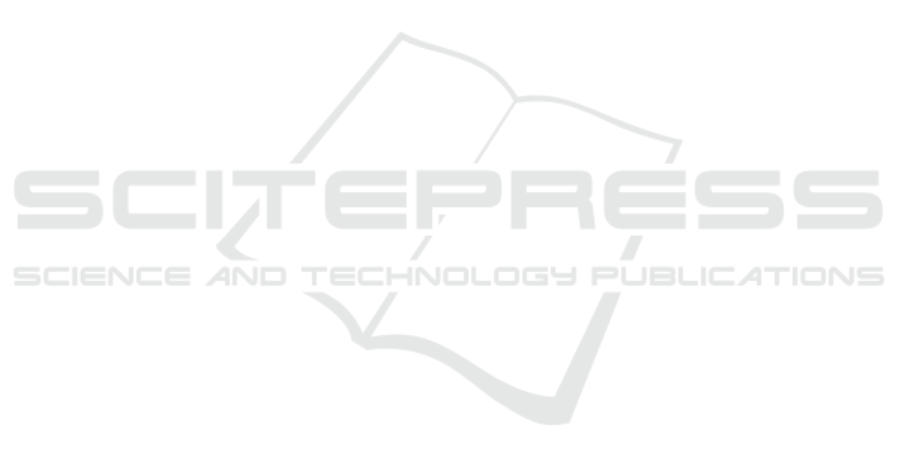
Kuechler, B. and Vaishnavi, V. (2011). Promoting relevance
in is research: An informing system for design science
research. InformingSciJ, 14:125–138.
L
¨
ohr, B., Brennig, K., Bartelheimer, C., Beverungen, D.,
and M
¨
uller, O. (2022). Process mining of knowledge-
intensive processes: An action design research study
in manufacturing. pages 251–267.
Maheswaran, S., Indhumathi, N., Dhanalakshmi, S., Nan-
dita, S., Shafiq, I. M., and Rithka, P. (2022). Iden-
tification and classification of groundnut leaf disease
using convolutional neural network. pages 251–270.
Mar-Raave, J. R. D., Bahs¸i, H., Mr
ˇ
si
´
c, L., and Hausknecht,
K. (2021). A machine learning-based forensic tool
for image classification - a design science approach.
Forensic Science International: Digital Investigation,
38.
Martin, D., K
¨
uhl, N., von Bischhoffshausen, J. K., and
Satzger, G. (2020). System-wide learning in cyber-
physical service systems: A research agenda. volume
12388 LNCS, pages 457–468. Springer Science and
Business Media Deutschland GmbH.
Mart
´
ınez-Plumed, F., Contreras-Ochando, L., Ferri, C.,
Hern
´
andez-Orallo, J., Kull, M., Lachiche, N.,
Ram
´
ırez-Quintana, M. J., and Flach, P. (2021). Crisp-
dm twenty years later: From data mining processes
to data science trajectories. IEEE Transactions on
Knowledge and Data Engineering, 33(8):3048–3061.
Mayring, P. (2000). Pensionierung als krise oder
gl
¨
ucksgewinn?–ergebnisse aus einer quantitativ-
qualitativen l
¨
angsschnittuntersuchung. Zeitschrift f
¨
ur
Gerontologie und Geriatrie, 33:124–133.
Miah, S. J., Solomonides, I., and Gammack, J. G. (2020). A
design-based research approach for developing data-
focussed business curricula. Education and Informa-
tion Technologies, 25:553–581.
Molnar, E., Molnar, R., and Gregus, M. (2020). Business
schools and ris3 – enterprise architecture perspective.
Mombini, H., Tulu, B., Strong, D., Agu, E., Nguyen, H.,
Lindsay, C., Loretz, L., Pedersen, P., and Dunn, R.
(2020). Design of a machine learning system for pre-
diction of chronic wound management decisions. vol-
ume 12388 LNCS, pages 15–27. Springer Science and
Business Media Deutschland GmbH.
