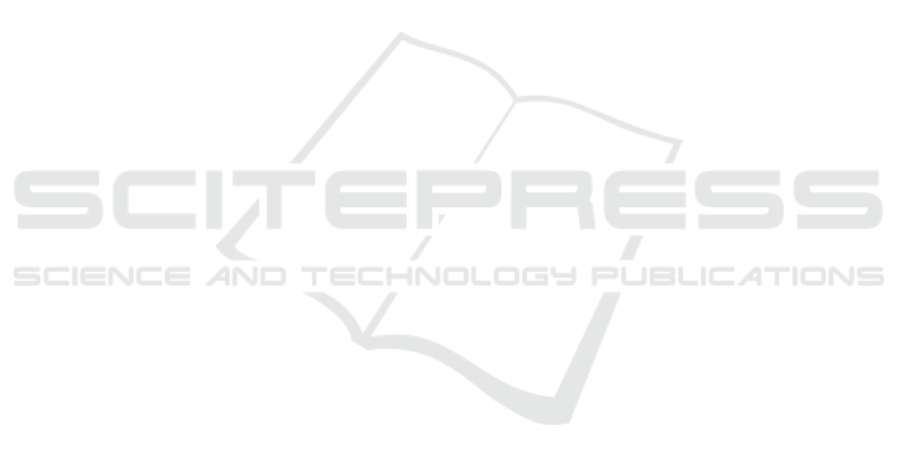
attacks in software defined networks. Journal of Net-
work and Computer Applications, 177:102942.
Bhardwaj, A., Mangat, V., and Vig, R. (2020). Hyperband
tuned deep neural network with well posed stacked
sparse autoencoder for detection of DDoS attacks in
cloud. IEEE Access, 8:181916–181929.
Chartuni, A. and M
´
arquez, J. (2021). Multi-classifier of
DDoS attacks in computer networks built on neural
networks. Applied Sciences, 11(22):10609.
CIC (2018). Cse-cic-ids-2018.
https://www.unb.ca/cic/datasets/ids-2018.html.
CIC (2019). Cic-ddos-2019.
https://www.unb.ca/cic/datasets/ddos-2019.html.
Cil, A. E., Yildiz, K., and Buldu, A. (2021). Detection
of DDoS attacks with feed forward based deep neu-
ral network model. Expert Systems with Applications,
169:114520.
de Assis, M. V., Carvalho, L. F., Rodrigues, J. J., Lloret,
J., and Proenc¸a Jr, M. L. (2020). Near real-time secu-
rity system applied to SDN environments in IoT net-
works using convolutional neural network. Computers
& Electrical Engineering, 86:106738.
Doriguzzi-Corin, R., Millar, S., Scott-Hayward, S.,
Martinez-del Rincon, J., and Siracusa, D. (2020). LU-
CID: A practical, lightweight deep learning solution
for DDoS attack detection. IEEE Trans. on Network
and Service Management, 17(2):876–889.
Elsayed, M. S., Le-Khac, N.-A., Dev, S., and Jurcut, A. D.
(2020). DDoSNet: A deep-learning model for detect-
ing network attacks. In Proc. of the 2020 IEEE 21st
Int. Symp. on ”A World of Wireless, Mobile and Mul-
timedia Networks” (WoWMoM), pages 391–396.
Ferrag, M. A., Shu, L., Djallel, H., and Choo, K.-K. R.
(2021). Deep learning-based intrusion detection for
distributed denial of service attack in agriculture 4.0.
Electronics, 10(11).
G
¨
um
¨
us¸bas¸, D., Yıldırım, T., Genovese, A., and Scotti,
F. (2021). A comprehensive survey of databases
and deep learning methods for cybersecurity and in-
trusion detection systems. IEEE Systems Journal,
15(2):1717–1731.
Haider, S., Akhunzada, A., Mustafa, I., Patel, T. B., Fernan-
dez, A., Choo, K.-K. R., and Iqbal, J. (2020). A deep
CNN ensemble framework for efficient DDoS attack
detection in software defined networks. IEEE Access,
8:53972–53983.
Kasim,
¨
O. (2020). An efficient and robust deep learn-
ing based network anomaly detection against dis-
tributed denial of service attacks. Computer Networks,
180:107390.
Kim, J., Kim, J., Kim, H., Shim, M., and Choi,
E. (2020). CNN-based network intrusion detec-
tion against denial-of-service attacks. Electronics,
9(6):916.
Lashkari, A. H., Draper-Gil, G., Mamun, M. S. I., Ghor-
bani, A. A., et al. (2017). Characterization of tor traf-
fic using time based features. In Proc. of the 3rd Int.
Conf. on Information Systems Security and Privacy
(ICISSP), pages 253–262.
Liang, X. and Znati, T. (2019). A long short-term mem-
ory enabled framework for DDoS detection. In
Proc. of the 2019 IEEE Global Communications Conf.
(GLOBECOM), pages 1–6.
Minka, T. (2000). Automatic choice of dimensionality for
PCA. In Leen, T., Dietterich, T., and Tresp, V., editors,
Advances in Neural Information Processing Systems,
volume 13.
Mittal, M., Kumar, K., and Behal, S. (2022). Deep learning
approaches for detecting DDoS attacks: A systematic
review. Soft Computing, pages 1–37.
Roopak, M., Tian, G. Y., and Chambers, J. (2020). An
intrusion detection system against DDoS attacks in
IoT networks. In Proc. of the 2020 10th Annual
Computing and Communication Workshop and Conf.
(CCWC), pages 0562–0567.
Roopak, M., Yun Tian, G., and Chambers, J. (2019). Deep
learning models for cyber security in IoT networks.
In Proc. of the 2019 IEEE 9th Annual Computing and
Communication Workshop and Conf. (CCWC), pages
0452–0457.
Sabeel, U., Heydari, S. S., Mohanka, H., Bendhaou, Y., El-
gazzar, K., and El-Khatib, K. (2019). Evaluation of
deep learning in detecting unknown network attacks.
In Proc. of the 2019 Int. Conf. on Smart Applications,
Communications and Networking (SmartNets), pages
1–6.
Sbai, O. and El boukhari, M. (2020). Data flooding intru-
sion detection system for MANETs using deep learn-
ing approach. In Proc. of the 13th Int. Conf. on In-
telligent Systems: Theories and Applications (SITA).
Association for Computing Machinery.
Srikanth Yadav., M. and Kalpana., R. (2019). Data prepro-
cessing for intrusion detection system using encoding
and normalization approaches. In Proc. of the 2019
11th Int. Conf. on Advanced Computing (ICoAC),
pages 265–269.
Wang, L. and Liu, Y. (2020). A DDoS attack detection
method based on information entropy and deep learn-
ing in SDN. In Proc. of the 2020 IEEE 4th Information
Technology, Networking, Electronic and Automation
Control Conf. (ITNEC), pages 1084–1088.
Anomaly-Based Intrusion Detection System for DDoS Attack with Deep Learning Techniques
275