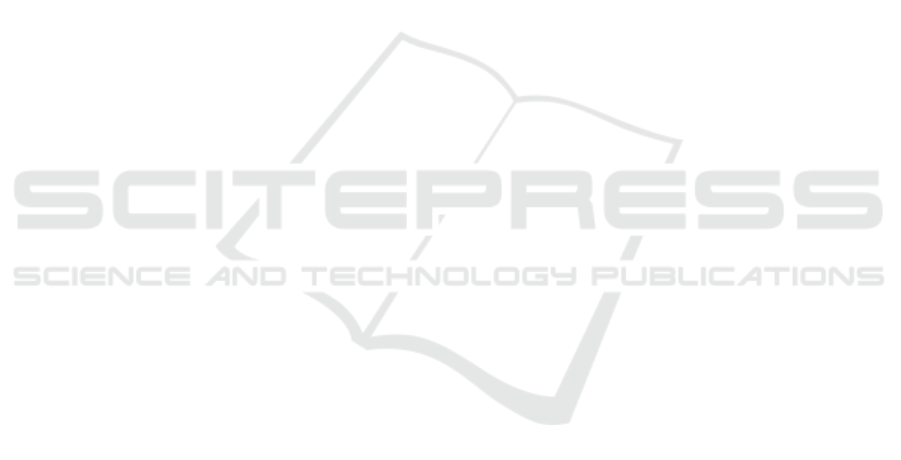
ther research is needed to improve the performance
of IDSs and develop approaches that can effectively
classify network intrusions into different classes.
5.2 Future Work
The study’s limitations include the small dataset size
that may not represent all attacks and an imbalanced
dataset. The classification models were only evalu-
ated on one dataset, and testing them on additional
datasets would be beneficial. Future work aims to
address the imbalance issue, evaluate the models on
more datasets, and compare their performance across
various data types. The study also plans to explore
other classification models, such as recurrent neural
networks and long short-term memory networks. Ad-
ditionally, integrating the results of multiple classifi-
cation models into a single anomaly detection system
could potentially enhance its overall performance.
REFERENCES
Alqahtani, H., Sarker, I., Kalim, A., Minhaz Hossain,
S., Ikhlaq, S., and Hossain, S. (2020). Cyber in-
trusion detection using machine learning classifica-
tion techniques. In Chaubey, N., Parikh, S., and
Amin, K., editors, Computing Science, Communica-
tion and Security, Communications in Computer and
Information Science, pages 121–131, United States.
Springer, Springer Nature. 1st International Confer-
ence on Computing Science, Communication and Se-
curity, COMS2 2020 ; Conference date: 26-03-2020
Through 27-03-2020.
Awan, M., Farooq, U., Babar, H., Yasin, A., Nobanee, H.,
Hussain, M., Hakeem, O., and Zain, A. (2021). Real-
time ddos attack detection system using big data ap-
proach. Sustainability, 13:10743.
Burbank, J. L. (2008). Security in cognitive radio networks:
The required evolution in approaches to wireless net-
work security. In 2008 3rd International Conference
on Cognitive Radio Oriented Wireless Networks and
Communications (CrownCom 2008), pages 1–7.
Cardenas, A., Baras, J., and Seamon, K. (2006). A frame-
work for the evaluation of intrusion detection systems.
In 2006 IEEE Symposium on Security and Privacy
(S&P’06), pages 15 pp.–77.
Communications Security Establishment (CSE), C. I. f.
C. C. (2018). Cse-cicids2018 dataset. https://www.un
b.ca/cic/datasets/ids-2018.html.
Dowd, P. and McHenry, J. (1998). Network security: it’s
time to take it seriously. Computer, 31(9):24–28.
Faker, O. and Dogdu, E. (2019). Intrusion detection using
big data and deep learning techniques. In Proceed-
ings of the 2019 ACM Southeast Conference, ACM
SE ’19, page 86–93, New York, NY, USA. Associa-
tion for Computing Machinery.
Ferrag, M. A., Maglaras, L., Moschoyiannis, S., and Jan-
icke, H. (2020). Deep learning for cyber security in-
trusion detection: Approaches, datasets, and compar-
ative study. Journal of Information Security and Ap-
plications, 50:102419.
for Cybersecurity (CIC), C. I. (2018). Intrusion detection
evaluation dataset (cic-ids2017). https://www.unb.ca
/cic/datasets/ids-2017.html.
He, J., Yang, J., Ren, K., Zhang, W., and Li, G. (2019).
Network security threat detection under big data by
using machine learning. Int. J. Netw. Secur., 21:768–
773.
Information and of California, C. S. U. (1999). Kdd cup 99
dataset. http://kdd.ics.uci.edu/databases/kddcup99.
Kanimozhi, V. and Jacob, T. P. (2019). Artificial intelli-
gence based network intrusion detection with hyper-
parameter optimization tuning on the realistic cyber
dataset cse-cic-ids2018 using cloud computing. In
2019 international conference on communication and
signal processing (ICCSP), pages 0033–0036. IEEE.
Karatas, G., Demir, O., and Sahingoz, O. K. (2020). In-
creasing the performance of machine learning-based
idss on an imbalanced and up-to-date dataset. IEEE
Access, 8:32150–32162.
Lin, P., Ye, K., and Xu, C.-Z. (2019). Dynamic net-
work anomaly detection system by using deep learn-
ing techniques. In International conference on cloud
computing, pages 161–176. Springer.
Marchang, N., Datta, R., and Das, S. K. (2017). A novel
approach for efficient usage of intrusion detection sys-
tem in mobile ad hoc networks. IEEE Transactions on
Vehicular Technology, 66(2):1684–1695.
Praneeth, V., Kumar, K. R., and Karyemsetty, N. (2021).
Security: intrusion prevention system using deep
learning on the internet of vehicles. Interna-
tional Journal of Safety and Security Engineering,
11(3):231–237.
Seth, S., Singh, G., and Kaur Chahal, K. (2021). A novel
time efficient learning-based approach for smart intru-
sion detection system. Journal of Big Data, 8(1):1–28.
Shin, H.-C., Roth, H. R., Gao, M., Lu, L., Xu, Z., Nogues,
I., Yao, J., Mollura, D., and Summers, R. M. (2016).
Deep convolutional neural networks for computer-
aided detection: Cnn architectures, dataset character-
istics and transfer learning. IEEE Transactions on
Medical Imaging, 35(5):1285–1298.
Sydney, U. Unsw-nb15 dataset. https://research.unsw.edu.
au/projects/unsw-nb15-dataset.
DATA 2023 - 12th International Conference on Data Science, Technology and Applications
318