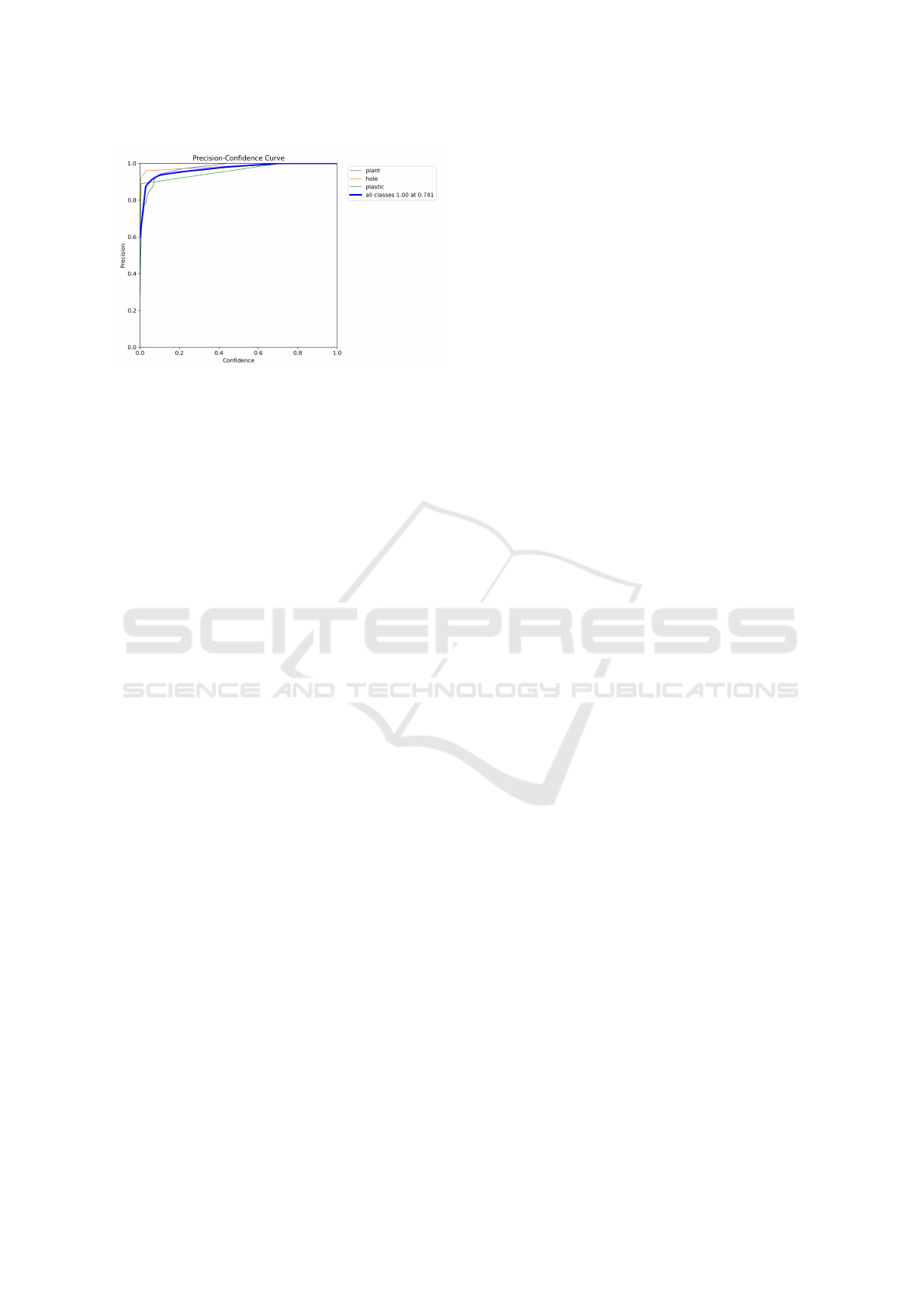
Figure 6: Performance evaluation of the model through Pre-
cision curve curve.
ACKNOWLEDGEMENTS
This publication is based upon work supported by the
Khalifa University of Science and Technology under
Award No. CIRA-2021-085, FSU-2021-019, RC1-
2018-KUCARS.
REFERENCES
Akram, W., Casavola, A., Kapetanovi
´
c, N., and Mi
ˇ
skovic,
N. (2022). A visual servoing scheme for autonomous
aquaculture net pens inspection using rov. Sensors,
22(9):3525.
