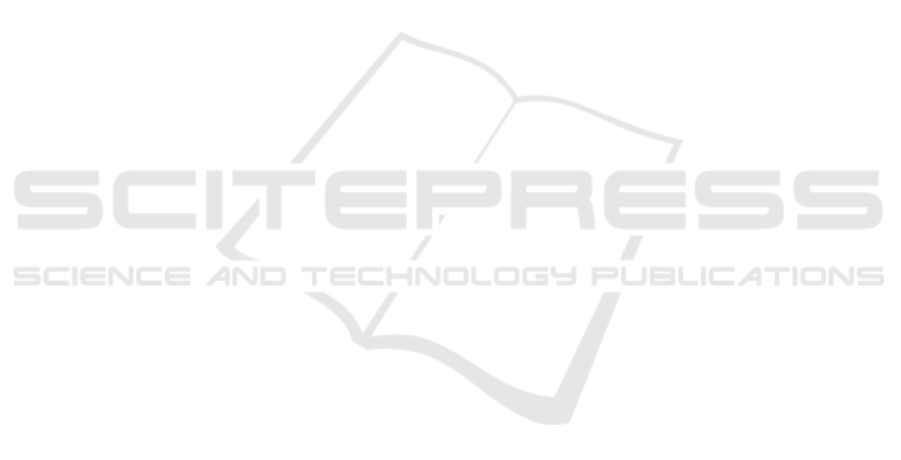
REFERENCES
Atas¸lar-Ayyıldız, B. (2023). Robust Trajectory Tracking
Control for Serial Robotic Manipulators Using Frac-
tional Order-Based PTID Controller. Fractal and
Fractional, 7(3):250.
Bellman, R. (1952). On the Theory of Dynamic Program-
ming. Proceedings of the National Academy of Sci-
ences, 38(8):716–719.
Bellman, R. (1958). Dynamic programming and stochastic
control processes. Information and Control, 1(3):228–
239.
Chua, K., Calandra, R., McAllister, R., and Levine, S.
(2018). Deep Reinforcement Learning in a Handful of
Trials using Probabilistic Dynamics Models. In Proc.
Adv. Neural Inform. Process. Syst., volume 31, pages
4754–4765.
de Boer, P.-T., Kroese, D. P., Mannor, S., and Rubin-
stein, R. Y. (2005). A Tutorial on the Cross-Entropy
Method. Annals of Operations Research, 134:19–67.
Duan, J., Gan, Y., Chen, M., and Dai, X. (2018). Adap-
tive variable impedance control for dynamic contact
force tracking in uncertain environment. Robot. Au-
ton. Syst., 102:54–65.
Formenti, A., Bucca, G., Shahid, A. A., Piga, D., and
Roveda, L. (2022). Improved impedance/admittance
switching controller for the interaction with a variable
stiffness environment. Complex Eng. Syst., 2(3):12.
Fujimoto, S., van Hoof, H., and Meger, D. (2018). Address-
ing Function Approximation Error in Actor-Critic
Methods. arXiv preprint.
Haarnoja, T., Zhou, A., Abbeel, P., and Levine, S.
(2018). Soft Actor-Critic: Off-Policy Maximum En-
tropy Deep Reinforcement Learning with a Stochastic
Actor. arXiv preprint.
Hogan, N. (1984). Impedance Control: An Approach to
Manipulation. In Proc. Amer. Contrl. Conf., pages
304–313, San Diego, CA, USA.
Iskandar, M., Ott, C., Albu-Sch
¨
affer, A., Siciliano, B., and
Dietrich, A. (2023). Hybrid Force-Impedance Control
for Fast End-Effector Motions. IEEE Robotics and
Automation Letters, 8(7):3931–3938.
Johannink, T., Bahl, S., Nair, A., Luo, J., Kumar, A.,
Loskyll, M., Ojea, J. A., Solowjow, E., and Levine, S.
(2019). Residual Reinforcement Learning for Robot
Control. In Proc. IEEE Int. Conf. Robot. Autom.,
pages 6023–6029, Montreal, QC, Canada.
Jung, S., Hsia, T. C. S., and Bonitz, R. G. (2004). Force
Tracking Impedance Control of Robot Manipulators
Under Unknown Environment. IEEE Trans. Control
Syst. Technol., 12(3):474–483.
