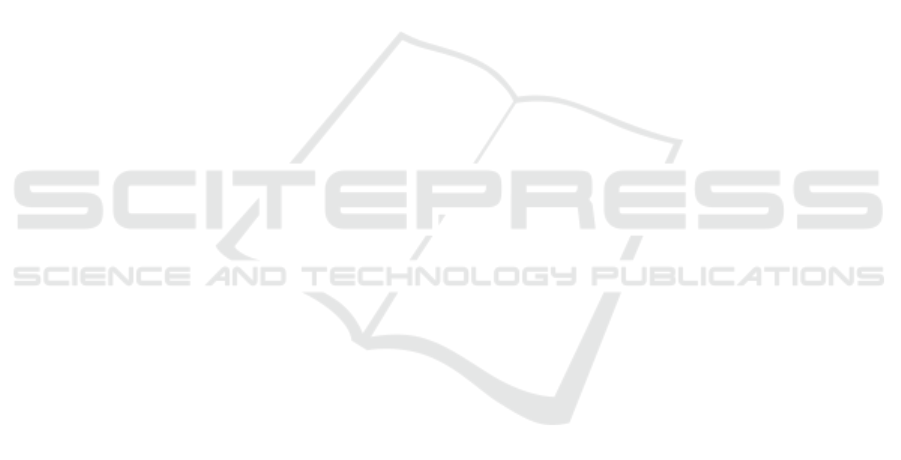
ACKNOWLEDGEMENTS
This work has been funded by the ValgrAI
Foundation, Valencian Graduate School and Re-
search Network of Artificial Intelligence through
a predoctoral grant. In addition, this publica-
tion is part of the project TED2021-130901B-I00,
funded by MCIN/AEI/10.13039/501100011033 and
by the European Union “NextGenerationE”/PRTR”
and of the projects PROMETEO/2021/075, and
GIGE/2021/150, funded by the Generalitat Valen-
ciana.
REFERENCES
Bellone, M., Reina, G., Caltagirone, L., and Wahde, M.
(2017). Learning traversability from point clouds in
challenging scenarios. IEEE Transactions on Intelli-
gent Transportation Systems, 19(1):296–305.
Chen, Z., Zhang, J., and Tao, D. (2019). Progressive lidar
adaptation for road detection. IEEE/CAA Journal of
Automatica Sinica, 6(3):693–702.
Choy, C., Gwak, J., and Savarese, S. (2019). 4D spatio-
temporal convnets: Minkowski convolutional neural
networks. In Proceedings of the IEEE/CVF con-
ference on computer vision and pattern recognition,
pages 3075–3084.
Fan, R., Wang, H., Cai, P., and Liu, M. (2020). Sne-
roadseg: Incorporating surface normal information
into semantic segmentation for accurate freespace de-
tection. In European Conference on Computer Vision,
pages 340–356. Springer.
Frey, J., Hoeller, D., Khattak, S., and Hutter, M. (2022). Lo-
comotion policy guided traversability learning using
volumetric representations of complex environments.
In 2022 IEEE/RSJ Int. Conf. on Intelligent Robots and
Systems (IROS), pages 5722–5729. IEEE.
Fusaro, D., Olivastri, E., Evangelista, D., Imperoli, M.,
Menegatti, E., and Pretto, A. (2023). Pushing the
limits of learning-based traversability analysis for au-
tonomous driving on cpu. In Intelligent Autonomous
Systems 17: Proceedings of the 17th Int. Conf. IAS-17,
pages 529–545. Springer.
Geiger, A., Lenz, P., and Urtasun, R. (2012). Are we ready
for autonomous driving? the kitti vision benchmark
suite. In 2012 IEEE conference on computer vision
and pattern recognition, pages 3354–3361. IEEE.
Gu, S., Zhang, Y., Tang, J., Yang, J., and Kong, H. (2019).
Road detection through crf based lidar-camera fusion.
