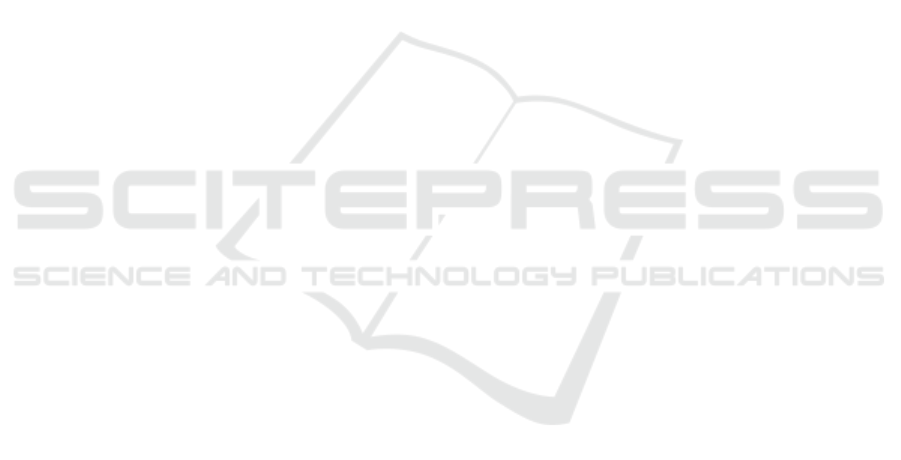
Dempster, A. P., Laird, N. M., and Rubin, D. B. (1977).
Maximum likelihood from incomplete data via the em
algorithm. Journal of the Royal Statistical Society:
Series B (Methodological), 39(1):1–22.
Garnett, R. (2022). Bayesian Optimization. Cambridge
University Press. in preparation.
Ijspeert, A. J., Nakanishi, J., Hoffmann, H., Pastor, P., and
Schaal, S. (2013). Dynamical Movement Primitives:
Learning Attractor Models for Motor Behaviors. Neu-
ral Computation, 25(2):328–373.
Kulak, T., Girgin, H., Odobez, J.-M., and Calinon, S. (2021).
Active learning of bayesian probabilistic movement
primitives. IEEE Robotics and Automation Letters,
6:2163–2170.
Lin, J. (1991). Divergence measures based on the shannon
entropy. IEEE Transactions on Information Theory,
37(1):145–151.
Pahi
ˇ
c, R., Ridge, B., Gams, A., Morimoto, J., and Ude,
A. (2020). Training of deep neural networks for the
generation of dynamic movement primitives. Neural
networks : the official journal of the International
Neural Network Society, 127:121–131.
Paraschos, A., Daniel, C., Peters, J., and Neumann, G.
(2013). Probabilistic movement primitives. In NIPS.
Pervez, A. and Lee, D. (2018). Learning task-parameterized
dynamic movement primitives using mixture of gmms.
Intelligent Service Robotics, 11:61–78.
Pervez, A., Mao, Y., and Lee, D. (2017). Learning deep
movement primitives using convolutional neural net-
works. 2017 IEEE-RAS 17th International Conference
on Humanoid Robotics (Humanoids), pages 191–197.
Rana, M. A., Chen, D., Ahmadzadeh, S. R., Williams, J.,
Chu, V., and Chernova, S. (2020). Benchmark for
skill learning from demonstration: Impact of user ex-
perience, task complexity, and start configuration on
performance. 2020 IEEE International Conference on
Robotics and Automation (ICRA), pages 7561–7567.
Sakoe, H. and Chiba, S. (1978). Dynamic programming
algorithm optimization for spoken word recognition.
IEEE Transactions on Acoustics, Speech, and Signal
Processing, 26:159–165.
Sanni, O., Bonvicini, G., Khan, M. A., L
´
opez-Custodio,
P. C., Nazari, K., and AmirM.Ghalamzan, E. (2022).
Deep movement primitives: toward breast cancer ex-
amination robot. ArXiv, abs/2202.09265.
Schaal, S. (2006). Dynamic Movement Primitives -A Frame-
work for Motor Control in Humans and Humanoid
Robotics, pages 261–280. Springer Tokyo, Tokyo.
Tosatto, S., Chalvatzaki, G., and Peters, J. (2020). Con-
textual latent-movements off-policy optimization for
robotic manipulation skills. 2021 IEEE International
Conference on Robotics and Automation (ICRA), pages
10815–10821.
Urain, J. and Peters, J. (2019). Generalized multiple correla-
tion coefficient as a similarity measurement between
trajectories. In 2019 IEEE/RSJ International Confer-
ence on Intelligent Robots and Systems (IROS), pages
1363–1369.
Welch, B. L. (1947). The generalization of ‘student’s’ prob-
lem when several different population varlances are
involved. Biometrika, 34:28–35.
Yang, Q., A. Stork, J., and Stoyanov, T. (2022). Mpr-
rl: Multi-prior regularized reinforcement learning for
knowledge transfer. IEEE Robotics and Automation
Letters, pages 1–8.
Zhou, Y., Gao, J., and Asfour, T. (2020). Movement primitive
learning and generalization: Using mixture density
networks. IEEE Robotics & Automation Magazine,
27:22–32.
TEAM: A Parameter-Free Algorithm to Teach Collaborative Robots Motions from User Demonstrations
577