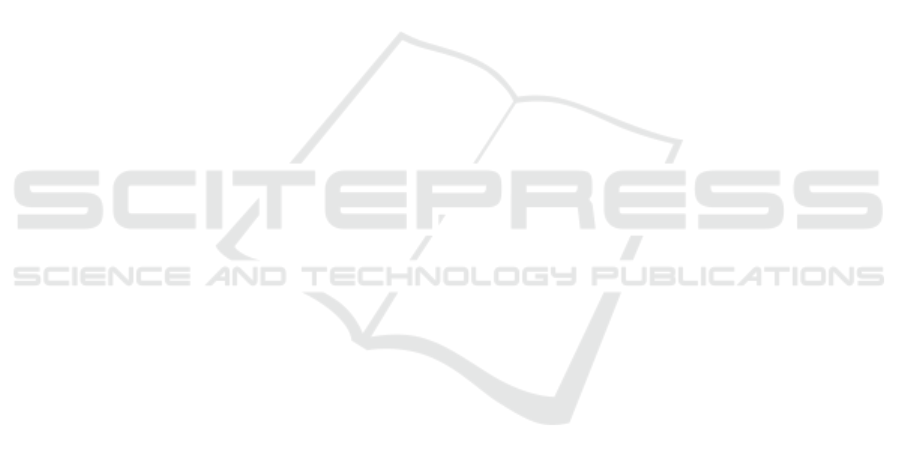
REFERENCES
Adamu, H., Lutfi, S. L., Malim, N. H. A. H., Hassan, R.,
Di Vaio, A., and Mohamed, A. S. A. (2021). Fram-
ing twitter public sentiment on nigerian government
covid-19 palliatives distribution using machine learn-
ing. Sustainability, 13(6):3497.
Agarwal, A., Agarwal, B., Harjule, P., and Agarwal, A.
(2021). Mental health analysis of students in major
cities of india during covid-19. Predictive and preven-
tive measures for Covid-19 pandemic, pages 51–67.
Alamoodi, A. H., Zaidan, B. B., Zaidan, A. A., Albahri,
O. S., Mohammed, K. I., Malik, R. Q., Almahdi,
E. M., Chyad, M. A., Tareq, Z., Albahri, A. S.,
et al. (2021). Sentiment analysis and its applica-
tions in fighting covid-19 and infectious diseases: A
systematic review. Expert systems with applications,
167:114155.
Bao, X., Wang, Z., Jiang, X., Xiao, R., and Li, S. (2022).
Aspect-based sentiment analysis with opinion tree
generation. IJCAI 2022, pages 4044–4050.
Das, S. and Dutta, A. (2020). Characterizing public emo-
tions and sentiments in COVID-19 environment: A
case study of India. J. Hum. Behav. Soc. Environ.,
31(1-4):1–14.
Fan, C., Chen, M., Wang, X., Wang, J., and Huang, B.
(2021). A review on data preprocessing techniques to-
ward efficient and reliable knowledge discovery from
building operational data. Frontiers in Energy Re-
search, 9:652801.
Fazzinga, B., Flesca, S., Furfaro, F., and Masciari, E.
(2013). Rfid-data compression for supporting aggre-
gate queries. ACM Transactions on Database Systems
(TODS), 38(2):1–45.
Flint, S. W., Piotrkowicz, A., and Watts, K. (2021). Use of
Artificial Intelligence to understand adults’ thoughts
and behaviours relating to COVID-19. Perspect. Pub-
lic Health, XX(X):1–8.
G
¨
uner, H. R., Hasano
˘
glu,
˙
I., and Aktas¸, F. (2020). Covid-
19: Prevention and control measures in community.
Turkish Journal of medical sciences, 50(9):571–577.
H. Manguri, K., N. Ramadhan, R., and R. Mohammed
Amin, P. (2020). Twitter Sentiment Analysis on
Worldwide COVID-19 Outbreaks. Kurdistan J. Appl.
Res., pages 54–65.