In 2019 International Conference on Robotics and Au-
tomation (ICRA), pages 3832–3838. IEEE.
Han, L., Gao, F., Zhou, B., and Shen, S. (2019). Fiesta:
Fast incremental euclidean distance fields for online
motion planning of aerial robots. In 2019 IEEE/RSJ
Int. Conf. on Intelligent Robots and Systems (IROS),
pages 4423–4430. IEEE.
He, K., Zhang, X., Ren, S., and Sun, J. (2016). Deep resid-
ual learning for image recognition. In Proceedings of
the IEEE conference on computer vision and pattern
recognition, pages 770–778.
Hornung, A., Wurm, K. M., Bennewitz, M., Stachniss, C.,
and Burgard, W. (2013). Octomap: An efficient prob-
abilistic 3D mapping framework based on octrees. Au-
tonomous robots, 34:189–206.
Jiang, P., Osteen, P., Wigness, M., and Saripalli, S. (2021).
Rellis-3D dataset: Data, benchmarks and analysis.
In 2021 IEEE Int. Conf. on robotics and automation
(ICRA), pages 1110–1116. IEEE.
Jiang, P. and Saripalli, S. (2021). Lidarnet: A boundary-
aware domain adaptation model for point cloud se-
mantic segmentation. In 2021 IEEE Int. Conf. on
Robotics and Automation (ICRA), pages 2457–2464.
IEEE.
Kragh, M., Jørgensen, R. N., and Pedersen, H. (2015). Ob-
ject detection and terrain classification in agricultural
fields using 3D lidar data. In Computer Vision Sys-
tems: 10th Int. Conf., ICVS 2015, Copenhagen, Den-
mark, July 6-9, 2015, Proceedings, pages 188–197.
Springer.
Langer, D., Rosenblatt, J., and Hebert, M. (1994). A
behavior-based system for off-road navigation. IEEE
Transactions on Robotics and Automation, 10(6):776–
783.
Martinez, J. L., Moran, M., Morales, J., Robles, A., and
Sanchez, M. (2020). Supervised learning of natural-
terrain traversability with synthetic 3D laser scans.
Applied Sciences, 10(3):1140.
Moravec, H. and Elfes, A. (1985). High resolution maps
from wide angle sonar. In Proceedings. 1985 IEEE
Int. Conf. on robotics and automation, volume 2,
pages 116–121. IEEE.
Oleynikova, H., Taylor, Z., Fehr, M., Siegwart, R., and Ni-
eto, J. (2017). Voxblox: Incremental 3D euclidean
signed distance fields for on-board mav planning. In
2017 IEEE/RSJ Int. Conf. on Intelligent Robots and
Systems (IROS), pages 1366–1373. IEEE.
Qi, C. R., Su, H., Mo, K., and Guibas, L. J. (2017). Point-
net: Deep learning on point sets for 3D classification
and segmentation. In Proceedings of the IEEE con-
ference on computer vision and pattern recognition,
pages 652–660.
Razani, R., Cheng, R., Taghavi, E., and Bingbing, L.
(2021). Lite-hdseg: Lidar semantic segmentation us-
ing lite harmonic dense convolutions. In 2021 IEEE
Int. Conf. on Robotics and Automation (ICRA), pages
9550–9556. IEEE.
Redmon, J. and Farhadi, A. (2018). Yolov3: An incremental
improvement. arXiv preprint arXiv:1804.02767.
Ronneberger, O., Fischer, P., and Brox, T. (2015). U-
net: Convolutional networks for biomedical image
segmentation. In Medical Image Computing and
Computer-Assisted Intervention–MICCAI 2015: 18th
Int. Conf., Munich, Germany, October 5-9, 2015, Pro-
ceedings, Part III 18, pages 234–241. Springer.
Vapnik, V. (1999). The nature of statistical learning theory.
Springer science & business media.
ICINCO 2023 - 20th International Conference on Informatics in Control, Automation and Robotics
392