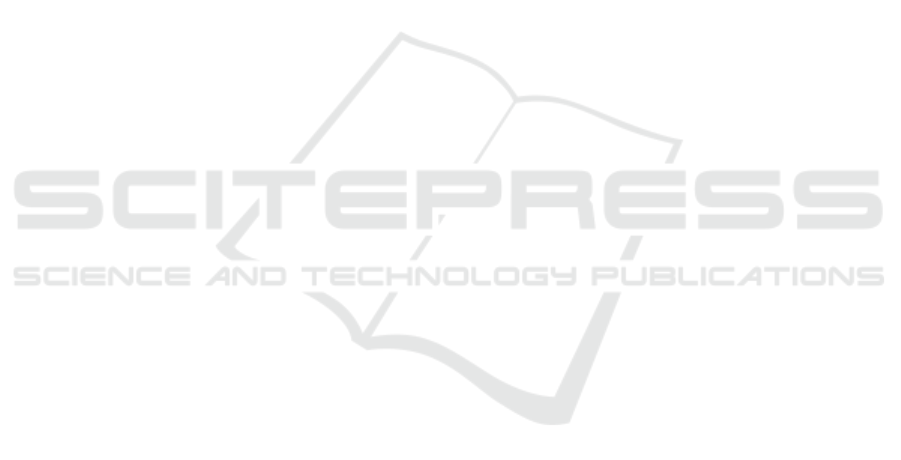
huge gap related to the fake news detection issue and
cross-disciplinary concepts related to it that can be in-
vestigated in the future.
ACKNOWLEDGEMENTS
The publication was created in the framework of Pol-
ish National Agency for Academic Exchange under
the ”STER Programme – Internationalisation of Doc-
toral Schools” as part of the project ”Curriculum for
advanced doctoral education & training – CADET
Academy of Lodz University of Technology”.
REFERENCES
Abdulrahman, A. and Baykara, M. (2020). Fake news de-
tection using machine learning and deep learning al-
gorithms. In 2020 International Conference on Ad-
vanced Science and Engineering (ICOASE), Duhok,
Iraq, pages 18–23.
Agrawal, C., Pandey, A., and Goyal, S. (2021). A survey on
role of machine learning and nlp in fake news detec-
tion on social media. In 4th International Conference
on Computing, Power and Communication Technolo-
gies (GUCON), pages 1–7.
Alharbi, R., Vu, M., and Thai, M. (2021). Evaluating
fake news detection models from explainable machine
learning perspectives. In Proceedings of IEEE IN-
TERNATIONAL CONFERENCE ON COMMUNICA-
TIONS (ICC 2021).
Babu, D., Sushmitha, G., Lasya, D., Krishna, D., and Ra-
jesh, V. (2022). Identifying fake news using machine
learning. In 2022 International Conference on Elec-
tronics and Renewable Systems (ICEARS), pages 1–6.
Baskaran, S. (2022). Publications trends in big data: A sci-
entometric analysis.
Biwalkar, A., Rao, A., and Shah, K. (2021). Real or fake:
An intrinsic analysis using supervised machine learn-
ing algorithms. In 5th International Conference on
I-SMAC (IoT in Social, Mobile, Analytics and Cloud)
(I-SMAC), pages 372–380.
Choras, M. (2020). Advanced machine learning techniques
for fake news (online disinformation) detection.
CSO, C. S. O. (2020). Information Integrity. Online,
https://www.cso.ie/en/releasesandpublications/ep/p-
isshisi/householdinternetsecurityandinformationintegrity
2021/informationintegrity/.
Ding, Y., Wang, Y., and Wang, Y. (2021). It’s time to con-
front fake news and rumors on social media: A bib-
liometric study based on vosviewer. In 4th Intern.
Conference on Computer and Communication Engi-
neering Technology, pages 226–232.
Gerbina, T. (2021). Science disinformation: On the problem
of fake news.
Guo, D., Chen, H., Long, R., Lu, H., and Long, Q. (2017).
A co-word analysis of organizational constraints for
maintaining sustainability.
Heilig, L. and Vob, S. (2014). A scientometric analysis of
cloud computing literature.
Jain, A. and Kasbe, A. (2018). Fake news detection.
Khalil, A., Jarrah, M., Aldwairi, M., and Jararweh, Y.
(2021). Detecting arabic fake news using machine
learning. In 2nd International Conference on Intelli-
gent Data Science Technologies and Applications (ID-
STA), pages 171–177.
Khokhlov, A. (2020). How scientometrics became the most
important science for researchers of all specialties.
Mazov, N., Gureev, V., and Glinskikh, V. (2020). The
methodological basis of defining research trends and
fronts.
Paor, S. D. and Heravi, B. (2020). Information literacy and
fake news: How the field of librarianship can help
combat the epidemic of fake news.
Raynaud, M. (2021). Impact of the covid-19 pandemic on
publication dynamics and non-covid-19 research pro-
duction.
Reuters (2021). Fact check: ’133 million registered
voters’ argument uses flawed logic. Online,
https://www.reuters.com/article/uk-factcheck-voters-
133-million-idUSKBN296284.
Rohman, M., Khairani, D., Hulliyah, K., Arini, P.,
Riswandi, and Lakoni, I. (2021). Systematic liter-
ature review on methods used in classification and
fake news detection in indonesian. In 9th Interna-
tional Conference on Cyber and IT Service Manage-
ment (CITSM), pages 1–4.
Schotten, M., el Aisati, M., Meester, W., Steiginga, S.,
and Ross, C. (2017). A brief history of scopus: The
world’s largest abstract and citation database of scien-
tific literature.
(SMU), S. M. U. (2020). Using VOSviewer as a
bibliometric mapping or analysis tool in Busi-
ness, Management & accounting. Online,
https://library.smu.edu.sg/topics-insights/using-
vosviewer-bibliometric-mapping-or-analysis-tool-
business-management.
Srainternational (2020). Enabling Collaborative Work
in Higher Education: An Exploration of Enhanc-
ing Research Collaborations Within an Institution.
Online, https://www.srainternational.org/blogs/srai-
jra1/2019/12/08/enabling-collaborative-work-in-
higher-education.
Tavares, B., da Silva, E. S., and de Souza, A. D. (2017).
Risk management analysis in scrum software projects.
van Eck, N. and Waltman, L. (2010). Software survey:
Vosviewer, a computer program for bibliometric map-
ping.
Vinhas, O. and Bastos, M. (2022). Fact-checking misinfor-
mation: Eight notes on consensus reality.
Wang, C.-C. (2020). Fake news and related concepts: Defi-
nitions and recent research developments.
Scientometric Analysis of Fake News Detection and Machine Learning Based on VOSviewer
555