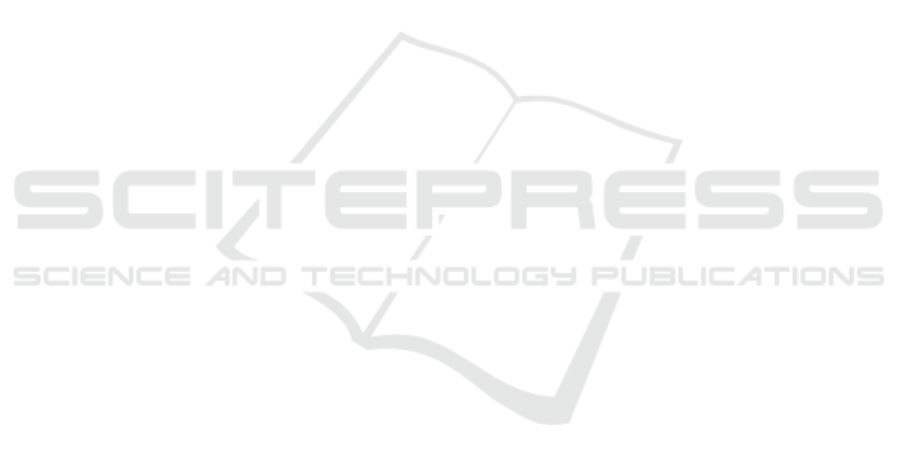
most used application protocols in the IoT scenario,
and both normal and malicious traffic, trying to iden-
tify four different attacks by using application-layer
packet features. This has demonstrated the full feasi-
bility in using synthetic traffic produced by IoT-Flock
as a base for IoT anomaly detection.
As regards future developments, we will try to
train the models on the synthetic traffic produced by
IoT-Flock and perform the testing phase on real la-
beled IoT traffic. Moreover, we will perform feature
selection to verify whether reducing the number of
considered features can lead to similar very high re-
sults.
REFERENCES
CVE-2016-10523, Common Enumeration of Vulner-
abilities. https://www.cve.org/CVERecord?id=
CVE-2016-10523. Accessed: 2023-01-30.
CVE-2019-12101, Common Enumeration of Vulnera-
bilities. https://www.cve.org/CVERecord?id=CVE-
2019-12101. Accessed: 2023-01-30.
CVE-2019-9004, Common Enumeration of Vulnerabil-
ities. https://www.cve.org/CVERecord?id=CVE-
2019-9004. Accessed: 2023-01-30.
Alrashdi, I., Alqazzaz, A., Alharthi, R., Aloufi, E., Zohdy,
M. A., and Ming, H. (2019). Fbad: Fog-based at-
tack detection for iot healthcare in smart cities. In
2019 IEEE 10th Annual Ubiquitous Computing, Elec-
tronics & Mobile Communication Conference (UEM-
CON), pages 0515–0522. IEEE.
Aversano, L., Bernardi, M. L., Cimitile, M., and Pecori, R.
(2021a). Anomaly detection of actual iot traffic flows
through deep learning. In 2021 20th IEEE Interna-
tional Conference on Machine Learning and Applica-
tions (ICMLA), pages 1736–1741.
Aversano, L., Bernardi, M. L., Cimitile, M., Pecori, R., and
Veltri, L. (2021b). Effective anomaly detection using
deep learning in IoT systems. Wireless Communica-
tions and Mobile Computing, 2021:1–14.
CAIDA (2023). Center for applied internet data analysis
(caida). ”https://catalog.caida.org/.
COAP (2014). The Constrained Application Protocol
(CoAP). Internet Engineering Task Force (IETF). Up-
dated by: RFC 7959, 8613, 8974, 9175.
DARPA (1998). Darpa. https://www.ll.mit.edu/r-d/datasets/
1998-darpa-intrusion-detection-evaluation-dataset.
Defcon (2023). https://defcon.org/html/links/dc-ctf.html.
Ghazanfar, S., Hussain, F., Rehman, A. U., Fayyaz, U. U.,
Shahzad, F., and Shah, G. A. (2020). Iot-flock: An
open-source framework for iot traffic generation. In
2020 International Conference on Emerging Trends in
Smart Technologies (ICETST), pages 1–6.
Hossain, E., Khan, I., Un-Noor, F., Sikander, S. S., and
Sunny, M. S. H. (2019). Application of big data and
machine learning in smart grid, and associated se-
curity concerns: A review. IEEE Access, 7:13960–
13988.
Hussain, F., Abbas, S. G., Shah, G. A., Pires, I. M., Fayyaz,
U. U., Shahzad, F., Garcia, N. M., and Zdravevski, E.
(2021). A framework for malicious traffic detection in
iot healthcare environment. Sensors, 21(9):3025.
KDD (1998). Kdd cup 1999 data. http://kdd.ics.uci.edu/
databases/kddcup99/kddcup99.html.
Kingma, D. P. and Ba, J. (2014). Adam: A method for
stochastic optimization.
Koroniotis, N., Moustafa, N., Sitnikova, E., and Turnbull,
B. (2019). Towards the development of realistic botnet
dataset in the internet of things for network forensic
analytics: Bot-iot dataset. Future Generation Com-
puter Systems, 100:779–796.
LBNL (2005). Lbnl/icsi enterprise tracing project. http:
//www.icir.org/enterprise-tracing/.
MQTT (2019). MQTT Version 5.0. OASIS Standard. Ver-
sion 5.
NSL (1999). Nsl-kdd dataset. htps://www.unb.ca/cic/
datasets/nsl.html.
Pecori, R., Tayebi, A., Vannucci, A., and Veltri, L. (2020).
Iot attack detection with deep learning analysis. In
2020 International Joint Conference on Neural Net-
works (IJCNN), pages 1–8.
Pundir, S., Wazid, M., Singh, D. P., Das, A. K., Rodrigues,
J. J., and Park, Y. (2019). Intrusion detection proto-
cols in wireless sensor networks integrated to internet
of things deployment: Survey and future challenges.
IEEE Access, 8:3343–3363.
Rathore, S. and Park, J. H. (2018). Semi-supervised learn-
ing based distributed attack detection framework for
iot. Applied Soft Computing, 72:79–89.
Rughoobur, P. and Nagowah, L. (2017). A lightweight
replay attack detection framework for battery de-
pended iot devices designed for healthcare. In 2017
International Conference on Infocom Technologies
and Unmanned Systems (Trends and Future Direc-
tions)(ICTUS), pages 811–817. IEEE.
Shamir, O. (2022). The implicit bias of benign overfit-
ting. In Loh, P.-L. and Raginsky, M., editors, Pro-
ceedings of Thirty Fifth Conference on Learning The-
ory, volume 178 of Proceedings of Machine Learning
Research, pages 448–478. PMLR.
UNIBS (2009). Unibs: Data sharing. http://netweb.ing.
unibs.it/
∼
ntw/tools/traces/index.php.
DATA 2023 - 12th International Conference on Data Science, Technology and Applications
182