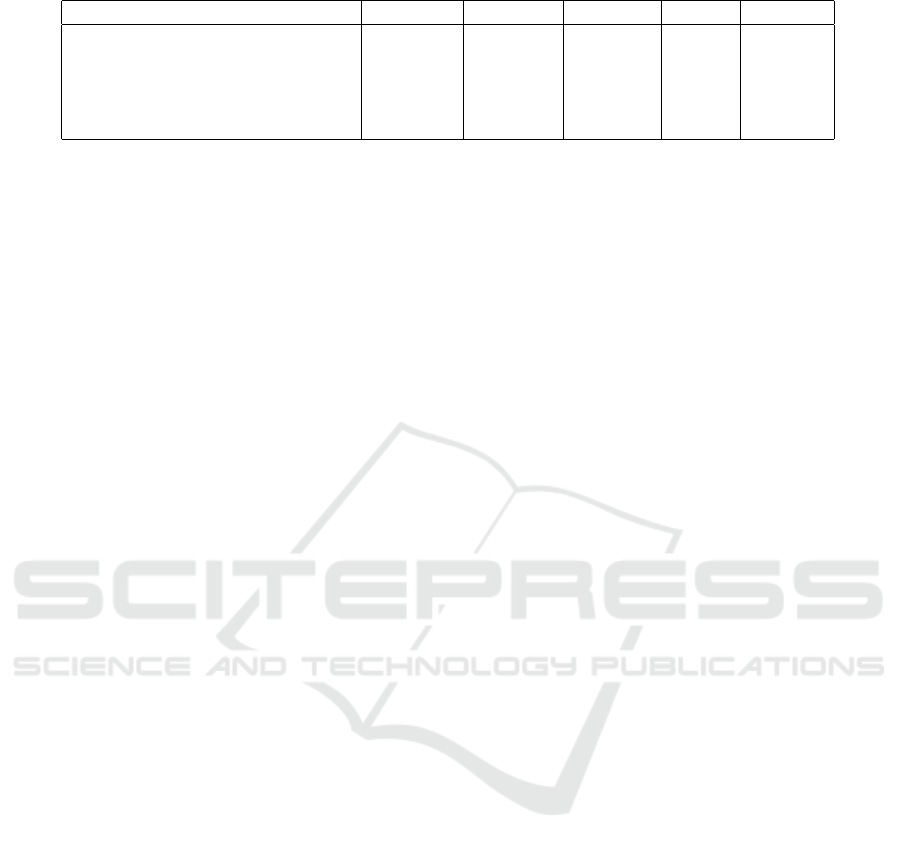
Table 3: Comparison of performance metrics’ for intrusion detection with NSL-KDD dataset.
Reference Approach Accuracy Precision Recall F1-score
(Caminero et al., 2019) AE-RL 0.8016 0.7974 0.8000 0.7940
(Suwannalai and Polprasert, 2020) AE-RL 0.8000 X X 0.7900
(Sethi et al., 2021) A-DQN 0.9720 0.9650 0.9910 0.9780
Our COCA-MADQN MADQN 0.9850 1.000 0.9850 0.9920
Our MADQN-GTN MADQN 0.9760 0.9750 0.9730 0.9740
REFERENCES
Ahsan, M. M., Raman, S., and Siddique, Z. (2023). Bsgan:
A novel oversampling technique for imbalanced pat-
tern recognitions. arXiv preprint arXiv:2305.09777.
Caminero, G., Lopez-Martin, M., and Carro, B. (2019).
Adversarial environment reinforcement learning algo-
rithm for intrusion detection. Computer Networks,
159:96–109.
Canese, L., Cardarilli, G. C., Di Nunzio, L., Fazzolari, R.,
Giardino, D., Re, M., and Span
`
o, S. (2021). Multi-
agent reinforcement learning: A review of challenges
and applications. Applied Sciences, 11(11):4948.
Ibrahim, A. M., Yau, K.-L. A., Chong, Y.-W., and Wu, C.
(2021). Applications of multi-agent deep reinforce-
ment learning: Models and algorithms. Applied Sci-
ences, 11(22):10870.
