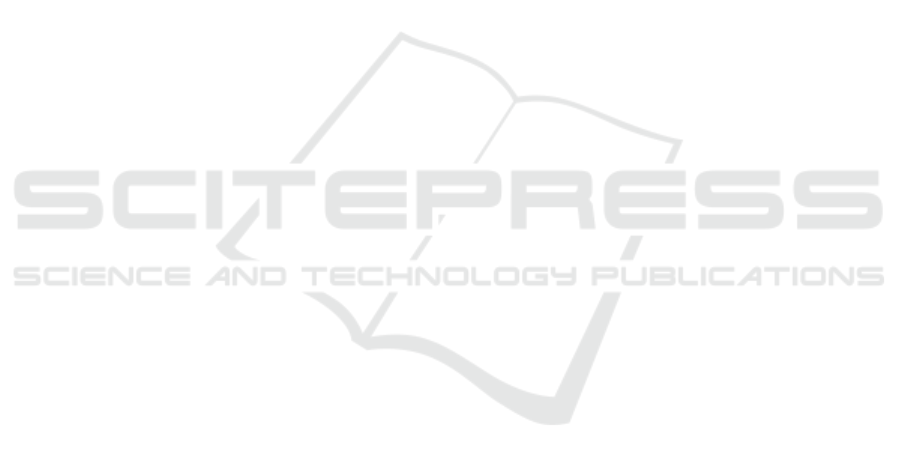
hypervolume. European Journal of Operational Re-
search, 181(3):1653–1669.
Brochu, E., Cora, V. M., and de Freitas, N. (2009). A tuto-
rial on bayesian optimization of expensive cost func-
tions, with application to active user modeling and hi-
erarchical reinforcement learning. Technical Report
UBC TR-2009-023 and arXiv:1012.2599, University
of British Columbia, Department of Computer Sci-
ence. http://arxiv.org/abs/1012.2599/.
Buitinck, L., Louppe, G., Blondel, M., Pedregosa, F.,
Mueller, A., Grisel, O., Niculae, V., Prettenhofer, P.,
Gramfort, A., Grobler, J., Layton, R., VanderPlas, J.,
Joly, A., Holt, B., and Varoquaux, G. (2013). API de-
sign for machine learning software: experiences from
the scikit-learn project. In ECML PKDD Workshop:
Languages for Data Mining and Machine Learning,
pages 108–122.
Cox, D. D. and John, S. (1992). A statistical method for
global optimization. In [Proceedings] 1992 IEEE In-
ternational Conference on Systems, Man, and Cyber-
netics, pages 1241–1246. IEEE.
Deb, K., Pratap, A., Agarwal, S., and Meyarivan, T. (2002).
A fast and elitist multiobjective genetic algorithm:
Nsga-ii. IEEE Transactions on Evolutionary Compu-
tation, 6(2):182–197.
Ehrgott, M. (2005). Multicriteria optimization, volume 491.
Springer Science & Business Media.
Frazier, P. I. (2018). A tutorial on bayesian optimization.
https://arxiv.org/abs/1807.02811/.
Galuzio, P. P., de Vasconcelos Segundo, E. H., dos San-
tos Coelho, L., and Mariani, V. C. (2020). Mobopt
— multi-objective bayesian optimization. SoftwareX,
12:100520.
Ghazali, M. R., Ahmad, M. A., Suid, M. H., and Tumari, M.
Z. M. (2022). A dc/dc buck-boost converter-inverter-
dc motor control based on model-free pid controller
tuning by adaptive safe experimentation dynamics al-
gorithm. In 2022 57th International Universities
Power Engineering Conference (UPEC), pages 1–6.
Jones, D. R. (2001). A taxonomy of global optimiza-
tion methods based on response surfaces. Journal of
Global Optimization, 21(4):345–383.
Jones, D. R., Schonlau, M., and Welch, W. J. (1998).
Efficient global optimization of expensive black-
box functions. Journal of Global Optimization,
13(4):455–492.
