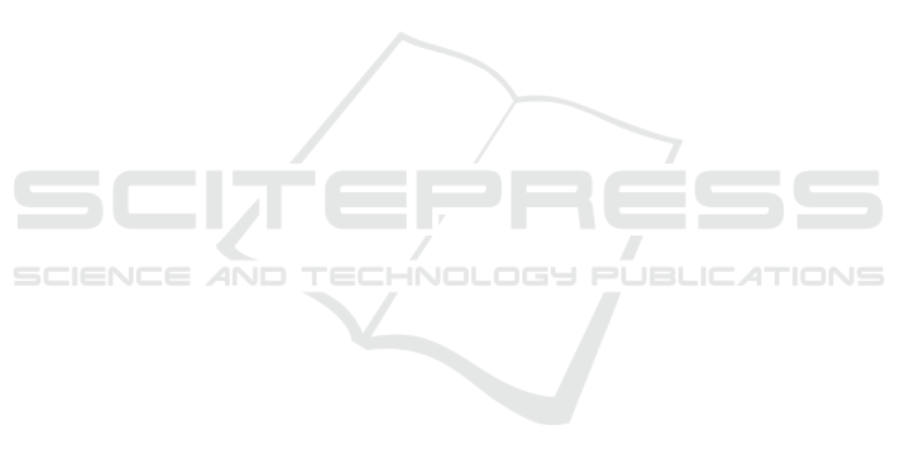
Faker, O. and Dogdu, E. (2019). Intrusion detection using
big data and deep learning techniques. In Proceedings
of the 2019 ACM Southeast conference, pages 86–93.
Gaurav, A., Gupta, B. B., and Panigrahi, P. K. (2022).
A comprehensive survey on machine learning ap-
proaches for malware detection in iot-based enterprise
information system. Enterprise Information Systems,
pages 1–25.
Gogoi, P., Bhuyan, M. H., Bhattacharyya, D., and Kalita,
J. K. (2012). Packet and flow based network intrusion
dataset. In IC3 2012, Noida, India, August 6-8, 2012.,
pages 322–334. Springer.
Guarino, A., Lettieri, N., Malandrino, D., and Zaccagnino,
R. (2021). A machine learning-based approach to
identify unlawful practices in online terms of ser-
vice: analysis, implementation and evaluation. Neural
Computing and Applications, 33:17569–17587.
Guarino, A., Malandrino, D., and Zaccagnino, R. (2022).
An automatic mechanism to provide privacy aware-
ness and control over unwittingly dissemination of
online private information. Computer Networks,
202:108614.
Gupta, B. B. and Sheng, Q. Z. (2019). Machine learning for
computer and cyber security: principle, algorithms,
and practices. CRC Press.
Hooshmand, M. K. and Hosahalli, D. (2022). Network
anomaly detection using deep learning techniques.
CAAI 2022, 7(2):228–243.
Jiang, Z., Sun, L., Philip, S. Y., Li, H., Ma, J., and Shen, Y.
(2020). Target privacy preserving for social networks.
In ICDE 2020, pages 1862–1865. IEEE.
Jing, C., Wang, C., and Yan, C. (2019). Thinking like a
fraudster: Detecting fraudulent transactions via statis-
tical sequential features. In FC 2019, pages 588–604.
Springer.
Koroniotis, N., Moustafa, N., Sitnikova, E., and Turnbull,
B. (2019). Towards the development of realistic botnet
dataset in the internet of things for network forensic
analytics: Bot-iot dataset. Future Generation Com-
puter Systems, 100:779–796.
Kwon, D., Natarajan, K., Suh, S. C., Kim, H., and Kim,
J. (2018). An empirical study on network anomaly
detection using convolutional neural networks. In
ICDCS 2018, pages 1595–1598. IEEE.
Li, Z., Qin, Z., Huang, K., Yang, X., and Ye, S. (2017). In-
trusion detection using convolutional neural networks
for representation learning. In ICONIP 2017, pages
858–866. Springer.
Lo, W. W., Layeghy, S., Sarhan, M., Gallagher, M., and
Portmann, M. (2022). E-graphsage: A graph neural
network based intrusion detection system for iot. In
NOMS 2022, pages 1–9.
Mazzawi, H., Dalal, G., Rozenblatz, D., Ein-Dorx, L., Nin-
iox, M., and Lavi, O. (2017). Anomaly detection in
large databases using behavioral patterning. In ICDE
2017, pages 1140–1149. IEEE.
Moustafa, N. and Slay, J. (2015). Unsw-nb15: a compre-
hensive data set for network intrusion detection sys-
tems. In MilCIS 2015, pages 1–6.
Narasimha Prasad, S., Senthamil Selvan, K., and Lak-
shmi Dhevi, B. (2022). Intrusion detection system in
wireless sensor networks and fair resource allocation
using geometric deep learning techniques. Wireless
Personal Communications, pages 1–12.
Potluri, S., Ahmed, S., and Diedrich, C. (2018). Convolu-
tional neural networks for multi-class intrusion detec-
tion system. In Mining Intelligence and Knowledge
Exploration, pages 225–238. Springer.
Potluri, S. and Diedrich, C. (2017). Deep feature extraction
for multi-class intrusion detection in industrial control
systems. Int. J. Comput. Theory Eng, 9(5):374–379.
Song, J., Takakura, H., and Okabe, Y. (2006). Description
of kyoto university benchmark data.
Sun, P., Li, J., Bhuiyan, M. Z. A., Wang, L., and Li, B.
(2019). Modeling and clustering attacker activities in
iot through machine learning techniques. Information
Sciences, 479:456–471.
Tang, T. A., Mhamdi, L., McLernon, D., Zaidi, S. A. R.,
and Ghogho, M. (2016). Deep learning approach for
network intrusion detection in software defined net-
working. In WINCOM 2016, pages 258–263. IEEE.
Tavallaee, M., Bagheri, E., Lu, W., and Ghorbani, A. A.
(2009). A detailed analysis of the kdd cup 99 data set.
In IEEE CISDA 2009, pages 1–6.
Vinayakumar, R., Soman, K., and Poornachandran, P.
(2017). Applying convolutional neural network for
network intrusion detection. In ICACCI 2017, pages
1222–1228.
Wang, C., Wang, C., Zhu, H., and Cui, J. (2020). Law:
learning automatic windows for online payment fraud
detection. IEEE TDSC, 18(5):2122–2135.
Wang, C. and Zhu, H. (2022). Wrongdoing monitor: A
graph-based behavioral anomaly detection in cyber se-
curity. IEEE TIFS, 17:2703–2718.
Zeng, J., Chua, Z. L., Chen, Y., Ji, K., Liang, Z., and Mao,
J. (2021). Watson: Abstracting behaviors from au-
dit logs via aggregation of contextual semantics. In
NDSS.
Zheng, L., Li, Z., Li, J., Li, Z., and Gao, J. (2019). Ad-
dgraph: Anomaly detection in dynamic graph using
attention-based temporal gcn. In IJCAI, volume 3,
page 7.
Zhu, Y., Xi, D., Song, B., Zhuang, F., Chen, S., Gu,
X., and He, Q. (2020). Modeling users’ behavior
sequences with hierarchical explainable network for
cross-domain fraud detection. In Proceedings of The
Web Conference 2020, pages 928–938.
Towards a Geometric Deep Learning-Based Cyber Security: Network System Intrusion Detection Using Graph Neural Networks
401