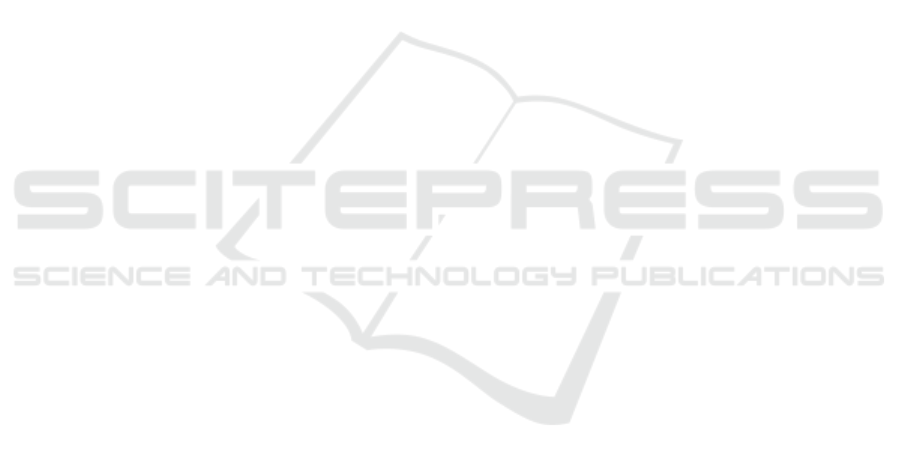
TD data, and designed a heterogeneous graph embed-
ding model along with metapath design. The pro-
posed frameworks were validated through a compari-
son with possible frameworks using combinations of
graph configurations and embedding models. In addi-
tion, through ablation experiments, we demonstrated
the usefulness of TD for disease prediction, and ef-
fects of the metapath design were investigated. Al-
though the proposed framework shows outstanding
performance compared to existing embedding mod-
els, further study for an enhanced embedding model
specific to our EHR-TD data can be conducted in the
future. We expect that the proposed framework will
contribute to more accurate disease prediction and
disease management in patients.
ACKNOWLEDGEMENTS
This work was supported by the National Research
Foundation of Korea(NRF) grant funded by the Korea
government(MSIT) (No. 2021R1F1A1059255).
REFERENCES
Cao, J., Lin, X., Guo, S., Liu, L., Liu, T., and Wang, B.
(2021). Bipartite graph embedding via mutual in-
formation maximization. In Proceedings of the 14th
ACM International Conference on Web Search and
Data Mining, WSDM ’21, page 635–643, New York,
NY, USA. Association for Computing Machinery.
Davis, A. P., Grondin, C. J., Johnson, R. J., Sci-
aky, D., Wiegers, J., Wiegers, T. C., and Mat-
tingly, C. J. (2020). Comparative Toxicogenomics
Database (CTD): update 2021. Nucleic Acids Re-
search, 49(D1):D1138–D1143.
DeSalvo, K. B., Bloser, N., Reynolds, K., He, J., and Munt-
ner, P. (2006). Mortality prediction with a single gen-
eral self-rated health question. Journal of General In-
ternal Medicine, 21(3):267–275.
Dong, Y., Chawla, N. V., and Swami, A. (2017). Meta-
path2vec: Scalable representation learning for het-
erogeneous networks. In Proceedings of the 23rd
ACM SIGKDD International Conference on Knowl-
edge Discovery and Data Mining, KDD ’17, page
135–144, New York, NY, USA. Association for Com-
puting Machinery.
Fu, X., Zhang, J., Meng, Z., and King, I. (2020). Magnn:
Metapath aggregated graph neural network for hetero-
geneous graph embedding. In Proceedings of The Web
Conference 2020, WWW ’20, page 2331–2341, New
York, NY, USA. Association for Computing Machin-
ery.
Gao, M., Chen, L., He, X., and Zhou, A. (2018). Bine: Bi-
partite network embedding. In The 41st International
ACM SIGIR Conference on Research & Development
in Information Retrieval, SIGIR ’18, page 715–724,
New York, NY, USA. Association for Computing Ma-
chinery.
Gentimis, T., Alnaser, A. J., Durante, A., Cook, K.,
and Steele, R. (2017). Predicting hospital length
of stay using neural networks on mimic iii data.
In 2017 IEEE 15th Intl Conf on Dependable,
Autonomic and Secure Computing, 15th Intl
Conf on Pervasive Intelligence and Comput-
ing, 3rd Intl Conf on Big Data Intelligence and
Computing and Cyber Science and Technology
Congress(DASC/PiCom/DataCom/CyberSciTech),
pages 1194–1201.
Grover, A. and Leskovec, J. (2016). node2vec: Scal-
able feature learning for networks. In Proceedings
of the 22nd ACM SIGKDD international conference
on Knowledge discovery and data mining, pages 855–
864.
Jin, B., Che, C., Liu, Z., Zhang, S., Yin, X., and Wei, X.
(2018). Predicting the risk of heart failure with ehr
sequential data modeling. IEEE Access, 6:9256–9261.
Johnson, A. E., Pollard, T. J., Shen, L., Lehman, L.-w. H.,
Feng, M., Ghassemi, M., Moody, B., Szolovits, P.,
Anthony Celi, L., and Mark, R. G. (2016). Mimic-
iii, a freely accessible critical care database. Scientific
Data, 3(1):160035.
Leskovec, J. and Mcauley, J. (2012). Learning to dis-
cover social circles in ego networks. In Pereira, F.,
Burges, C., Bottou, L., and Weinberger, K., editors,
Advances in Neural Information Processing Systems,
volume 25. Curran Associates, Inc.
Ma, F., You, Q., Xiao, H., Chitta, R., Zhou, J., and Gao, J.
(2018). Kame: Knowledge-based attention model for
diagnosis prediction in healthcare. In Proceedings of
the 27th ACM International Conference on Informa-
tion and Knowledge Management, CIKM ’18, page
743–752, New York, NY, USA. Association for Com-
puting Machinery.
Nasiri, E., Berahmand, K., Rostami, M., and Dabiri, M.
(2021). A novel link prediction algorithm for protein-
protein interaction networks by attributed graph em-
bedding. Computers in Biology and Medicine,
137:104772.
Perozzi, B., Al-Rfou, R., and Skiena, S. (2014). Deep-
walk: Online learning of social representations. In
Proceedings of the 20th ACM SIGKDD International
Conference on Knowledge Discovery and Data Min-
ing, KDD ’14, page 701–710, New York, NY, USA.
Association for Computing Machinery.
DATA 2023 - 12th International Conference on Data Science, Technology and Applications
104