Kaminski, M. (2020). Nature-inspired algorithm imple-
mented for stable radial basis function neural con-
troller of electric drive with induction motor. Ener-
gies, 13(24).
Khosravi, M., Behrunani, V. N., Myszkorowski, P.,
Smith, R. S., Rupenyan, A., and Lygeros, J. (2021).
Performance-driven cascade controller tuning with
bayesian optimization. IEEE Transactions on Indus-
trial Electronics, 69(1):1032–1042.
Kushner, H. J. (1964). A New Method of Locating the
Maximum Point of an Arbitrary Multipeak Curve in
the Presence of Noise. Journal of Basic Engineering,
86(1):97–106.
K
¨
onig, C., Turchetta, M., Lygeros, J., Rupenyan, A., and
Krause, A. (2021). Safe and efficient model-free adap-
tive control via bayesian optimization. In 2021 IEEE
International Conference on Robotics and Automation
(ICRA), pages 9782–9788.
Magtrol Inc. (2022a). Dsp7000 – high speed programmable
controller. https://www.magtrol.com/product/
dsp7000-high-speed-programmable-controller/,
Accessed: 2022-07-15.
Magtrol Inc. (2022b). Hd series – hysteresis dy-
namometers. https://www.magtrol.com/product/
hysteresis-dynamometers-hd-series/, Accessed:
2022-07-15.
Mockus, J. (1975). On bayesian methods for seeking the
extremum. In Marchuk, G. I., editor, Optimization
Techniques IFIP Technical Conference Novosibirsk,
July 1–7, 1974, pages 400–404, Berlin, Heidelberg.
Springer Berlin Heidelberg.
Mockus, J. (2012). Bayesian approach to global optimiza-
tion: theory and applications, volume 37. Springer
Science & Business Media.
Naidu Kommula, B. and Reddy Kota, V. (2022). Design of
mfa-pso based fractional order pid controller for effec-
tive torque controlled bldc motor. Sustainable Energy
Technologies and Assessments, 49:101644.
Neumann-Brosig, M., Marco, A., Schwarzmann, D., and
Trimpe, S. (2020). Data-efficient autotuning with
bayesian optimization: An industrial control study.
IEEE Transactions on Control Systems Technology,
28(3):730–740.
Ngatchou, P., Zarei, A., and El-Sharkawi, A. (2005). Pareto
multi objective optimization. In Proceedings of the
13th International Conference on, Intelligent Systems
Application to Power Systems, pages 84–91.
Rasmussen, C. E. (2003). Gaussian processes in machine
learning. In Summer school on machine learning,
pages 63–71. Springer.
Shahriari, B., Swersky, K., Wang, Z., Adams, R. P., and
de Freitas, N. (2016). Taking the human out of the
loop: A review of bayesian optimization. Proceedings
of the IEEE, 104(1):148–175.
Sharma, A., Sharma, V., and Rahi, O. P. (2022). Pso tuned
pid controller for dc motor speed control. In Suhag,
S., Mahanta, C., and Mishra, S., editors, Control and
Measurement Applications for Smart Grid, pages 79–
89, Singapore. Springer Nature Singapore.
Snoek, J., Larochelle, H., and Adams, R. P. (2012). Practi-
cal bayesian optimization of machine learning algo-
rithms. In Pereira, F., Burges, C., Bottou, L., and
Weinberger, K., editors, Advances in Neural Infor-
mation Processing Systems, volume 25. Curran Asso-
ciates, Inc.
Tarczewski, T. and Grzesiak, L. M. (2018). An applica-
tion of novel nature-inspired optimization algorithms
to auto-tuning state feedback speed controller for
pmsm. IEEE Transactions on Industry Applications,
54(3):2913–2925.
Zitzler, E., Knowles, J., and Thiele, L. (2008). Quality
Assessment of Pareto Set Approximations. Springer
Berlin Heidelberg, Berlin, Heidelberg.
ICINCO 2023 - 20th International Conference on Informatics in Control, Automation and Robotics
114