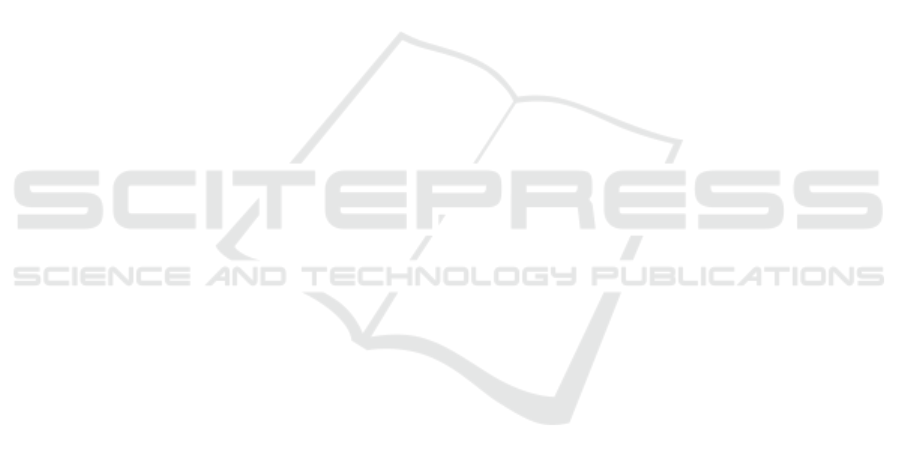
able to effectively process both spatial and temporal
information, resulting in precise predictions of min-
eral percentages. Our proposed approach has a direct
added value on the monitoring of flotation processes.
The model demonstrated a good level of accuracy and
precision, indicating its ability to be generalized on
the whole differential flotation. The use of the Con-
vLSTM network in flotation froth monitoring, show-
cases its potential for similar industrial applications
that require processing of spatial and temporal infor-
mation.
The real time monitoring the flotation froth is a cru-
cial aspect of optimizing and controlling the flotation
process. Our proposed approach offers significant ad-
vantages over existing monitoring techniques, as it is
not only less expensive and low-maintenance but also
provides real-time information on mineral grades.
This makes it a valuable addition to the flotation mon-
itoring process. Once implemented on the zinc cir-
cuit, our proposed soft sensor will be tested for its
ability to accurately monitor the mineral grades of the
CMG differential flotation circuit’s three base miner-
als: lead, copper, and zinc. By combining froth fea-
tures, physio-chemical sensors, and intelligent control
techniques, this innovative approach has the potential
to become a reliable and effective flotation monitor-
ing system. Our proposed approach of using Con-
vLSTM neural networks for real-time monitoring of
mineral grades in flotation froth has significant po-
tential for future industrial applications beyond lead,
copper, and zinc differential flotation sites. Specif-
ically, we will be testing the generalization of our
approach to other mineral compositions using froth
video data, an aspect that will be addressed in future
work. We acknowledge the limited experimental data
used in this study and are committed to conducting
further research to validate the effectiveness of our
proposed solution in a wider range of industrial set-
tings.
ACKNOWLEDGEMENTS
The Smart Connected Mine project, which this re-
search is a part of, was supported by the Moroccan
Ministry of Higher Education, Scientific Research
and Innovation, the Digital Development Agency
(DDA), and the National Center for Scientific and
Technical Research of Morocco (CNRST) through
the Al-Khawarizmi program. The project was a
collaboration between MASCIR (Moroccan Founda-
tion for Advanced Science, Innovation and Research),
REMINEX R&D (an engineering and project man-
agement subsidiary of the MANAGEM Group), UCA
(Cadi Ayyad University), ENSMR (National School
of Mines of Rabat), and ENSIAS (National School
of Computer Science and Systems Analysis at Mo-
hammed V University). We would like to express our
gratitude to the MANAGEM Group and its subsidiary
CMG for providing us with the opportunity to conduct
research, collect and validate data on-site, and for be-
ing an industrial partner in this project.
REFERENCES
Aldrich, C., Avelar, E., and Liu, X. (2022). Recent advances
in flotation froth image analysis. Minerals Engineer-
ing, 188:107823.
Bendaouia, A., Abdelwahed, E. H., Qassimi, S., Boussetta,
A., Benhayoun, A., Benzakour, I., Amar, O., Zen-
nayi, Y., Bourzeix, F., Ba
¨
ına, K., Ba
¨
ına, S., Khalil, A.,
Cherkaoui, M., and Hasidi, O. (2022). Digital Trans-
formation of the Flotation Monitoring Towards an On-
line Analyzer. In Hamlich, M., Bellatreche, L., Sia-
dat, A., and Ventura, S., editors, Smart Applications
and Data Analysis, Communications in Computer and
Information Science, pages 325–338, Cham. Springer
International Publishing.
Cao, W., Wang, R., Fan, M., Fu, X., Wang, H., and
Wang, Y. (2022). A new froth image classification
method based on the MRMR-SSGMM hybrid model
for recognition of reagent dosage condition in the coal
flotation process. Appl. Intell.
Cascone, L., Sadiq, S., Ullah, S., Mirjalili, S., Siddiqui, H.
U. R., and Umer, M. (2023). Predicting Household
Electric Power Consumption Using Multi-step Time
Series with Convolutional LSTM. Big Data Research,
31:100360.
Farrokhpay, S. (2011). The significance of froth stability
in mineral flotation — A review. Advances in Colloid
and Interface Science, 166:1–7.
Huang, L., Hong, X., Yang, Z., Liu, Y., and Zhang, B.
(2022). CNN-LSTM network-based damage detec-
tion approach for copper pipeline using laser ultra-
sonic scanning. Ultrasonics, 121:106685.
Kaartinen, J., H
¨
at
¨
onen, J., Hy
¨
otyniemi, H., and Miettunen,
J. (2006). Machine-vision-based control of zinc flota-
tion—A case study. Control Engineering Practice, 14.
Lindemann, B., Maschler, B., Sahlab, N., and Weyrich, M.
(2021). A survey on anomaly detection for technical
systems using LSTM networks. Computers in Indus-
try, 131:103498.
Liu, J., Gao, Q., Tang, Z., Xie, Y., Gui, W., Ma, T., and
Niyoyita, J. P. (2020). Online Monitoring of Flotation
Froth Bubble-Size Distributions via Multiscale De-
blurring and Multistage Jumping Feature-Fused Full
Convolutional Networks. IEEE Transactions on In-
strumentation and Measurement, 69:9618–9633.
Qassimi, S. and Abdelwahed, E. H. (2022). Disruptive
Innovation in Mining Industry 4.0. In Distributed
Sensing and Intelligent Systems, Studies in Distributed
Conv-LSTM for Real Time Monitoring of the Mineral Grades in the Flotation Froth
95