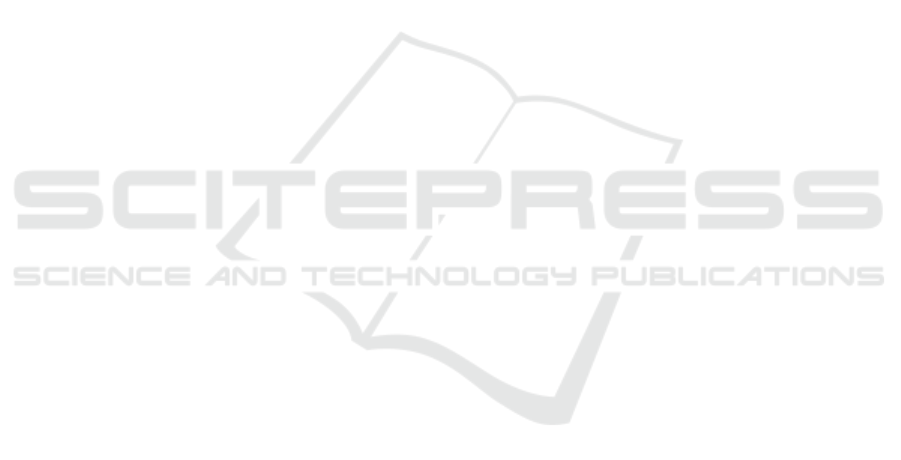
this dataset, respectively, and usability recommenda-
tion (See Table 3).
5 CONCLUSION
Both sectors, including research and the industry,
have shown incredible concerns about vehicular net-
work security. Therefore, intra-vehicular network se-
curity needs to be addressed as well. In accordance
with the current solutions, studying intra -vehicular
security datasets will provide a strong base for the re-
search and development to acquire valuable enhanced
solutions. This paper is devoted to presenting a com-
prehensive study of various intra-vehicular network
security datasets and their related quality measures.
In addition, this study addresses the major phase of
datasets, which is preprocessing. Moreover, it exam-
ines the available existing datasets and presents their
impact through comparative analyses that show their
benefits and limitations.
REFERENCES
Aksu, D. and Aydin, M. A. (2022). Mga-ids: Optimal fea-
ture subset selection for anomaly detection framework
on in-vehicle networks-can bus based on genetic algo-
rithm and intrusion detection approach. Computers &
Security, 118:102717.
Al-Jarrah, O. Y., Maple, C., Dianati, M., Oxtoby, D., and
Mouzakitis, A. (2019). Intrusion detection systems
for intra-vehicle networks: A review. IEEE Access,
7:21266–21289.
Bi, Z., Xu, G., Xu, G., Tian, M., Jiang, R., and Zhang, S.
(2022). Intrusion detection method for in-vehicle can
bus based on message and time transfer matrix. Secu-
rity and Communication Networks, 2022.
Chiscop, I., Gazdag, A., Bosman, J., and Bicz
´
ok, G. (2021).
Detecting message modification attacks on the can bus
with temporal convolutional networks. arXiv preprint
arXiv:2106.08692.
Duo, W., Zhou, M., and Abusorrah, A. (2022). A survey
of cyber attacks on cyber physical systems: Recent
advances and challenges. IEEE/CAA Journal of Auto-
matica Sinica, 9(5):784–800.
Dupont, G., Den Hartog, J., Etalle, S., and Lekidis, A.
(2019). Evaluation framework for network intrusion
detection systems for in-vehicle can. In 2019 IEEE
International Conference on Connected Vehicles and
Expo (ICCVE), pages 1–6. IEEE.
Foruhandeh, M., Man, Y., Gerdes, R., Li, M., and Chantem,
T. (2019). Simple: Single-frame based physical
layer identification for intrusion detection and preven-
tion on in-vehicle networks. In Proceedings of the
35th annual computer security applications confer-
ence, pages 229–244.
Group, T. S. (2019). Eindhoven university of technology.
Haddaji, A., Ayed, S., and Fourati, L. C. (2022). Artifi-
cial intelligence techniques to mitigate cyber-attacks
within vehicular networks: Survey. Computers and
Electrical Engineering, 104:108460.
Han, M. L., Kwak, B. I., and Kim, H. K. (2018). Anomaly
intrusion detection method for vehicular networks
based on survival analysis. Vehicular communica-
tions, 14:52–63.
Hanselmann, M., Strauss, T., Dormann, K., and Ulmer, H.
(2020). Canet: An unsupervised intrusion detection
system for high dimensional can bus data. Ieee Access,
8:58194–58205.
Jichici, C., Groza, B., Ragobete, R., Murvay, P.-S., and An-
dreica, T. (2022). Effective intrusion detection and
prevention for the commercial vehicle sae j1939 can
bus. IEEE Transactions on Intelligent Transportation
Systems, 23(10):17425–17439.
Lee, H., Jeong, S. H., and Kim, H. K. (2017). Otids: A
novel intrusion detection system for in-vehicle net-
work by using remote frame. In 2017 15th An-
nual Conference on Privacy, Security and Trust (PST),
pages 57–5709. IEEE.
Rajapaksha, S., Kalutarage, H., Al-Kadri, M. O., Petrovski,
A., Madzudzo, G., and Cheah, M. (2023). Ai-based
intrusion detection systems for in-vehicle networks: A
survey. ACM Computing Surveys, 55(11):1–40.
Rathore, R. S., Hewage, C., Kaiwartya, O., and Lloret,
J. (2022). In-vehicle communication cyber security:
challenges and solutions. Sensors, 22(17):6679.
Seo, E., Song, H. M., and Kim, H. K. (2018). Gids: Gan
based intrusion detection system for in-vehicle net-
work. In 2018 16th Annual Conference on Privacy,
Security and Trust (PST), pages 1–6. IEEE.
Shah, Z., Ullah, I., Li, H., Levula, A., and Khurshid, K.
(2022). Blockchain based solutions to mitigate dis-
tributed denial of service (ddos) attacks in the internet
of things (iot): A survey. Sensors, 22(3):1094.
Shokravi, H., Shokravi, H., Bakhary, N., Heidarrezaei,
M., Rahimian Koloor, S. S., and Petr
˚
u, M. (2020).
A review on vehicle classification and potential
use of smart vehicle-assisted techniques. Sensors,
20(11):3274.
Verma, M. E., Iannacone, M. D., Bridges, R. A., Hollifield,
S. C., Kay, B., and Combs, F. L. (2020). Road: The
real ornl automotive dynamometer controller area net-
work intrusion detection dataset (with a comprehen-
sive can ids dataset survey & guide). arXiv preprint
arXiv:2012.14600.
SIMULTECH 2023 - 13th International Conference on Simulation and Modeling Methodologies, Technologies and Applications
408