Kasruddin Nasir, A. N., Ahmad, M. A., and Tokhi, M. O.
(2021). Hybrid spiral-bacterial foraging algorithm for
a fuzzy control design of a flexible manipulator. Jour-
nal of Low Frequency Noise, Vibration and Active
Control, 41(1):340–358.
Khatib, O. (1987). A unified approach for motion and force
control of robot manipulators: The operational space
formulation. IEEE J. Robot. Autom., 3(1):43–53.
Lee, K.-k. and Buss, M. (2008). Force Tracking Impedance
Control with Variable Target Stiffness. IFAC Proc.
Vol., 41(2):6751–6756.
Li, K., He, Y., Li, K., and Liu, C. (2023). Adaptive
fractional-order admittance control for force tracking
in highly dynamic unknown environments. Industrial
Robot: the international journal of robotics research
and application.
Liang, X., Zhao, H., Li, X., and Ding, H. (2018). Force
tracking impedance control with unknown environ-
ment via an iterative learning algorithm. In Proc.
IEEE 3rd Int. Conf. Adv. Robot. Mechatron., pages
158–164, Singapore, Singapore.
Lillicrap, T. P., Hunt, J. J., Pritzel, A., Heess, N., Erez, T.,
Tassa, Y., Silver, D., and Wierstra, D. (2015). Contin-
uous control with deep reinforcement learning. arXiv
preprint.
Liu, Y., Jiang, D., Yun, J., Sun, Y., Li, C., Jiang, G., Kong,
J., Tao, B., and Fang, Z. (2022). Self-Tuning Con-
trol of Manipulator Positioning Based on Fuzzy PID
and PSO Algorithm. Frontiers in Bioengineering and
Biotechnology, 9.
Mason, M. T. (1981). Compliance and Force Control for
Computer Controlled Manipulators. IEEE Trans. Syst.
Man Cybern.: Syst., 11(6):418–432.
Mohd Hanif, M. I. F., Ahmad, M. A., and Jui, J. J. (2021).
PID Tuning Method Using Chaotic Safe Experimen-
tation Dynamics Algorithm for Elastic Joint Manip-
ulator. Journal Europ
´
een des Syst
`
emes Automatis
´
es,
54(5):693–698.
Nagabandi, A., Kahn, G., Fearing, R. S., and Levine, S.
(2018). Neural Network Dynamics for Model-Based
Deep Reinforcement Learning with Model-Free Fine-
Tuning. In Proc. IEEE Int. Conf. Robot. Autom., Bris-
bane, QLD, Australia.
Obadina, O. O., Thaha, M. A., Mohamed, Z., and Sha-
heed, M. H. (2022). Grey-box modelling and fuzzy
logic control of a Leader–Follower robot manipulator
system: A hybrid Grey Wolf–Whale Optimisation ap-
proach. ISA Transactions, 129:572–593.
Paszke, A., Gross, S., Massa, F., Lerer, A., Bradbury, J.,
Chanan, G., Killeen, T., Lin, Z., Gimelshein, N.,
Antiga, L., Desmaison, A., K
¨
opf, A., Yang, E., De-
Vito, Z., Raison, M., Tejani, A., Chilamkurthy, S.,
Steiner, B., Fang, L., Bai, J., and Chintala, S. (2019).
PyTorch: An Imperative Style, High-Performance
Deep Learning Library. In Proc. Adv. Neural Inform.
Process. Syst., Red Hook, NY, USA. Curran Asso-
ciates Inc.
Peng, G., Philip Chen, C. L., He, W., and Yang, C. (2021).
Neural-Learning-Based Force Sensorless Admittance
Control for Robots With Input Deadzone. IEEE Trans.
Ind. Electron., 68(6):5184–5196.
Preitl, Z., Precup, R.-E., Tar, J. K., and Tak
´
acs, M. (2006).
Use of multi-parametric quadratic programming in
fuzzy control systems. Acta Polytechnica Hungarica,
3(3):29–43.
Puricelli, L., Pozzi, A., Petrone, V., Ferrentino, E., Chi-
acchio, P., Braghin, F., and Roveda, L. (2023). Op-
timized Residual Action for Interaction Control with
Learned Environments. TechRxiv Preprint.
Experimental Validation of an Actor-Critic Model Predictive Force Controller for Robot-Environment Interaction Tasks
403