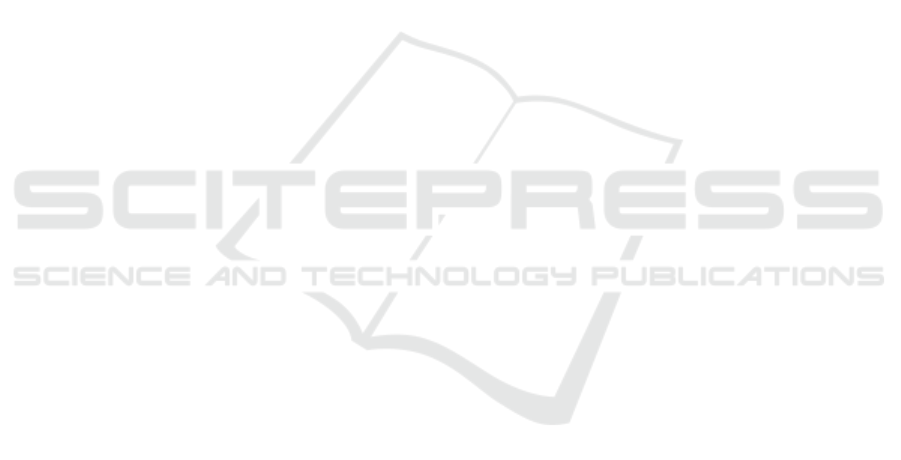
tation indices and centrality. Procedia Computer Sci-
ence, 57:606–613.
Breiman, L. (2001). Random forests. Machine learning,
45(1):5–32.
Chung, D. (2023). Machine learning for predictive model in
entrepreneurship research: predicting entrepreneurial
action. Small Enterprise Research, pages 1–18.
crunchbase.com (2007). Crunchbase: Discover innovative
companies and the people behind them.
Fern
´
andez-Delgado, M., Cernadas, E., Barro, S., and
Amorim, D. (2014). Do we need hundreds of classi-
fiers to solve real world classification problems? The
journal of machine learning research, 15(1):3133–
3181.
Ferrati, F., Muffatto, M., et al. (2021). Entrepreneurial
finance: emerging approaches using machine learn-
ing and big data. Foundations and Trends® in En-
trepreneurship, 17(3):232–329.
Gonz
´
alez-Pern
´
ıa, J. L., Kuechle, G., and Pe
˜
na-Legazkue,
I. (2013). An assessment of the determinants of uni-
versity technology transfer. Economic Development
Quarterly, 27(1):6–17.
Hirsch, J. E. (2005). An index to quantify an individual’s
scientific research output. Proceedings of the National
academy of Sciences, 102(46):16569–16572.
Hook, D. W., Porter, S. J., and Herzog, C. (2018). Di-
mensions: Building context for search and evaluation.
Frontiers in Research Metrics and Analytics, 3:23.
Karnani, F. (2013). The university’s unknown knowledge:
Tacit knowledge, technology transfer and university
spin-offs findings from an empirical study based on
the theory of knowledge. The Journal of Technology
Transfer, 38(3):235–250.
Kotsiantis, S. B. (2013). Decision trees: a recent overview.
Artificial Intelligence Review, 39(4):261–283.
Li, T. and Zhou, M. (2016). Ecg classification using
wavelet packet entropy and random forests. Entropy,
18(8):285.
Montebruno, P., Bennett, R. J., Smith, H., and Van Lieshout,
C. (2020). Machine learning classification of en-
trepreneurs in british historical census data. Informa-
tion Processing & Management, 57(3):102210.
M
¨
uller, K. (2010). Academic spin-off’s transfer
speed—analyzing the time from leaving university to
venture. Research Policy, 39(2):189–199.
Olson, R. S., La Cava, W., Orzechowski, P., Urbanowicz,
R. J., and Moore, J. H. (2017). Pmlb: a large bench-
mark suite for machine learning evaluation and com-
parison. BioData mining, 10(1):1–13.
Pedregosa, F., Varoquaux, G., Gramfort, A., Michel, V.,
Thirion, B., Grisel, O., Blondel, M., Prettenhofer,
P., Weiss, R., Dubourg, V., Vanderplas, J., Passos,
A., Cournapeau, D., Brucher, M., Perrot, M., and
Duchesnay, E. (2011). Scikit-learn: Machine learning
in Python. Journal of Machine Learning Research,
12:2825–2830.
Pirnay, F., Surlemont, B., Nlemvo, F., et al. (2003). Toward
a typology of university spin-offs. Small business eco-
nomics, 21(4):355–369.
Rothaermel, F. T., Agung, S. D., and Jiang, L. (2007). Uni-
versity entrepreneurship: a taxonomy of the literature.
Industrial and corporate change, 16(4):691–791.
Sabahi, S. and Parast, M. M. (2020). The impact of en-
trepreneurship orientation on project performance: A
machine learning approach. International Journal of
Production Economics, 226:107621.
Sharchilev, B., Roizner, M., Rumyantsev, A., Ozornin,
D., Serdyukov, P., and de Rijke, M. (2018). Web-
based startup success prediction. In Proceedings of
the 27th ACM international conference on informa-
tion and knowledge management, pages 2283–2291.
Siegel, D. S. and Wright, M. (2015). Academic en-
trepreneurship: time for a rethink? British journal
of management, 26(4):582–595.
Van Burg, E., Romme, A. G. L., Gilsing, V. A., and Rey-
men, I. M. (2008). Creating university spin-offs: a
science-based design perspective. Journal of Product
Innovation Management, 25(2):114–128.
Wright, M. (2007). Academic entrepreneurship in Europe.
Edward Elgar Publishing.
˙
Zbikowski, K. and Antosiuk, P. (2021). A machine learning,
bias-free approach for predicting business success us-
ing crunchbase data. Information Processing & Man-
agement, 58(4):102555.
Discovering Potential Founders Based on Academic Background
125