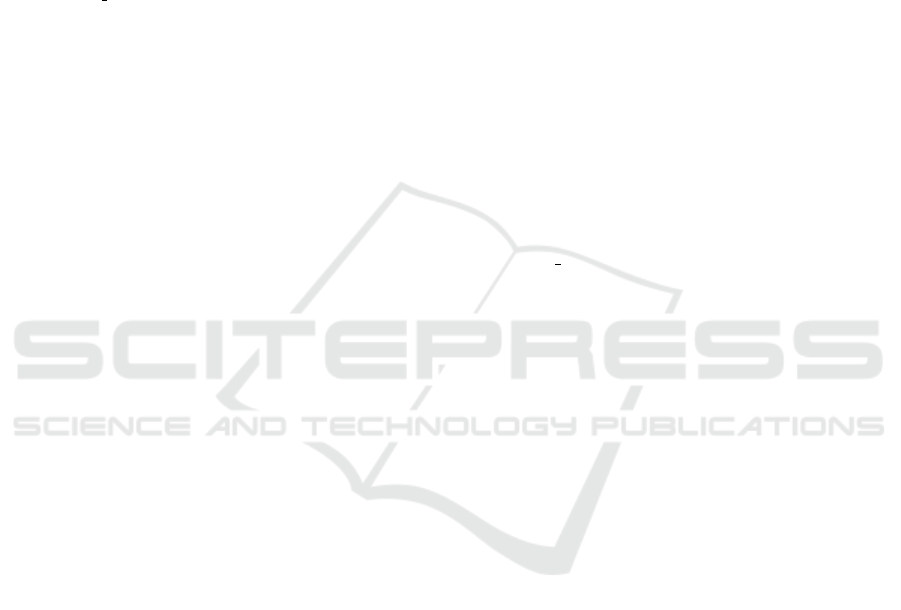
correlation coefficient. Noise reduction in speech pro-
cessing, pages 1–4.
Deeplearning4j (2023). Introduction to core deeplearning4j
concepts. https://deeplearning4j.konduit.ai/. [Online;
accessed 24-February-2023].
Deng, A. and Hooi, B. (2021). Graph neural network-based
anomaly detection in multivariate time series. In Pro-
ceedings of the AAAI conference on artificial intelli-
gence, volume 35, pages 4027–4035.
EarlyStopping (2023). What is early stopping? https://
deeplearning4j.konduit.ai/. [Online; accessed 24-
February-2023].
FONDRIEST
ENVIRONMENTAL (2023). Turner De-
signs C3 Submersible Fluorometer. https://www.fo
ndriest.com/turner-designs-c3-submersible-fluorom
eter.htm. [Online; accessed 23-February-2023].
Garg, N. (2013). Apache kafka. Packt Publishing Birming-
ham, UK.
Hochreiter, S. and Schmidhuber, J. (1997). Long short-term
memory. Neural computation, 9(8):1735–1780.
Hundman, K., Constantinou, V., Laporte, C., Colwell,
I., and Soderstrom, T. (2018). Detecting space-
craft anomalies using lstms and nonparametric dy-
namic thresholding. In Proceedings of the 24th ACM
SIGKDD international conference on knowledge dis-
covery & data mining, pages 387–395.
King, A. L., Sørensen, K., Jaccard, P., and Mengeot, C.
(2021). One ocean expedition - leg 2 - underway fer-
rybox. http://metadata.nmdc.no/metadata-api/landing
page/d508ffaf5ba3ba8618e25ad83686db75. [Online:
accessed 23-February-2023].
Knoblauch, F. M. (2022). Measurement of wave properties
with a custom built sensor system as part of the one
ocean expedition (2021-2023): Technical aspects and
post processing of field data. Master’s thesis.
Laxhammar, R. and Falkman, G. (2013). Online learning
and sequential anomaly detection in trajectories. IEEE
transactions on pattern analysis and machine intelli-
gence, 36(6):1158–1173.
