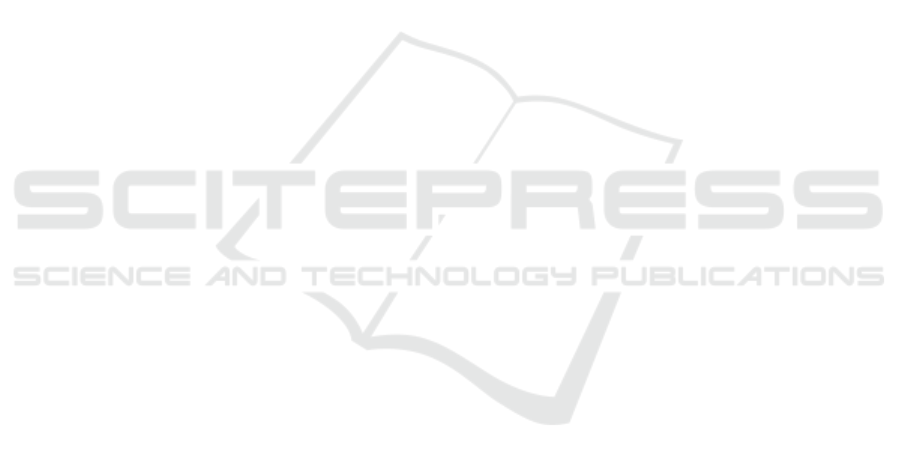
6 CONCLUSION
We have proposed an IMAGINE dataset for bench-
marking digital camera identification algorithms. Our
dataset contains number of images coming from mod-
ern CMOS-based devices. This dataset may be used
for testing digital camera identification algorithms
using different methodologies, including statistical
methods, machine learning or deep models with con-
volutional neural networks (CNN). We have evalu-
ated our dataset on a set of modern state-of-the-art
algorithms for individual source camera identifica-
tion. Results confirmed the reliability of IMAGINE
dataset.
ACKNOWLEDGEMENTS
The project financed under the program of the Pol-
ish Minister of Science and Higher Education under
the name “Regional Initiative of Excellence” in the
years 2019–2022 project number 020/RID/2018/19,
the amount of financing 12,000,000.00 PLN.
The authors would like to thank the Editorial Of-
fice of Optyczne.pl (Optyczne, 2023) website for
sharing part of images to the proposed dataset.
REFERENCES
Bondi, L., Baroffio, L., Guera, D., Bestagini, P., Delp,
E. J., and Tubaro, S. (2017). First steps toward cam-
era model identification with convolutional neural net-
works. IEEE Signal Process. Lett., 24(3):259–263.
De Marsico, M., Nappi, M., Riccio, D., and Wechsler, H.
(2015). Mobile iris challenge evaluation (miche)-i,
biometric iris dataset and protocols. Pattern Recog-
nition Letters, 57:17–23.
Ding, X., Chen, Y., Tang, Z., and Huang, Y. (2019). Cam-
era identification based on domain knowledge-driven
deep multi-task learning. IEEE Access, 7:25878–
25890.
Fi
´
errez-Aguilar, J., Ortega-Garcia, J., Toledano, D. T.,
and Gonzalez-Rodriguez, J. (2007). Biosec baseline
corpus: A multimodal biometric database. Pattern
Recognition, 40(4):1389–1392.
Flickr (2023). Flickr, https://www.flickr.com/. Online; ac-
cessed 5 April 2023.
Gloe, T. and B
¨
ohme, R. (2010). The ‘Dresden Image
Database’ for benchmarking digital image forensics.
In Proceedings of the 25th Symposium On Applied
Computing (ACM SAC 2010), volume 2, pages 1585–
1591.
Goljan, M. (2008). Digital camera identification from im-
ages - estimating false acceptance probability. In Digi-
tal Watermarking, 7th International Workshop, IWDW
2008, pages 454–468.
Hadwiger, B. and Riess, C. (2020). The forchheim im-
age database for camera identification in the wild. In
Bimbo, A. D., Cucchiara, R., Sclaroff, S., Farinella,
G. M., Mei, T., Bertini, M., Escalante, H. J., and
Vezzani, R., editors, Pattern Recognition. ICPR Inter-
national Workshops and Challenges - Virtual Event,
January 10-15, 2021, Proceedings, Part VI, volume
12666 of Lecture Notes in Computer Science, pages
500–515. Springer.
Jr., J. S. D., Bowyer, K. W., and Flynn, P. J. (2013). Vari-
ation in accuracy of textured contact lens detection
based on sensor and lens pattern. In IEEE Sixth In-
ternational Conference on Biometrics: Theory, Appli-
cations and Systems, BTAS 2013, Arlington, VA, USA,
September 29 - October 2, 2013, pages 1–7.
Kirchner, M. and Johnson, C. (2020). SPN-CNN: boost-
ing sensor-based source camera attribution with deep
learning. CoRR, abs/2002.02927.
Kohli, N., Yadav, D., Vatsa, M., and Singh, R. (2013). Re-
visiting iris recognition with color cosmetic contact
lenses. In International Conference on Biometrics,
ICB 2013, 4-7 June, 2013, Madrid, Spain, pages 1–
7.
Li, R., Li, C., and Guan, Y. (2018). Inference of a compact
representation of sensor fingerprint for source camera
identification. Pattern Recognition, 74:556–567.
Luk
´
as, J., Fridrich, J. J., and Goljan, M. (2006). Digital
camera identification from sensor pattern noise. IEEE
Trans. Information Forensics and Security, 1(2):205–
214.
Mandelli, S., Cozzolino, D., Bestagini, P., Verdoliva, L.,
and Tubaro, S. (2020). Cnn-based fast source device
identification. IEEE Signal Process. Lett., 27:1285–
1289.
MICHE (2019). Miche database, http://biplab.unisa.it/
miche/database/. Online; accessed 1 December 2019.
Optyczne (2023). Optyczne.pl, https://www.optyczne.pl/.
Online; accessed 5 April 2023.
Shaya, O. A., Yang, P., Ni, R., Zhao, Y., and Piva, A. (2018).
A new dataset for source identification of high dy-
namic range images. Sensors, 18(11):3801.
Shullani, D., Fontani, M., Iuliani, M., Shaya, O. A., and
Piva, A. (2017). VISION: a video and image dataset
for source identification. EURASIP J. Information Se-
curity, 2017:15.
Tuama, A., Comby, F., and Chaumont, M. (2016). Cam-
era model identification with the use of deep convolu-
tional neural networks. In IEEE International Work-
shop on Information Forensics and Security, WIFS
2016, Abu Dhabi, United Arab Emirates, December
4-7, 2016, pages 1–6. IEEE.
Yao, H., Qiao, T., Xu, M., and Zheng, N. (2018). Ro-
bust multi-classifier for camera model identification
based on convolution neural network. IEEE Access,
6:24973–24982.
SECRYPT 2023 - 20th International Conference on Security and Cryptography
804