Lee, H., Hong, J., and Jeong, J. (2022). Marl-based dual re-
ward model on segmented actions for multiple mobile
robots in automated warehouse environment. Applied
Sciences, 12(9):4703.
Lopez-Martin, M., Sanchez-Esguevillas, A., Arribas, J. I.,
and Carro, B. (2022). Supervised contrastive learn-
ing over prototype-label embeddings for network in-
trusion detection. Information Fusion, 79:200–228.
Mnih, V., Kavukcuoglu, K., Silver, D., Rusu, A. A., Ve-
ness, J., Bellemare, M. G., Graves, A., Riedmiller, M.,
Fidjeland, A. K., Ostrovski, G., et al. (2015). Human-
level control through deep reinforcement learning. na-
ture, 518(7540):529–533.
Molina-Coronado, B., Mori, U., Mendiburu, A., and
Miguel-Alonso, J. (2020). Survey of network intru-
sion detection methods from the perspective of the
knowledge discovery in databases process. IEEE
Transactions on Network and Service Management,
17(4):2451–2479.
Nguyen, N. D., Nguyen, T. T., Pham, N. T., Nguyen, H.,
Nguyen, D. T., Nguyen, T. D., Lim, C. P., Johnstone,
M., Bhatti, A., Creighton, D., et al. (2022). To-
wards designing a generic and comprehensive deep
reinforcement learning framework. Applied Intelli-
gence, pages 1–22.
Nguyen, T. T., Nguyen, N. D., and Nahavandi, S. (2020).
Deep reinforcement learning for multiagent systems:
A review of challenges, solutions, and applications.
IEEE transactions on cybernetics, 50(9):3826–3839.
Nguyen, T. T. and Reddi, V. J. (2021). Deep reinforce-
ment learning for cyber security. IEEE Transactions
on Neural Networks and Learning Systems.
Qi, J., Zhou, Q., Lei, L., and Zheng, K. (2021). Federated
reinforcement learning: Techniques, applications, and
open challenges. arXiv preprint arXiv:2108.11887.
Servin, A. and Kudenko, D. (2008). Multi-agent rein-
forcement learning for intrusion detection. In Adap-
tive Agents and Multi-Agent Systems III. Adaptation
and Multi-Agent Learning: 5th, 6th, and 7th Euro-
pean Symposium, ALAMAS 2005-2007 on Adaptive
and Learning Agents and Multi-Agent Systems, Re-
vised Selected Papers, pages 211–223. Springer.
Sethi, K., Madhav, Y. V., Kumar, R., and Bera, P. (2021).
Attention based multi-agent intrusion detection sys-
tems using reinforcement learning. Journal of Infor-
mation Security and Applications, 61:102923.
Sutton, R. S., Barto, A. G., et al. (1998). Introduction to
reinforcement learning, volume 135. MIT press Cam-
bridge.
Suwannalai, E. and Polprasert, C. (2020). Network in-
trusion detection systems using adversarial reinforce-
ment learning with deep q-network. In 2020 18th In-
ternational Conference on ICT and Knowledge Engi-
neering (ICT&KE), pages 1–7. IEEE.
Vlontzos, A., Alansary, A., Kamnitsas, K., Rueckert, D.,
and Kainz, B. (2019). Multiple landmark detec-
tion using multi-agent reinforcement learning. In
Medical Image Computing and Computer Assisted
Intervention–MICCAI 2019: 22nd International Con-
ference, Shenzhen, China, October 13–17, 2019, Pro-
ceedings, Part IV 22, pages 262–270. Springer.
Zhang, K., Yang, Z., and Bas¸ar, T. (2021). Multi-agent rein-
forcement learning: A selective overview of theories
and algorithms. Handbook of reinforcement learning
and control, pages 321–384.
Zhu, C., Dastani, M., and Wang, S. (2022). A survey of
multi-agent reinforcement learning with communica-
tion. arXiv preprint arXiv:2203.08975.
Zhu, M., Hu, Z., and Liu, P. (2014). Reinforcement learning
algorithms for adaptive cyber defense against heart-
bleed. In Proceedings of the first ACM workshop on
moving target defense, pages 51–58.
Improving Intrusion Detection Systems with Multi-Agent Deep Reinforcement Learning: Enhanced Centralized and Decentralized
Approaches
777