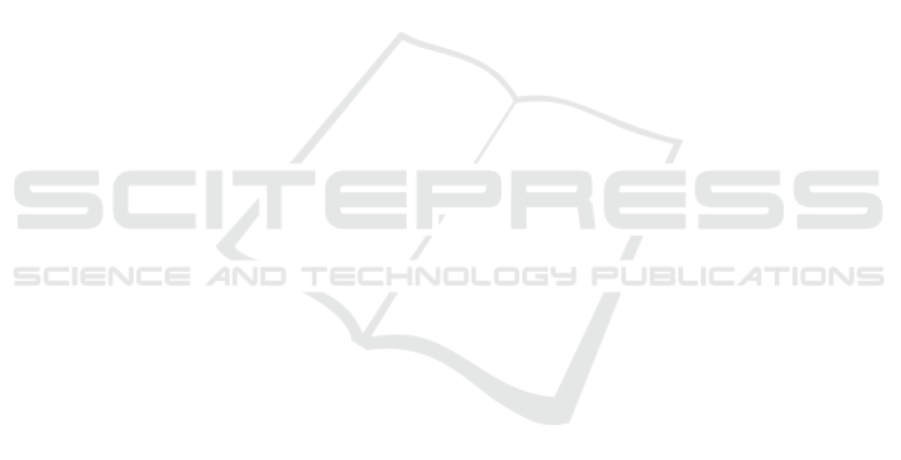
significant delays but, if they do coordinate, they can
both delay the process significantly and also have a
significant influence on the decision outcomes (con-
trolling which decisions are finally made).
A small number of SAs, as little as 10% can, under
the right conditions, double the time to reach consen-
sus (and thus also doubling the cost, as a measure of
effort).
A group of well coordinated SAs that make up
more than 20% of the group can cause delays ranging
from about doubling the time for project completion
to completely stall projects.
As future work we propose Social Psychology re-
search experiments to covertly observe the behaviour
of a planted agent within a mock project and the ef-
fect this could have. For one, can such an agent stay
hidden, what would be good strategies for subverting
team members, and, when to give up being subver-
sive, since our research suggest most of the damage
had been done about halfway through the project, and
thus that seems to be a good point in time to stop be-
ing subversive, or at least reduce risk of detection by
being more cooperative.
From a computational intelligence perspective,
extending a multi-agent system with AI-based sub-
versive behaviour could yield more complex strate-
gies; and, ways to address such behaviour, both form
a detection as well as a mitigation perspective.
REFERENCES
Al-Ahmad, W., Al-Fagih, K., Khanfar, K., Alsamara, K.,
Abuleil, S., and Abu-Salem, H. (2009). A taxonomy
of an it project failure: root causes. International
Management Review, 5(1):93.
Amirkhani, A. and Barshooi, A. H. (2022). Consensus in
multi-agent systems: a review. Artificial Intelligence
Review, 55(5):3897–3935.
Bao, G., Ma, L., and Yi, X. (2022). Recent advances on
cooperative control of heterogeneous multi-agent sys-
tems subject to constraints: A survey. Systems Science
& Control Engineering, 10(1):539–551.
Barnes, M. and Prior, D. (2009). Subversive citizens: Power,
agency and resistance in public services. Policy Press.
Browning, G. (2010). Agency and influence in the his-
tory of political thought: The agency of influence and
the influence of agency. History of Political Thought,
31(2):345–366.
Chang, M.-h. and Harrington, J. E. (2004). Agent-based
models of organizations working paper, no. 515.
Den Boon, A. K. and Van Meurs, A. (1991). Measuring
opinion distributions: An instrument for the measure-
ment of perceived opinion distributions. Quality and
Quantity, 25(4):359–379.
Dunbar, R. I. (1998). The social brain hypothesis. Evo-
lutionary Anthropology: Issues, News, and Reviews:
Issues, News, and Reviews, 6(5):178–190.
Evans, M. S. and Romerstein, H. (2012). Stalin’s secret
agents: the subversion of Roosevelt’s government. Si-
mon and Schuster.
Gilbert, N. and Gilbert, G. N. (2010). Computational social
science. null.
Kian, M. E., Sun, M., and Bosch
´
e, F. (2016). A consistency-
checking consensus-building method to assess com-
plexity of energy megaprojects. Procedia-social and
behavioral sciences, 226:43–50.
Lang, J. W., Bliese, P. D., and de Voogt, A. (2018). Mod-
eling consensus emergence in groups using longi-
tudinal multilevel methods. Personnel Psychology,
71(2):255–281.
Leishman, T. G., Green, D. G., and Driver, S. (2008). Self-
organization in simulated social networks. null.
Manky, O. and Dolores, J. (2022). Subversive en-
trepreneurs: Business agency and commodification of
peruvian higher education (1992–2012). Latin Amer-
ican Perspectives, 49(3):162–180.
McDowell, K. (2002). Roll of thunder, hear my cry: A
culturally specific, subversive concept of child agency.
Children’s Literature in Education, 33:213–225.
Stocker, R., Green, D. G., and Newth, D. (2001). Consensus
and cohesion in simulated social networks. Journal of
Artificial Societies and Social Simulation, 4(4).
Tesfatsion, L. and Judd, K. L. (2006). Handbook of compu-
tational economics: agent-based computational eco-
nomics. Elsevier.
Vorster, J. S. and Leenen, L. (2023). Consensus simulator
for organisational structures. Proceedings of the 13th
International Conference on Simulation and Modeling
Methodologies, Technologies and Applications.
Waheeb, R. A. and Andersen, B. S. (2022). Causes of
problems in post-disaster emergency re-construction
projects—iraq as a case study. Public Works Manage-
ment & Policy, 27(1):61–97.
Whitney, K. M. and Daniels, C. B. (2013). The root cause
of failure in complex it projects: Complexity itself.
Procedia Computer Science, 20:325–330.
Will, M. G., Al-Kfairy, M., and Mellor, R. B. (2019). How
organizational structure transforms risky innovations
into performance–a computer simulation. Simulation
Modelling Practice and Theory, 94:264–285.
Yang, R., Liu, L., and Feng, G. (2022). An overview of
recent advances in distributed coordination of multi-
agent systems. Unmanned Systems, 10(03):307–325.
SIMULTECH 2023 - 13th International Conference on Simulation and Modeling Methodologies, Technologies and Applications
114