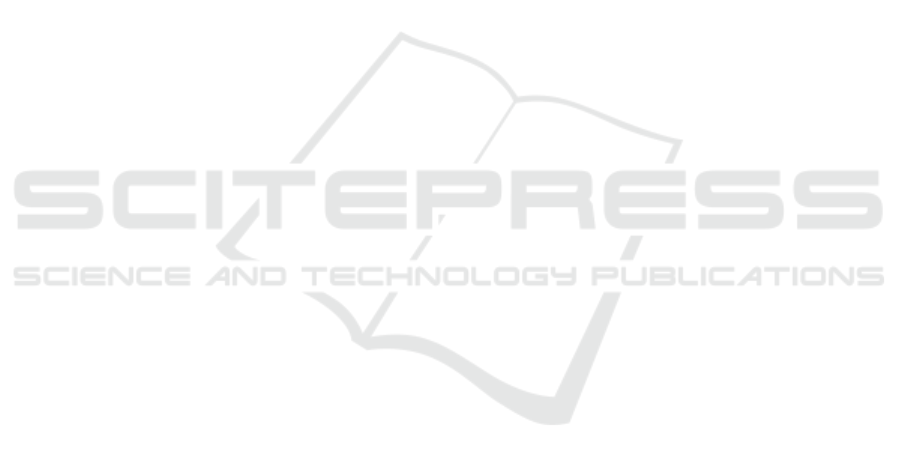
work, which can reduce client-server communication
cost (e.g., hashing-based protocols such as BLH and
OLH) in bandwidth-constrained environments. Third,
recall from Section 5 that bucket sizes are equal for
all buckets (R). We plan to investigate the utility and
privacy impacts of uneven bucket sizes, e.g., whether
uneven bucket sizes can help improve estimation util-
ity, and whether small bucket sizes may increase the
risk of identifiability. Furthermore, we plan to in-
vestigate whether bucket sizes can be automatically
learned from the underlying data.
ACKNOWLEDGEMENTS
We gratefully acknowledge the support from The
Scientific and Technological Research Council of
Turkiye (TUBITAK) under project number 121E303.
REFERENCES
Anderson, B., Lin, S., Newing, A., Bahaj, A., and James, P.
(2017). Electricity consumption and household char-
acteristics: Implications for census-taking in a smart
metered future. Computers, Environment and Urban
Systems, 63:58–67.
Ausgrid (2023). Solar home electricity data.
https://www.ausgrid.com.au/Industry/Our-Research/
Data-to-share/Solar-home-electricity-data.
Beckel, C., Sadamori, L., and Santini, S. (2013). Auto-
matic socio-economic classification of households us-
ing electricity consumption data. Proceedings of the
fourth international conference on Future energy sys-
tems.
Beckel, C., Sadamori, L., Staake, T., and Santini, S. (2014).
Revealing household characteristics from smart meter
data. Energy, 78:397–410.
Benitez, K. and Malin, B. (2010). Evaluating re-
identification risks with respect to the hipaa privacy
rule. Journal of the American Medical Informatics
Association, 17:169–177.
Buchmann, E., B
¨
ohm, K., Burghardt, T., and Kessler, S.
(2012). Re-identification of smart meter data. Per-
sonal and Ubiquitous Computing, 17:653–662.
Cleemput, S., Mustafa, M. A., Marin, E., and Preneel, B.
(2018). De-pseudonymization of smart metering data:
Analysis and countermeasures. 2018 Global Internet
of Things Summit (GIoTS).
Cormode, G., Jha, S., Kulkarni, T., Li, N., Srivastava, D.,
and Wang, T. (2018). Privacy at scale: Local differen-
tial privacy in practice. In Proceedings of the 2018
International Conference on Management of Data,
pages 1655–1658. ACM.
Cz
´
et
´
any, L., V
´
amos, V., Horv
´
ath, M., Szalay, Z., Mota-
Babiloni, A., Deme-B
´
elafi, Z., and Csoknyai, T.
(2021). Development of electricity consumption pro-
files of residential buildings based on smart meter data
clustering. Energy and Buildings, 252:111376.
Datastore, L. (2023). Smartmeter energy
consumption data in london house-
holds. https://data.london.gov.uk/dataset/
smartmeter-energy-use-data-in-london-households.
de Montjoye, Y.-A., Hidalgo, C. A., Verleysen, M., and
Blondel, V. D. (2013). Unique in the crowd: The pri-
vacy bounds of human mobility. Scientific Reports,
3.
Eibl, G., Burkhart, S., and Engel, D. (2019). Insights into
unsupervised holiday detection from low-resolution
smart metering data. In International Conference on
Information Systems Security and Privacy (ICISSP),
pages 281–302. Springer.
Eibl, G. and Engel, D. (2014). Influence of data granularity
on smart meter privacy. IEEE Transactions on Smart
Grid, 6(2):930–939.
Emam, E., Dankar, F. K., Neisa, A., and Jonker, E. (2013).
Evaluating the risk of patient re-identification from
adverse drug event reports. BMC Medical Informat-
ics and Decision Making, 13.
Erlingsson,
´
U., Pihur, V., and Korolova, A. (2014). Rappor:
Randomized aggregatable privacy-preserving ordinal
response. In Proceedings of the 2014 ACM SIGSAC
Conference on Computer and Communications Secu-
rity, pages 1054–1067. ACM.
Fanti, G., Pihur, V., and Erlingsson,
´
U. (2016). Building a
rappor with the unknown: Privacy-preserving learning
of associations and data dictionaries. Proceedings on
Privacy Enhancing Technologies, 2016(3):41–61.
Fung, B. C., Wang, K., Chen, R., and Yu, P. S. (2010).
Privacy-preserving data publishing: A survey of re-
cent developments. ACM Computing Surveys (CSUR),
42(4):1–53.
Gai, N., Xue, K., Zhu, B., Yang, J., Liu, J., and He, D.
(2022). An efficient data aggregation scheme with lo-
cal differential privacy in smart grid. Digital Commu-
nications and Networks, 8(3):333–342.
Gursoy, M. E., Liu, L., Chow, K.-H., Truex, S., and Wei,
W. (2022). An adversarial approach to protocol anal-
ysis and selection in local differential privacy. IEEE
Transactions on Information Forensics and Security,
17:1785–1799.
Gursoy, M. E., Tamersoy, A., Truex, S., Wei, W., and
Liu, L. (2019). Secure and utility-aware data collec-
tion with condensed local differential privacy. IEEE
Transactions on Dependable and Secure Computing,
18(5):2365–2378.
Jia, J. and Gong, N. Z. (2019). Calibrate: Frequency es-
timation and heavy hitter identification with local dif-
ferential privacy via incorporating prior knowledge. In
IEEE International Conference on Computer Commu-
nications (INFOCOM), pages 2008–2016. IEEE.
Khwaja, A. S., Anpalagan, A., Naeem, M., and Venkatesh,
B. (2020). Smart meter data obfuscation using cor-
related noise. IEEE Internet of Things Journal,
7(8):7250–7264.
On the Effectiveness of Re-Identification Attacks and Local Differential Privacy-Based Solutions for Smart Meter Data
121