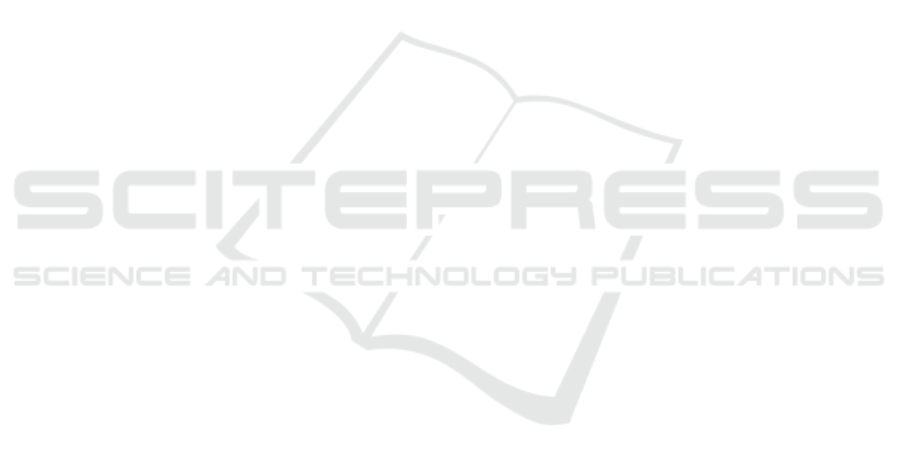
rine organisms. While AUVs have previously op-
erated semi-autonomously under human oversight,
significant strides towards full autonomy have been
achieved, as documented in (Issac et al., 1979).
Currently, AUVs have found extensive use in
mapping and surveying tasks since their inception in
the 1970s. Notably, the HUGIN series, developed by
Kongsberg Maritime and the Norwegian Defense Re-
search Establishment, stands out as a highly success-
ful commercial AUV platform. Nevertheless, AUVs
face challenges related to navigation, communication,
autonomy, and endurance, with a strong focus on en-
hancing autonomy in ongoing research. Various con-
trol techniques are applied for distinct operations, en-
compassing pitch and depth control, tracking and for-
mation control, and obstacle avoidance strategies that
make use of forward-looking sonar for detecting and
evading static obstacles (Desa et al., 2001), (Coleman,
2003), (Issac et al., 1979).
In both land and sea environments, the hurdle of
obstacle avoidance presents significant difficulties for
robots. Ground robots can execute maneuvers like
stop-back-turn, but underwater vehicles face unique
complications due to the necessity of halting for-
ward momentum and maintaining a stationary posi-
tion (Healey and Kim, 2000). This challenge has
implications for the autonomy and navigation of Au-
tonomous Underwater Vehicles (AUVs) ((Desa et al.,
2001). Particularly in uncharted territories, AUVs
grapple with the complexities of adhering to pro-
grammed waypoints, thus driving the exploration of
artificial intelligence techniques to dynamically navi-
gate around obstacles in the dynamic marine environ-
ment (Coleman, 2003). Moreover, the current designs
of AUVs reveal limitations in their ability to adapt to
various littoral conditions (Blidberg, 2003).
The Indian Underwater Robotics Society (IURS),
founded by Indian students abroad, introduced au-
tonomous underwater vehicle technology to India
with the creation of BhAUV in 2005 (Li and Xiao,
2005). Subsequently, IURS advanced their efforts by
developing a cost-effective AUV named Jal, which is
equipped with navigational sensors, sonar, and com-
puter vision capabilities. Similarly, the National In-
stitute of Oceanography (NIO) in Goa made strides
in 2009 by launching Maya, a compact autonomous
underwater vehicle designed for oceanic data collec-
tion (Prestero, 2009). Moreover, the Central Mechan-
ical Engineering Research Institute (CMERI) played
a pivotal role in India’s autonomous underwater ve-
hicle development, producing a versatile platform ca-
pable of tasks such as mapping, surveillance, mine
countermeasures, and oceanographic measurements,
even in challenging weather conditions (Li and Xiao,
2005) and (Prestero, 2009).
Navigating underwater vehicles while avoiding
obstacles presents multifaceted challenges related to
autonomy, nonlinear modeling, and environmental
uncertainties. Researchers have explored diverse
control strategies, including Sliding Mode Control
(SMC), Fuzzy Logic Control (FLC), and Backstep-
ping, to tackle these complexities. Advancements like
pseudo-spectral methods, Rapidly-exploring Random
Trees (RRT), and Probabilistic RoadMap (PRM) have
elevated trajectory planning and control precision, en-
hancing overall performance. Nevertheless, existing
trajectory planning methods often overlook dynamic
constraints, curtailing optimization and tracking ca-
pabilities. To surmount this, a refined mathematical
model is recommended to attain more accurate and
trackable navigation trajectories. These innovations
have the potential to substantially elevate AUV per-
formance, especially in dynamic underwater condi-
tions.
In this study, the central focus centers on devis-
ing a control methodology that leverages SMC along-
side imaging sonar sensors for precise pitch and depth
control, specifically geared towards obstacle avoid-
ance. The integration of these advanced techniques
aims to enhance AUV maneuverability and naviga-
tion accuracy, contributing to safer and more effective
underwater operations.
The article is structured into distinct sections. Sec-
tion II covers AUV modeling, encompassing kine-
matic and dynamic equations. In Section III, the uti-
lization of SMC for obstacle avoidance is elucidated,
employing sonar technology to identify obstacles in
the AUV’s trajectory. It details the AUV’s depth ad-
justment to navigate a new path based on obstruction
height and range data. Section IV showcases exper-
imental outcomes validating the effectiveness of the
proposed approach. Finally, Section V offers con-
cluding remarks, suggesting potential avenues for fu-
ture work.
2 AUV SYSTEM MODEL
2.1 Kinematic and Dynamic Modeling
The AUV used in this work is treated as a rigid body,
assuming minimal deflections during movement. The
acceleration of the earth has negligible impact on the
car’s center of mass, as noted by (Healey and Kim,
2000). The primary forces acting on the AUV in-
clude inertial, gravitational, hydrostatic, propulsion,
thruster, and hydrodynamic forces from lift and drag,
as explained by (Healey and Kim, 2000). Euler rota-
ICINCO 2023 - 20th International Conference on Informatics in Control, Automation and Robotics
530