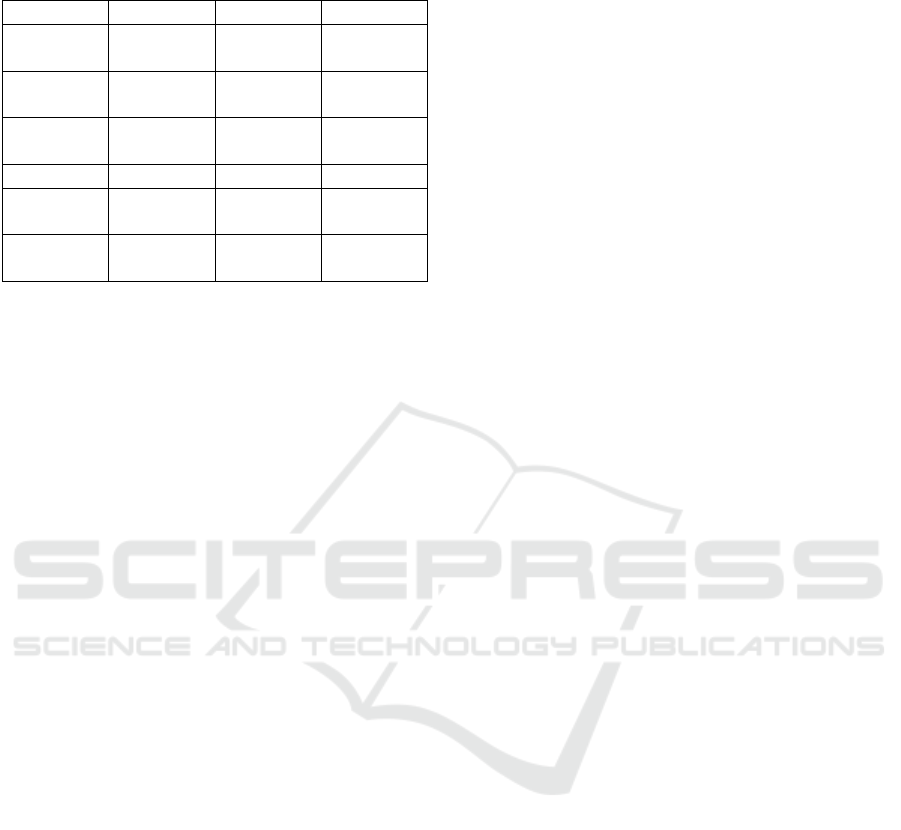
Table 8: Handball results with automatically detected match
objects.
Detector Recall Precision Corr.
Shot on
target
0,28 0,16 0,22
Shot off
target
0,17 0,04 0,11
Shot
defended
0 0 0
Penalty 0,63 0,71 0,67
Game
restart
0,86 0,40 0,63
Ball own-
ership
0,41 0,75 0,58
be emphasised that the ISVP AI prototype does not
require the construction of a dedicated camera, which
in the future may significantly reduce the costs of pro-
duction and purchase of such a system.
6 DISCUSSION AND FURTHER
RESEARCH
The prototype of the ISVP AI system proves the ef-
fectiveness of using modern methods of detecting ob-
jects in systems to support the production of sports
recordings. The obtained results also show that it is
possible to fully automate this process. Nevertheless,
the key result that was achieved is a potentially sig-
nificant reduction in the cost of production of match
recordings.
Thanks to the technology used, a system does not
require the employment of additional employees or
the purchase of specialised equipment. It is this po-
tential difference in the cost of using ISVP AI that
may have a positive impact on the practice of record-
ing futsal and handball matches. Compared to other
modern systems, the ISVP AI system manages to
achieve better results in the match object detection
metric, as well as create a completely new rule-based
system for futsal and handball event detection.
Nevertheless, further development of the ideas
presented here is still necessary. The obtained results
indicate that even relatively high results of match ob-
ject detection do not ensure high efficiency in detect-
ing more complex events. However, this is crucial
in building a virtual representation of the game state,
which is necessary to implement the basic function-
alities of the system. Hence, further research in this
area should focus primarily on improving methods of
combining the work of image processing algorithms
with expert systems.
REFERENCES
Agile Sports Technologies, I. (2021). Efficient analysis:
Record and upload automatically. https://www.hudl.
com/en_gb/products/focus.
Ariki, Y., Kubota, S., and Kumano, M. (2006). Auto-
matic production system of soccer sports video by dig-
ital camera work based on situation recognition. In
Eighth IEEE International Symposium on Multimedia
(ISM’06), pages 851–860. IEEE.
Benjumea, A., Teeti, I., Cuzzolin, F., and Bradley, A.
(2021). Yolo-z: Improving small object detection in
yolov5 for autonomous vehicles.
Bochkovskiy, A., Wang, C. Y., and Liao, H. (2016). Opti-
mal speed and accuracy of object detection.
Carion, N., Massa, F., Synnaeve, G., Usunier, N., Kirillov,
A., and Zagoruyko, S. (2020). End-to-end object de-
tection with transformers. In Computer Vision–ECCV
2020: 16th European Conference, Glasgow, UK, Au-
gust 23–28, 2020, Proceedings, Part I 16, pages 213–
229. Springer International Publishing.
Deng, J., Dong, W., Socher, R., Li, L. J., Li, K., and Fei-Fei,
L. (2009). Imagenet: A large-scale hierarchical image
database. In 2009 IEEE conference on computer vi-
sion and pattern recognition, pages 248–255.
Everingham, M., Gool, L. V., Williams, C. K., Winn, J., and
Zisserman, A. (2009). The pascal visual object classes
(voc) challenge. In International journal of computer
vision, pages 303–308.
Girshick, R. (2015). Fast r-cnn. In Proceedings of the IEEE
international conference on computer vision, pages
1440–1448.
Girshick, R., Donahue, J., Darrell, T., and Malik, J. (2013).
Rich feature hierarchies for accurate object detec-
tion and semantic segmentation. In Proceedings of
the IEEE conference on computer vision and pattern
recognition, pages 580–587.
Horvat, M. and Gledec, G. (2022). A comparative study
of yolov5 models performance for image localization
and classification. In Central European Conference on
Information and Intelligent Systems, pages 349–356.
Faculty of Organization and Informatics Varazdin.
Lin, T. Y., Dollár, P., Girshick, R., He, K., Hariharan, B.,
and Belongie, S. (2017). Feature pyramid networks
for object detection. In Proceedings of the IEEE con-
ference on computer vision and pattern recognition,
pages 2117–2125.
Lin, T. Y., Maire, M., Belongie, S., Hays, J., Perona,
P., Ramanan, D., and Zitnick, C. L. (2014). Mi-
crosoft coco: Common objects in context. In Com-
puter Vision–ECCV 2014: 13th European Confer-
ence, Zurich, Switzerland, September 6-12, 2014, Pro-
ceedings, Part V 13, pages 740–755. Springer Interna-
tional Publishing.
Quiroga, J., Maldonado, H., Ruiz, E., and Zapata, L. (2020).
As seen on tv: Automatic basketball video produc-
tion using gaussian-based actionness and game states
recognition. In Proceedings of the IEEE/CVF Con-
ference on Computer Vision and Pattern Recognition
Workshops, pages 894–895.
icSPORTS 2023 - 11th International Conference on Sport Sciences Research and Technology Support
32