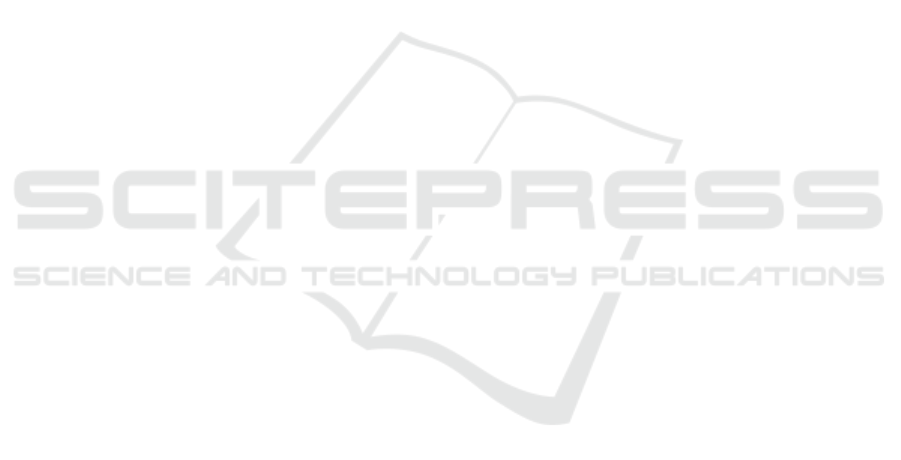
ronment, and use this information to assess their rela-
tionship with crime in street segments. To avoid that,
Forradellas and others propose in (Reier Forradel-
las and Rodriguez, 2021) a crime prediction model
through a neural network called multilayer percep-
tron in order to obtain future information not only
regarding possible crimes, but with a level of de-
tail adequate for their definition. In addition, in
(Ana Amante, 2021) it is indicated that conclusions
are drawn based on the experiences of municipalities,
police and administration, which contribute to the de-
bate on community crime prevention and highlight
the need for multidisciplinary, multilevel and place-
specific approaches. Likewise, Janakiramaiah and
others (B. Janakiramaiah, 2021) describes and pro-
poses an automated method for detecting abnormal
human behavior in intelligent surveillance systems.
On the other hand, in (Kimihiro Hino, 2021) Hino
and Chronopoulos reviews crime prevention policies
in the Adachi district where the Beautiful Windows
Movement and Action Plan is discussed. In another
case, as stated in (William E, 2020) by William and
others, it was developed a detection algorithm that
incorporated facets of teacher-reported outsourcing
problems and other known risk factors. We exam-
ined detection approaches based on logistic regres-
sion and machine learning algorithms. While it is
true that, Van Steden (Steden, 2021) based his re-
search on categorizing the following items: effect,
mechanisms, moderators, implementations, and eco-
nomics. It was concluded that these groups can gen-
erate a greater problem for citizens, since they try to
confront crime directly (without the presence of au-
thorities), which can generate the exposure of more
people and lead to new crimes. Communication and
technology can be a good way to support against the
crime rate they are facing in the Netherlands. In addi-
tion, in (Hongjie Yu and Lan, 2020) it is demonstrated
the complexity of the spatial and temporal distribu-
tion of criminal activities and stressed that the con-
struction of covariates based on classical crime the-
ory and fine-scale data are effective for crime predic-
tion. Another research by Niu and others (Niu, 2019),
is based on being able to create, test and compare
crime prediction algorithms based on the patterns of
criminal activity and why they are influenced in the
community areas of the city of Chicago. In addition,
K-means (KNN), decision tree (DT), Naive Bayes
(NB) and Support Vector Machine (SVM) algorithms
were used. Moreover, in (Wajiha Safat, 2021) is de-
scribed improved efficiency for accurate crime pre-
diction compared to what was previously achieved
with additional analysis based on different machine
learning algorithms. In addition, Albahli and others
(Albahli, 2021) propose a prediction method using
Machine Learning technology (Naive Bayes, Random
Forest, KNN, Decision Tree, Deep Learning) and se-
lection methods such as: FAMD (Mixed Data Factor
Analysis and PCA (Principal Component Analysis).
Also, the proposed method has as its main objective to
predict the factors that most affected crimes in Saudi
Arabia. In addition, in (Myung-Sun Baek and Lee,
2021) MYUNG-SUN and others reports that differ-
ent prediction models were developed to detect the
type of crime, of which respective tests were made
to verify their performance and authentication at the
time of analysis of criminal cases. It was verified
that their differences are minimal, ranging between
7% and 8% difference in results, and that they can be
viable for the use of case analysis. On the other hand,
in (Obagbuwa and Abidoye, 2021) is indicated that
crime data analysis can extract vital unknown infor-
mation from raw data and thus help the government
speed up procedures to solve crimes. It would en-
able the relevant government authorities to gain a bet-
ter understanding of crime trends and mitigate them.
When crime is prevented it can boost different eco-
nomic areas and attract more people to invest in the
locality. Along with, Kim and others (Kim, 2021) in-
dicate that using predictive technology in geographic
areas where they suffer from burglary will reduce the
triggering of potential burglaries in areas surround-
ing the burglarized areas. Likewise, Verma and others
(Verma, 2021) perform model training, validation and
testing using the Random Forest and Gradient Boost
Machine (GBM) ensemble approach with a hyper-
parameter optimizer using the “CSE-CIC-IDS2018-
V2” dataset and demonstrating performance testing
with attack categories such as infiltration, SQL In-
jection, etc. In (Aziz and Kumar, 2022) Aziz, Hus-
sain and others detail a Machine Learning based soft
computing regression analysis approach for analyzing
crime data occurred in India. Different regression al-
gorithms will be used, which are simple linear regres-
sion, multiple linear regression, decision tree regres-
sion, support vector regression, and random forest re-
gression. Also, in (Machin, 2021) is indicated that
privacy and security of shared information in cogni-
tive cities become critical issues that need to be ad-
dressed to ensure the proper deployment of cogni-
tive cities and the fundamental rights of individuals.
Dahlstedt and Foultier (Dahlstedt and Foultier, 2021)
point out as a point of improvement the promotion of
peer safety and the feeling of support among citizens,
and as a specific approach, schools and municipali-
ties are mentioned as key points where important cit-
izen information can be imparted to reduce the crime
rate. Likewise, in (Chaparro L., 2021) Chaparro and
ICINCO 2023 - 20th International Conference on Informatics in Control, Automation and Robotics
116