Monteiro, J., Barata, J., Veloso, M., Veloso, L., and Nunes,
J. (2022). A scalable digital twin for vertical farming.
JAIHC.
Odu, N. B., Prasad, R., Onime, C., and Sharma, B. K.
(2022). How to implement a decision support for dig-
ital health: Insights from design science perspective
for action research in tuberculosis detection. IJIM, 2.
Pan, Y. and Zhang, L. (2021). A bim-data mining integrated
digital twin framework for advanced project manage-
ment. Automation in Construction, 124.
Panzner, M., von Enzberg, S., Meyer, M., and Dumitrescu,
R. (2022). Characterization of usage data with the
help of data classifications. Journal of the Knowledge
Economy.
Pascal, A. and Renaud, A. (2020). 15 years of informa-
tion systems design science research: A bibliographic
analysis. Proceedings of HICSS 2020, pages 5016–
5025.
Peffers, K., Rothenberger, M., Tuunanen, T., and Vaezi, R.
(2012). Design science research evaluation. volume
7286, pages 398–410.
Peffers, K., Tuunanen, T., Gengler, C. E., Rossi, M., Hui,
W., Virtanen, V., and Bragge, J. (2006). The design
science research process: A model for producing and
presenting information systems research. pages 83–
106.
Peffers, K., Tuunanen, T., Rothenberger, M. A., and Chat-
terjee, S. (2007). A design science research method-
ology for information systems research. JMIS, 24:45–
77.
Peixoto, D., Faria, M., Macedo, R., Peixoto, H., Lopes,
J., Barbosa, A., Guimar
˜
aes, T., and Santos, M. F.
(2022). Determining internal medicine length of stay
by means of predictive analytics. volume 13566
LNAI, pages 171–182.
Pohl, M., Staegemann, D. G., and Turowski, K. (2022).
The performance benefit of data analytics applica-
tions. volume 201, pages 679–683. Elsevier B.V.
Romanov, D., Molokanov, V., Kazantsev, N., and Jha,
A. K. (2022). Removing order effects from human-
classified datasets: A machine learning method to im-
prove decision making systems. Decision Support
Systems.
Saar-Tsechansky, M. (2015). The business of business data
science in is journals. MIS Quarterly: Management
Information Systems, 39:iii–vi.
Samuel-Ojo, O., Shimabukuro, D., Chatterjee, S., Muthui,
M., Babineau, T., Prasertsilp, P., Ewais, S., and
Young, M. (2010). Meta-analysis of design science
research within the is community: Trends, patterns,
and outcomes. Global Perspectives on Design Science
Research, pages 124–138.
Schuster, T., Waidelich, L., and Volz, R. (2021). Matu-
rity models for the assessment of artificial intelligence
in small and medium-sized enterprises. volume 429
LNBIP, pages 22–36. Springer Science and Business
Media Deutschland GmbH.
Schwade, F. (2021). Social collaboration analytics frame-
work: A framework for providing business intelli-
gence on collaboration in the digital workplace. Deci-
sion Support Systems, 148.
Schwaiger, J., Hammerl, T., Florian, J., and Leist, S. (2021).
Ur: Smart–a tool for analyzing social media content.
ISeB, 19:1275–1320.
Schweiger, L., Barth, L., and Meierhofer, J. (2020). Data
resources to create digital twins. Proceedings of SDS
2020, pages 55–56.
Simonofski, A., Fink, J., and Burnay, C. (2021). Supporting
policy-making with social media and e-participation
platforms data: A policy analytics framework. GIQ,
38.
Soares, C. M. (2022). Crowdsourced data to improve mu-
nicipalities governance : Sesimbra’s case. pages 22–
25.
Sonnenberg, C. and Brocke, J. V. (2012). Lncs 7286 - eval-
DATA 2023 - 12th International Conference on Data Science, Technology and Applications
134