Lee, M.-C. and Lin, J.-C. (2023). RePAD2: Real-time,
lightweight, and adaptive anomaly detection for open-
ended time series. In Proceedings of the 8th Inter-
national Conference on Internet of Things, Big Data
and Security - IoTBDS, pages 208–217. INSTICC,
SciTePress. arXiv preprint arXiv:2303.00409.
Lee, M.-C., Lin, J.-C., and Gan, E. G. (2020a). ReRe: A
lightweight real-time ready-to-go anomaly detection
approach for time series. In 2020 IEEE 44th Annual
Computers, Software, and Applications Conference
(COMPSAC), pages 322–327. IEEE. arXiv preprint
arXiv:2004.02319.
Lee, M.-C., Lin, J.-C., and Gran, E. G. (2020b). RePAD:
real-time proactive anomaly detection for time series.
In Advanced Information Networking and Applica-
tions: Proceedings of the 34th International Confer-
ence on Advanced Information Networking and Ap-
plications (AINA-2020), pages 1291–1302. Springer.
arXiv preprint arXiv:2001.08922.
Lee, M.-C., Lin, J.-C., and Gran, E. G. (2021). How far
should we look back to achieve effective real-time
time-series anomaly detection? In Advanced Infor-
mation Networking and Applications: Proceedings of
the 35th International Conference on Advanced In-
formation Networking and Applications (AINA-2021),
Volume 1, pages 136–148. Springer. arXiv preprint
arXiv:2102.06560.
Pang, G. and Aggarwal, C. (2021). Toward explainable
deep anomaly detection. In Proceedings of the 27th
ACM SIGKDD Conference on Knowledge Discovery
& Data Mining, pages 4056–4057.
Ren, H., Xu, B., Wang, Y., Yi, C., Huang, C., Kou, X., Xing,
T., Yang, M., Tong, J., and Zhang, Q. (2019). Time-
series anomaly detection service at microsoft. In Pro-
ceedings of the 25th ACM SIGKDD international con-
ference on knowledge discovery & data mining, pages
3009–3017.
Rettig, L., Khayati, M., Cudr
´
e-Mauroux, P., and
Pi
´
orkowski, M. (2019). Online anomaly detection
over big data streams. Applied Data Science: Lessons
Learned for the Data-Driven Business, pages 289–
312.
Schroeder, F., Mizerkowski, B., and Petersen, W. (2008).
The pocketferrybox–a new portable device for water
quality monitoring in oceans and rivers. Journal of
Operational Oceanography, 1(2):51–57.
SEA.BIRD
Scientific (2023). SBE45 MicroTSG Thermos-
alinograph. https://www.seabird.com/sbe45-micro
tsg-thermosalinograph/product?id=54627900541.
[Online; accessed 23-February-2023].
Su, Y., Zhao, Y., Niu, C., Liu, R., Sun, W., and Pei, D.
(2019). Robust anomaly detection for multivariate
time series through stßochastic recurrent neural net-
work. In Proceedings of the 25th ACM SIGKDD inter-
national conference on knowledge discovery & data
mining, pages 2828–2837.
Wu, W., He, L., Lin, W., Su, Y., Cui, Y., Maple, C., and
Jarvis, S. (2020). Developing an unsupervised real-
time anomaly detection scheme for time series with
multi-seasonality. IEEE Transactions on Knowledge
and Data Engineering, 34(9):4147–4160.
Yao, Y., Sharma, A., Golubchik, L., and Govindan, R.
(2010). Online anomaly detection for sensor systems:
A simple and efficient approach. Performance Evalu-
ation, 67(11):1059–1075.
Zhao, H., Wang, Y., Duan, J., Huang, C., Cao, D., Tong, Y.,
Xu, B., Bai, J., Tong, J., and Zhang, Q. (2020). Multi-
variate time-series anomaly detection via graph atten-
tion network. In 2020 IEEE International Conference
on Data Mining (ICDM), pages 841–850. IEEE.
ICSOFT 2023 - 18th International Conference on Software Technologies
322