Bochkovskiy, A., Wang, C.-Y., and Liao, H.-Y. M. (2020).
YOLOv4: Optimal Speed and Accuracy of Object De-
tection. arXiv, 2020.
Girshick, R. et al. (2015). Fast R-CNN. ICCV, 2015.
Gui, F., Zhu, H., and Feng, D. (2019). Research progress
on hydrodynamic characteristics of marine aquacul-
ture netting. Fish. Mod, 46:9–14.
He, K., Gkioxari, G., Dollar, P., and Girshick, R. Mask
R-CNN. IEEE International Conference on Computer
Vision (ICCV), 2017.
Jocher, G., Chaurasia, A., and Qiu, J. (2023). Yolo by ultr-
alytics.
Jocher, G., Chaurasia, A., Stoken, A., Borovec, J.,
NanoCode012, Kwon, Y., Michael, K., TaoXie, Fang,
J., imyhxy, Lorna, Yifu, Z., Wong, C., V, A., Montes,
D., Wang, Z., Fati, C., Nadar, J., Laughing, UnglvK-
itDe, Sonck, V., tkianai, yxNONG, Skalski, P., Hogan,
A., Nair, D., Strobel, M., and Jain, M. (2022). ultralyt-
ics/yolov5: v7.0 - YOLOv5 SOTA Realtime Instance
Segmentation.
Liao, W., Zhang, S., Wu, Y., An, D., and Wei, Y. (2022). Re-
search on intelligent damage detection of far-sea cage
based on machine vision and deep learning. Aquacul-
tural Engineering, 96:102219.
Liu, W., Anguelov, D., Erhan, D., Szegedy, C., Reed, S.,
Fu, C.-Y., and Berg, A. C. (2016). SSD: Single Shot
MultiBox Detector. ECCV, 2016.
Madshaven, A., Schellewald, C., and Stahl, A. (2022). Hole
detection in aquaculture net cages from video footage.
In Fourteenth International Conference on Machine
Vision (ICMV 2021), volume 12084, pages 258–267.
SPIE.
Qiu, W., Pakrashi, V., and Ghosh, B. (2020). Fishing
net health state estimation using underwater imaging.
Journal of Marine Science and Engineering, 8(9):707.
Roboflow (2022). Net Defect Detection Dataset-v1. Re-
trieved: December 14th, 2022.
Sun, M., Yang, X., and Xie, Y. (2020). Deep learning in
aquaculture: A review. J. Comput, 31(1):294–319.
Tao, Q., Huang, K., Qin, C., Guo, B., Lam, R., and
Zhang, F. (2018). Omnidirectional surface vehicle for
fish cage inspection. In OCEANS 2018 MTS/IEEE
Charleston, pages 1–6. IEEE.
Wang, C.-Y., Bochkovskiy, A., and Liao, H.-Y. M. (2023).
Yolov7: Trainable bag-of-freebies sets new state-of-
the-art for real-time object detectors. In Proceedings
of the IEEE/CVF Conference on Computer Vision and
Pattern Recognition, pages 7464–7475.
Wei, Y., Wei, Q., and An, D. (2020). Intelligent monitor-
ing and control technologies of open sea cage culture:
A review. Computers and electronics in agriculture,
169:105119.
Yan, G., Ni, X., and Mo, J. (2018). Research status and
development tendency of deep sea aquaculture equip-
ments: a review. Journal of Dalian Ocean University,
33(1):123–129.
Yang, X., Zhang, S., Liu, J., Gao, Q., Dong, S., and Zhou,
C. (2021). Deep learning for smart fish farming: ap-
plications, opportunities and challenges. Reviews in
Aquaculture, 13(1):66–90.
Zhang, Z., Gui, F., Qu, X., and Feng, D. (2022). Net-
ting damage detection for marine aquaculture facili-
ties based on improved mask r-cnn. Journal of Marine
Science and Engineering, 10(7):996.
Zhou, W., Shi, J., Yu, W., Liu, F., Song, W., Gui, F., and
Yang, F. (2018). Current situation and development
trend of marine seine culture in china. Fish. Inf. Strat-
egy, 33:259–266.
Evaluating Deep Learning Assisted Automated Aquaculture Net Pens Inspection Using ROV
591