Hiremath, B. N. and Patil, M. M. (2022). Enhancing op-
timized personalized therapy in clinical decision sup-
port system using natural language processing. Jour-
nal of King Saud University-Computer and Informa-
tion Sciences, 34(6):2840–2848.
Hussain, A., Tahir, A., Hussain, Z., Sheikh, Z., Gogate, M.,
Dashtipour, K., Ali, A., and Sheikh, A. (2021). Arti-
ficial intelligence-enabled analysis of public attitudes
on facebook and twitter toward COVID-19 vaccines
in the United Kingdom and the United States: Obser-
vational study. J. Med. Internet Res., 23(4):1–10.
Jelodar, H., Wang, Y., Orji, R., and Huang, H. (2020).
Deep sentiment classification and topic discovery on
novel coronavirus or COVID-19 online discussions:
NLP using LSTM recurrent neural network approach.
arXiv, 24(10):2733–2742.
Kwan, J. S.-L. and Lim, K. H. (2021). Tweetcovid: a sys-
tem for analyzing public sentiments and discussions
about covid-19 via twitter activities. In 26th Inter-
national Conference on Intelligent User Interfaces-
Companion, pages 58–60.
Luo, W., Majumder, M. S., Liu, D., Poirier, C., Mandl,
K. D., Lipsitch, M., and Santillana, M. (2020). The
role of absolute humidity on transmission rates of the
covid-19 outbreak. MedRxiv, pages 2020–02.
Lwin, M. O., Lu, J., Sheldenkar, A., Schulz, P. J., Shin,
W., Gupta, R., and Yang, Y. (2020). Global senti-
ments surrounding the COVID-19 pandemic on Twit-
ter: Analysis of Twitter trends. JMIR Public Heal.
Surveill., 6(2):1–4.
Manguri, K. H., Ramadhan, R. N., and Amin, P. R. M.
(2020). Twitter sentiment analysis on worldwide
covid-19 outbreaks. Kurdistan Journal of Applied Re-
search, pages 54–65.
Masciari, E. (2007). Rfid data management for effective ob-
jects tracking. In Proceedings of the 2007 ACM sym-
posium on Applied computing, pages 457–461.
Nemes, L. and Kiss, A. (2021). Social media sentiment
analysis based on covid-19. Journal of Information
and Telecommunication, 5(1):1–15.
Praveen, S. V., Ittamalla, R., and Deepak, G. (2021). Ana-
lyzing Indian general public’s perspective on anxiety,
stress and trauma during Covid-19 - A machine learn-
ing study of 840,000 tweets. Diabetes Metab. Syndr.
Clin. Res. Rev., 15(3):667–671.
Raheja, S. and Asthana, A. (2021). Sentimental analysis of
twitter comments on COVID-19. Proc. Conflu. 2021
11th Int. Conf. Cloud Comput. Data Sci. Eng., pages
704–708.
Singh, M., Jakhar, A. K., and Pandey, S. (2021). Senti-
ment analysis on the impact of coronavirus in social
life using the BERT model. Soc. Netw. Anal. Min.,
11(1):1–11.
Ullah, M. H., Gelli, G., and Verde, F. (2023). Visible
light backscattering with applications to the internet
of things: State-of-the-art, challenges, and opportuni-
ties. Internet of Things, page 100768.
Umair, A. and Masciari, E. (2023). Sentimental and spa-
tial analysis of covid-19 vaccines tweets. Journal of
Intelligent Information Systems, 60(1):1–21.
Umair, A., Masciari, E., and Habib Ullah, M. H. (2021).
Sentimental analysis applications and approaches dur-
ing covid-19: a survey. In Proceedings of the 25th
International Database Engineering & Applications
Symposium, pages 304–308.
Yu, J. and Jiang, J. (2019). Adapting bert for target-oriented
multimodal sentiment classification. IJCAI.
Yue, L., Chen, W., Li, X., Zuo, W., and Yin, M. (2019). A
survey of sentiment analysis in social media. Knowl-
edge and Information Systems, 60:617–663.
An Advanced BERT LayerSum Model for Sentiment Classification of COVID-19 Tweets
151