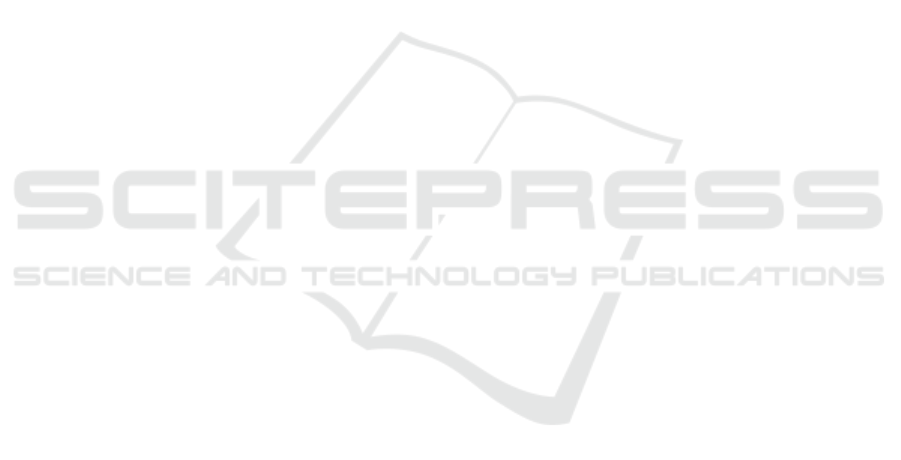
Proceedings of the Workshop on NLP for COVID-19
(Part 2) at EMNLP.
Karni, A. and Thomas, K. (2020). Trump says he’s taking
hydroxychloroquine, prompting warning from health
experts. The New York Times.
Kawintiranon, K. and Singh, L. (2021). Knowledge en-
hanced masked language model for stance detection.
In Proceedings of the Conference of the North Amer-
ican Chapter of the Association for Computational
Linguistics: Human Language Technologies.
Kawintiranon, K. and Singh, L. (2023). DeMis: Data-
efficient misinformation detection using reinforce-
ment learning. In Proceedings of the European
Conference on Machine Learning and Principles
and Practice of Knowledge Discovery in Databases
(ECML-PKDD), pages 224–240. Springer.
Kucher, K., Martins, R. M., Paradis, C., and Kerren, A.
(2020). StanceVis Prime: Visual analysis of sentiment
and stance in social media texts. Journal of Visualiza-
tion, 23(6):1015–1034.
Kumar, S. and Shah, N. (2018). False information on web
and social media: A survey. CRC Press.
Liptak, K. and Klein, B. (2020). Trump says FDA will fast-
track treatments for novel coronavirus, but there are
still months of research ahead. CNN.
Ma, J., Gao, W., Mitra, P., Kwon, S., Jansen, B. J., Wong,
K.-F., and Cha, M. (2016). Detecting rumors from mi-
croblogs with recurrent neural networks. In Proceed-
ings of the International Joint Conference on Artificial
Intelligence.
Maragakis, L. and Kelen, G. D. (2021). COVID-19—myth
versus fact. https://www.hopkinsmedicine.org/health/
conditions-and-diseases/coronavirus/2019-novel-
coronavirus-myth-versus-fact.
McGlynn, J., Baryshevtsev, M., and Dayton, Z. A. (2020).
Misinformation more likely to use non-specific au-
thority references: Twitter analysis of two covid-19
myths. Harvard Kennedy School Misinformation Re-
view, 1(3).
Morgan, I. (2020). Florida orders controver-
sial anti-malaria drug touted by President
Trump as treatment for COVID-19. https:
//floridaphoenix.com/2020/03/28/florida-orders-
controversial-anti-malaria-drug-touted-by-president-
trump-as-treatment-for-covid-19/.
Nielsen, D. S. and McConville, R. (2022). MuMiN: A
large-scale multilingual multimodal fact-checked mis-
information social network dataset. In Proceedings of
the International ACM SIGIR Conference on Research
and Development in Information Retrieval.
Oyeyemi, S. O., Gabarron, E., and Wynn, R. (2014). Ebola,
Twitter, and misinformation: a dangerous combina-
tion? Bmj, 349.
Pedregosa, F., Varoquaux, G., Gramfort, A., Michel, V.,
Thirion, B., Grisel, O., Blondel, M., Prettenhofer, P.,
Weiss, R., Dubourg, V., et al. (2011). Scikit-learn:
Machine learning in Python. Journal of Machine
Learning Research, 12:2825–2830.
Reimers, N. and Gurevych, I. (2019). Sentence-bert: Sen-
tence embeddings using siamese bert-networks. In
Proceedings of the Conference on Empirical Methods
in Natural Language Processing.
Shannon, C. E. (1951). Prediction and entropy of printed
english. Bell System Technical Journal, 30(1):50–64.
Shao, C., Ciampaglia, G. L., Varol, O., Yang, K., Flam-
mini, A., and Menczer, F. (2018). The spread of
low-credibility content by social bots. Nature Comm.,
9(1):1–9.
Shu, K., Sliva, A., Wang, S., Tang, J., and Liu, H. (2017).
Fake news detection on social media: A data mining
perspective. ACM SIGKDD Explorations Newsletter,
19(1):22–36.
Singh, L., Bode, L., Budak, C., Kawintiranon, K., Padden,
C., and Vraga, E. (2020). Understanding high- and
low-quality URL sharing on covid-19 Twitter streams.
Journal of Comp. Social Science, 3(2):343–366.
Vosoughi, S., Roy, D., and Aral, S. (2018). The spread of
true and false news online. Science, 359(6380):1146–
1151.
Wang, Y., Yang, W., Ma, F., Xu, J., Zhong, B., Deng, Q.,
and Gao, J. (2020). Weak supervision for fake news
detection via reinforcement learning. In Proceedings
of the AAAI Conference on Artificial Intelligence.
Weinzierl, M. and Harabagiu, S. (2022). Identifying
the adoption or rejection of misinformation targeting
covid-19 vaccines in Twitter discourse. In Proceed-
ings of the ACM Web Conference.
WHO (2021). Steps towards measuring the burden of info-
demics. In Infodemic Management Conference. World
Health Organization.
WHO (2022). Coronavirus disease (COVID-
19) advice for the public: Mythbusters.
https://www.who.int/emergencies/diseases/novel-
coronavirus-2019/advice-for-public/myth-busters.
Yang, F., Pentyala, S. K., Mohseni, S., Du, M., Yuan, H.,
Linder, R., Ragan, E. D., Ji, S., and Hu, X. (2019).
XFake: Explainable fake news detector with visual-
izations. In Proceedings of the International Confer-
ence on World Wide Web. Association for Comp. Ma-
chinery.
Zhang, T., Kishore, V., Wu, F., Weinberger, K. Q., and
Artzi, Y. (2019). BERTScore: Evaluating text gener-
ation with BERT. In Proceedings of the International
Conference on Learning Representations.
DATA 2023 - 12th International Conference on Data Science, Technology and Applications
76