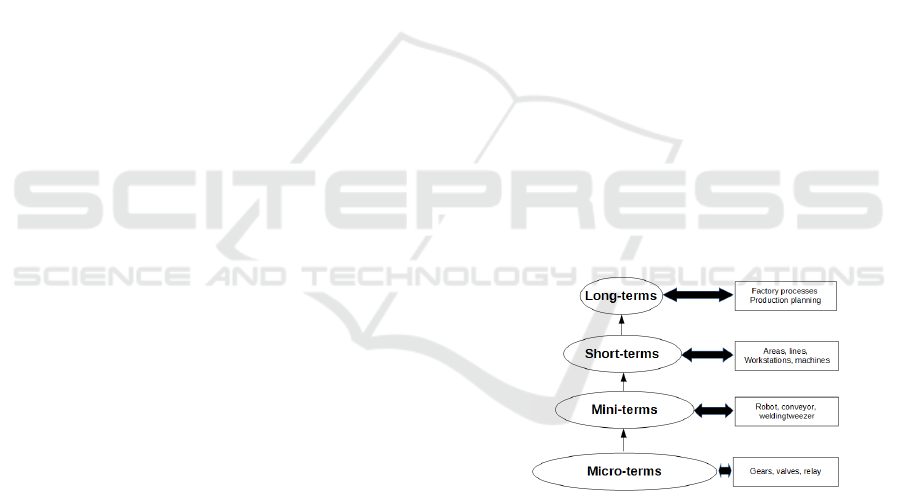
of substantial advantages. These encompass cost re-
duction, heightened operational efficiency, enhanced
productivity, reduced errors, and bolstered security
(Ghobakhloo, 2020). Furthermore, the amalgamation
of these technologies contributes to sustainability ef-
forts and bolsters environmental preservation.
Despite the undeniable benefits, the implementa-
tion of Industry 4.0 and advanced scheduling solu-
tions is not without its challenges. Concerns span cy-
bersecurity vulnerabilities (Rajalingham, 2020), skills
and training deficits (Bauernhansl et al., 2014), inter-
operability issues (Wang et al., 2016), and substantial
initial investments (Bauernhansl et al., 2014).
In this transformative landscape, sensorization, as
a critical component of IIoT (Peinado-Asensi et al.,
2023a), plays a pivotal role in redefining how pro-
duction scheduling is executed using the philosophy
and the results of our previous works (Garcia, 2022;
Llopis, 2022). By enabling real-time data collec-
tion from various operational facets, sensorization
empowers decision-makers with essential insights to
optimize Production Scheduling processes efficiently.
However, sensorization in IIoT is not without its chal-
lenges, including managing and analyzing vast vol-
umes of data, industrial cybersecurity, and interoper-
ability between systems and devices (Peinado-Asensi
et al., 2023b).
1.1 Previous Works
In our previous works, (Peinado-Asensi et al., 2023a;
Peinado-Asensi et al., 2023b), a new concept for gen-
erating industrializable IIoT applications, called In-
dustrializable Industrial Internet of Things (I3oT )
was presented. As we briefly explain in the introduc-
tion, there is an important limitation that is signifi-
cantly slowing down its massive proliferation in the
IIoT application industry. The installation of sensors,
their wiring and data extraction through the IT net-
work to the OT network, and the increasing number
of machines or components to be sensorized prevent
the proposed solutions from being applied in the in-
dustry in a massive way, due to the high cost involved
in their implementation. The idea of the (I3oT ) is to
use the installation available in factories to develop
IIoT applications from them. The machines installed
in the industry operate automatically and have sen-
sors that provide the information received by the PLC
to control the lines.
1.1.1 Miniterm-Based Big Data for Predictive
Maintenance
Previous works carried out by the research group
following the (I3oT ) philosophy was in (Garcia,
2022)where we have presented an innovative solu-
tion for the early detection of faults in industrial ma-
chinery by using a virtual sensor called Mini-term de-
fined in (Garc
´
ıa, 2016), see figure 1. The mathemat-
ical model proposed in (Garc
´
ıa, 2016) was reformu-
lated in (Garc
´
ıa and Mont
´
es, 2017), by using tensor
algebra, which reduces the computational cost of the
model, especially when the number of mini-terms and
micro-terms is high. The Miniterm is based on the
measurement of the technical cycle time as a param-
eter to predict failure. When the component is ap-
proaching the end of its useful life, the cycle time in-
creases alerting that it must be replaced. The great ad-
vantage of the miniterm is that it does not require the
installation of any additional sensor, but uses the sen-
sorization of the machine’s own automatic system and
only requires the programming of a timer in the PLC.
In (Garcia, 2022) a case study was presented in which
the Miniterm was implemented in a production line of
a vehicle manufacturing company, in particular, in the
Ford factory based in Almussafes (Valencia). In this
factory there are more than 24,000 mini-terms moni-
toring cylinders, clamps, elevators, screwdrivers, etc.
The results showed that the virtual sensor could de-
tect anomalies, which allowed the maintenance team
to take preventive measures in order to avoid the stop-
page of the production line. This fact has made that
different indicators of the plant, among which is the
TAV (Technical Availability), increased significantly,
see (Garcia, 2022).
Figure 1: From the micro-term to the long-term.
1.1.2 Manufacturing Maps for Smart Factory
Management
In the study by (Llopis, 2022) a new tool called Man-
ufacturing Maps is described which is a smart fac-
tory management tool that relies on the combination
of Petri nets and big data mini-terms.
A manufacturing map is a hierarchical construc-
tion of Petri nets in which the lowest level net is a
temporary Petri net based on mini-terms, and in which
the highest level is a global view of the entire plant.
The manufacturing map is fed by Big Data based on
miniterms, which allows it to have in real time the
ICINCO 2023 - 20th International Conference on Informatics in Control, Automation and Robotics
274