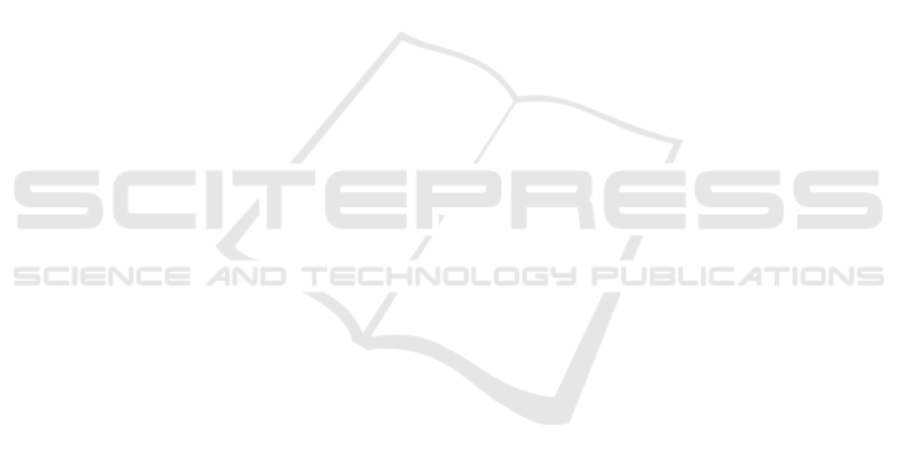
REFERENCES
Abdar, M., Pourpanah, F., Hussain, S., Rezazadegan, D.,
Liu, L., Ghavamzadeh, M., Fieguth, P. W., Cao, X.,
Khosravi, A., Acharya, U. R., Makarenkov, V., and
Nahavandi, S. (2021). A review of uncertainty quan-
tification in deep learning: Techniques, applications
and challenges. Inf. Fusion, 76.
Boros, T., Dumitrescu, S. D., and Pipa, S. (2017). Fast and
accurate decision trees for natural language process-
ing tasks. In RANLP. INCOMA Ltd.
Goodfellow, I. J., Pouget-Abadie, J., Mirza, M., Xu, B.,
Warde-Farley, D., Ozair, S., Courville, A. C., and Ben-
gio, Y. (2014). Generative adversarial nets. In NIPS.
Guillermo, L., Rojas, J.-M., and Ugarte, W. (2022). Emo-
tional 3d speech visualization from 2d audio visual
data. International Journal of Modeling, Simulation,
and Scientific Computing, 0(0):2450002.
Hou, X., Zhang, X., Liang, H., Shen, L., Lai, Z., and Wan,
J. (2022). Guidedstyle: Attribute knowledge guided
style manipulation for semantic face editing. Neural
Networks, 145.
Huang, X. and Belongie, S. J. (2017). Arbitrary style trans-
fer in real-time with adaptive instance normalization.
In ICCV. IEEE.
Karras, T., Laine, S., and Aila, T. (2021). A style-based
generator architecture for generative adversarial net-
works. IEEE Trans. Pattern Anal. Mach. Intell.,
43(12).
Leon-Urbano, C. and Ugarte, W. (2020). End-to-end elec-
troencephalogram (EEG) motor imagery classification
with long short-term. In SSCI, pages 2814–2820.
IEEE.
Matsumori, S., Abe, Y., Shingyouchi, K., Sugiura, K., and
Imai, M. (2021). Lattegan: Visually guided language
attention for multi-turn text-conditioned image manip-
ulation. IEEE Access, 9.
Patashnik, O., Wu, Z., Shechtman, E., Cohen-Or, D., and
Lischinski, D. (2021). Styleclip: Text-driven manipu-
lation of stylegan imagery. In ICCV. IEEE.
Radford, A., Kim, J. W., Hallacy, C., Ramesh, A., Goh, G.,
Agarwal, S., Sastry, G., Askell, A., Mishkin, P., Clark,
J., Krueger, G., and Sutskever, I. (2021). Learning
transferable visual models from natural language su-
pervision. In ICML, volume 139. PMLR.
Shen, Y., Gu, J., Tang, X., and Zhou, B. (2020). Interpreting
the latent space of gans for semantic face editing. In
CVPR. IEEE.
Tewari, A., Elgharib, M., R., M. B., Bernard, F., Seidel, H.,
P
´
erez, P., Zollh
¨
ofer, M., and Theobalt, C. (2020). PIE:
portrait image embedding for semantic control. ACM
Trans. Graph., 39(6).
Vint, D., Anderson, M., Yang, Y., Ilioudis, C. V., Cate-
rina, G. D., and Clemente, C. (2021). Automatic
target recognition for low resolution foliage penetrat-
ing SAR images using cnns and gans. Remote. Sens.,
13(4).
V
´
azquez, B. C. (2021). El papel de los influencers en la
creaci
´
on y reproducci
´
on del estereotipo de belleza fe-
menina en instagram. Master’s thesis, Universidad de
Salamanca.
Xu, X., Chen, Y., Tao, X., and Jia, J. (2022). Text-
guided human image manipulation via image-text
shared space. IEEE Trans. Pattern Anal. Mach. Intell.,
44(10).
Ysique-Neciosup, J., Chavez, N. M., and Ugarte, W. (2022).
Deephistory: A convolutional neural network for au-
tomatic animation of museum paintings. Comput. An-
imat. Virtual Worlds, 33(5).
Zhu, D., Mogadala, A., and Klakow, D. (2019). Im-
age manipulation with natural language using two-
sidedattentive conditional generative adversarial net-
work. CoRR, abs/1912.07478.
ICSBT 2023 - 20th International Conference on Smart Business Technologies
82