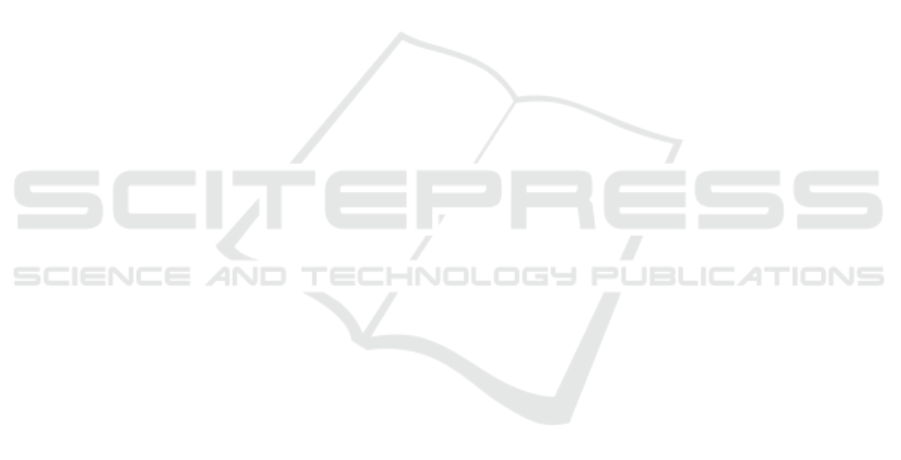
data. It surpasses the available model in accuracy
and false positive rate. Additionally, we consider the
time series data factor through LSTM, unlike other
proposed models. Therefore, it is a first of its kind
for anomaly detection of smart meter data, keeping in
mind their resource constrained nature. In the near
future, we would focus more on the causes of anoma-
lies like anomalies due to faulty meter and anomalies
caused by theft using LSTM-DAE. Thereby, specifi-
cally focusing on anomaly due to attacks and not due
to meter faults.
REFERENCES
Cui, L., Guo, L., Gao, L., Cai, B., Qu, Y., Zhou, Y.,
and Yu, S. (2021). A covert electricity-theft cyber-
attack against machine learning-based detection mod-
els. IEEE Transactions on Industrial Informatics.
Erkin, Z., Troncoso-Pastoriza, J. R., Lagendijk, R. L., and
P
´
erez-Gonz
´
alez, F. (2013). Privacy-preserving data
aggregation in smart metering systems: An overview.
IEEE Signal Processing Magazine, 30(2):75–86.
Fan, Y., Zhang, Z., Trinkle, M., Dimitrovski, A. D., Song,
J. B., and Li, H. (2015). A cross-layer defense mech-
anism against gps spoofing attacks on pmus in smart
grids. IEEE Transactions on Smart Grid, 6(6):2659–
2668.
Givnan, S., Chalmers, C., Fergus, P., Ortega-Martorell, S.,
and Whalley, T. (2022). Anomaly detection using au-
toencoder reconstruction upon industrial motors. Sen-
sors, 22(9):3166.
Goldstein, M. and Uchida, S. (2016). A comparative eval-
uation of unsupervised anomaly detection algorithms
for multivariate data. PloS one, 11(4):e0152173.
Hart, D. G. (2008). Using ami to realize the smart
grid. In Power and energy society general meeting-
Conversion and delivery of electrical energy in the
21st Century, pages 1–2.
Huang, Y. and Xu, Q. (2021). Electricity theft detection
based on stacked sparse denoising autoencoder. Inter-
national Journal of Electrical Power & Energy Sys-
tems, 125:106448.
Illera, A. G. and Vidal, J. V. (2014). Lights off! the darkness
of the smart meters. BlackHat Europe.
Knirsch, F., Eibl, G., and Engel, D. (2016). Error-
resilient masking approaches for privacy preserving
data aggregation. IEEE Transactions on Smart Grid,
9(4):3351–3361.
Li, M., Zhang, K., Liu, J., Gong, H., and Zhang, Z.
(2020). Blockchain-based anomaly detection of elec-
tricity consumption in smart grids. Pattern Recogni-
tion Letters, 138:476–482.
Lindemann, B., Maschler, B., Sahlab, N., and Weyrich, M.
(2021). A survey on anomaly detection for technical
systems using lstm networks. Computers in Industry,
131:103498.
Lo, C.-H. and Ansari, N. (2013). Consumer: A novel hybrid
intrusion detection system for distribution networks in
smart grid. IEEE Transactions on Emerging Topics in
Computing, 1(1):33–44.
Merrill, N. and Eskandarian, A. (2020). Modified autoen-
coder training and scoring for robust unsupervised
anomaly detection in deep learning. IEEE Access,
8:101824–101833.
Mitchell, R. and Chen, R. (2013). Behavior-rule based
intrusion detection systems for safety critical smart
grid applications. IEEE Transactions on Smart Grid,
4(3):1254–1263.
Nagi, J., Yap, K. S., Tiong, S. K., Ahmed, S. K., and Mo-
hamad, M. (2009). Nontechnical loss detection for
metered customers in power utility using support vec-
tor machines. IEEE transactions on Power Delivery,
25(2):1162–1171.
Nizar, A., Dong, Z., and Wang, Y. (2008). Power utility
nontechnical loss analysis with extreme learning ma-
chine method. IEEE Transactions on Power Systems,
23(3):946–955.
Sheikh, N. U., Asghar, H. J., Farokhi, F., and Kaafar, M. A.
(2021). Do auto-regressive models protect privacy in-
ferring fine-grained energy consumption from aggre-
gated model parameters. IEEE Transactions on Ser-
vices Computing.
Tellbach, D. and Li, Y.-F. (2018). Cyber-attacks on smart
meters in household nanogrid: modeling, simulation
and analysis. Energies, 11(2):316.
Vincent, P., Larochelle, H., Bengio, Y., and Manzagol, P.-
A. (2008). Extracting and composing robust features
with denoising autoencoders. In Proceedings of the
25th international conference on Machine learning,
pages 1096–1103.
Wang, K., Du, M., Maharjan, S., and Sun, Y. (2017). Strate-
gic honeypot game model for distributed denial of ser-
vice attacks in the smart grid. IEEE Transactions on
Smart Grid, 8(5):2474–2482.
Wueest, C. (2014). Targeted attacks against the energy sec-
tor. Symantec Security Response, Mountain View, CA.
Yi, P., Zhu, T., Zhang, Q., Wu, Y., and Pan, L. (2016). Pup-
pet attack: A denial of service attack in advanced me-
tering infrastructure network. Journal of Network and
Computer Applications, 59:325–332.
Yip, S.-C., Tan, W.-N., Tan, C., Gan, M.-T., and Wong, K.
(2018). An anomaly detection framework for identi-
fying energy theft and defective meters in smart grids.
International Journal of Electrical Power & Energy
Systems, 101:189–203.
Yip, S.-C., Wong, K., Hew, W.-P., Gan, M.-T., Phan, R. C.-
W., and Tan, S.-W. (2017). Detection of energy theft
and defective smart meters in smart grids using linear
regression. International Journal of Electrical Power
& Energy Systems, 91:230–240.
Zhou, G., Liu, M., and Liu, X. (2021). An autoencoder-
based model for forest disturbance detection using
landsat time series data. International Journal of Dig-
ital Earth, 14(9):1087–1102.
Anomaly Detection in Smart Grid Networks Using Power Consumption Data
837