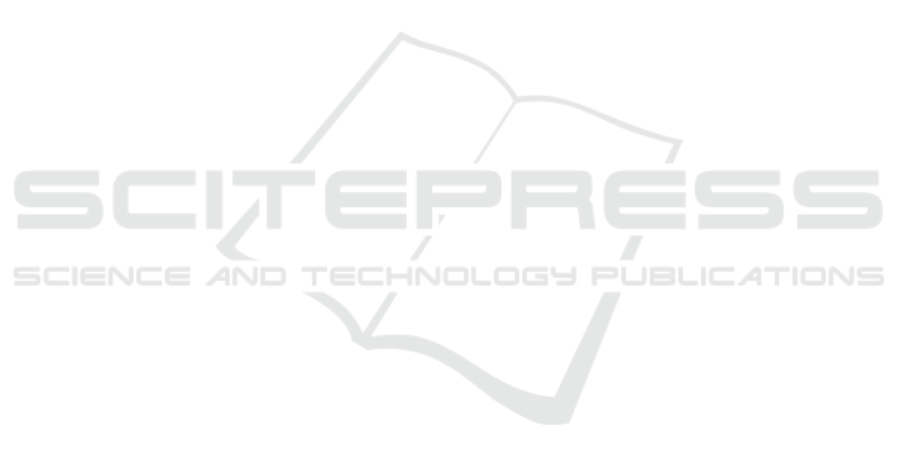
Brunello, A., Montanari, A., and Reynolds, M. (2019).
Synthesis of ltl formulas from natural language texts:
State of the art and research directions. In 26th Inter-
national symposium on temporal representation and
reasoning (TIME 2019). Schloss Dagstuhl-Leibniz-
Zentrum fuer Informatik.
Chan, B., M
¨
oller, T., Pietsch, M., and Soni, T. (2020). Open
sourcing german bert model.
Chen, J.-J. and Marwedel, P. (1990). Petri nets. IEEE
Transactions on knowledge and data engineering,
2(3):311–319.
Devlin, J., Chang, M.-W., Lee, K., and Toutanova,
K. (2019). BERT: Pre-training of Deep Bidirec-
tional Transformers for Language Understanding.
arXiv:1810.04805 [cs].
Frederiks, P. J. M. and van der Weide, T. P. (2006). Infor-
mation modeling: The process and the required com-
petencies of its participants. Data & Knowledge En-
gineering, 58(1):4–20.
Friedrich, F., Mendling, J., and Puhlmann, F. (2011). Pro-
cess model generation from natural language text. In
King, R., editor, Active Flow and Combustion Control
2018, volume 141, pages 482–496. Springer Interna-
tional Publishing. Series Title: Notes on Numerical
Fluid Mechanics and Multidisciplinary Design.
Hamp, B. and Feldweg, H. (1997). Germanet-a lexical-
semantic net for german. In Automatic information
extraction and building of lexical semantic resources
for NLP applications.
Han, X., Dang, Y., Mei, L., Wang, Y., Li, S., and Zhou,
X. (2019). A novel part of speech tagging frame-
work for nlp based business process management. In
2019 IEEE International Conference on Web Services
(ICWS), pages 383–387. IEEE.
Hochreiter, S. and Schmidhuber, J. (1997). Long short-term
memory. Neural computation, 9(8):1735–1780.
Honnibal, M., Montani, I., Van Landeghem, S., Boyd, A.,
et al. (2020). spacy: Industrial-strength natural lan-
guage processing in python.
Kolb, J., Leopold, H., Mendling, J., and Reichert, M.
(2013). Creating and updating personalized and ver-
balized business process descriptions. In The Practice
of Enterprise Modeling: 6th IFIP WG 8.1 Working
Conference, PoEM 2013, Riga, Latvia, November 6-
7, 2013, Proceedings 6, pages 191–205. Springer.
Lacoste, A., Luccioni, A., Schmidt, V., and Dandres, T.
(2019). Quantifying the carbon emissions of machine
learning. arXiv preprint arXiv:1910.09700.
Leopold, H., van Der Aa, H., and Reijers, H. A.
(2018). Identifying candidate tasks for robotic pro-
cess automation in textual process descriptions. In
Enterprise, Business-Process and Information Sys-
tems Modeling: 19th International Conference, BP-
MDS 2018, 23rd International Conference, EMMSAD
2018, Held at CAiSE 2018, Tallinn, Estonia, June 11-
12, 2018, Proceedings 19, pages 67–81. Springer.
Liang, P. P., Wu, C., Morency, L.-P., and Salakhutdinov, R.
(2021). Towards understanding and mitigating social
biases in language models. In International Confer-
ence on Machine Learning, pages 6565–6576. PMLR.
L
´
opez, H. A., Marquard, M., Muttenthaler, L., and
Strømsted, R. (2019). Assisted declarative process
creation from natural language descriptions. In 2019
IEEE 23rd International Enterprise Distributed Ob-
ject Computing Workshop (EDOCW), pages 96–99.
ISSN: 2325-6605.
Mendling, J., Leopold, H., and Pittke, F. (2014). 25 chal-
lenges of semantic process modeling. International
Journal of Information Systems and Software Engi-
neering for Big Companies (IJISEBC), 1(1):78–94.
Mendling, J., van Dongen, B. F., and van der Aalst, W. M.
(2007). On the degree of behavioral similarity be-
tween business process models. In EPK, volume 303,
pages 39–58.
Miller, G. A. (1998). WordNet: An electronic lexical
database. MIT press.
Nivre, J. (2005). Dependency grammar and dependency
parsing. MSI report, 5133(1959):1–32.
Qian, C., Wen, L., Kumar, A., Lin, L., Lin, L., Zong, Z.,
Li, S., and Wang, J. (2020). An approach for process
model extraction by multi-grained text classification.
In International Conference on Advanced Information
Systems Engineering, pages 268–282. Springer.
Schumacher, P., Minor, M., Walter, K., and Bergmann, R.
(2012). Extraction of procedural knowledge from the
web: A comparison of two workflow extraction ap-
proaches. In Proceedings of the 21st International
Conference on World Wide Web, pages 739–747.
Sintoris, K. and Vergidis, K. (2017). Extracting busi-
ness process models using natural language process-
ing (nlp) techniques. In 2017 IEEE 19th conference
on business informatics (CBI), volume 1, pages 135–
139. IEEE.
Van der Aalst, W. M. (1998). The application of petri nets to
workflow management. Journal of circuits, systems,
and computers, 8(01):21–66.
Walter, K., Minor, M., and Bergmann, R. (2011). Workflow
extraction from cooking recipes. In Proceedings of the
ICCBR 2011 Workshops, pages 207–216.
Winter, K. and Rinderle-Ma, S. (2018). Detecting con-
straints and their relations from regulatory documents
using nlp techniques. In On the Move to Meaningful
Internet Systems. OTM 2018 Conferences: Confeder-
ated International Conferences: CoopIS, C&TC, and
ODBASE 2018, Valletta, Malta, October 22-26, 2018,
Proceedings, Part I, pages 261–278. Springer.
Winter, K. and Rinderle-Ma, S. (2019). Deriving and
combining mixed graphs from regulatory documents
based on constraint relations. In International Confer-
ence on Advanced Information Systems Engineering,
pages 430–445. Springer.
Zhang, Z., Webster, P., Uren, V. S., Varga, A., and
Ciravegna, F. (2012). Automatically extracting proce-
dural knowledge from instructional texts using natural
language processing. In LREC, volume 2012, pages
520–527. Citeseer.
DATA 2023 - 12th International Conference on Data Science, Technology and Applications
342