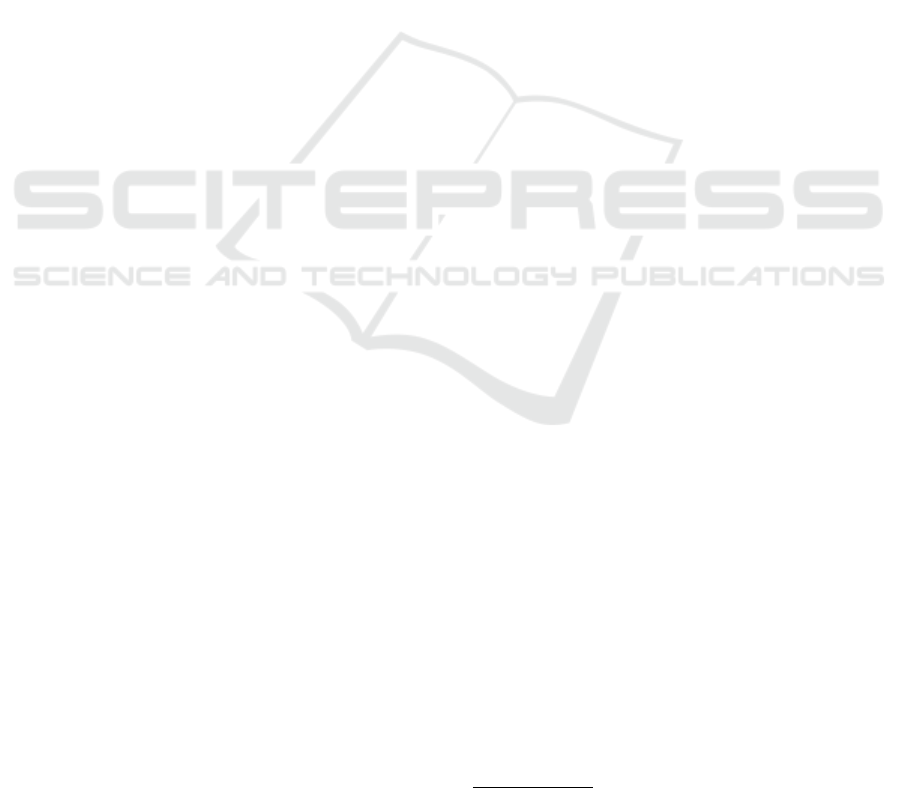
SIGDAT Conference on Empirical Methods in Natural
Language Processing, EMNLP ’14, pages 740–750.
ACL.
Churchill, R. and Singh, L. (2022). The evolution of topic
modeling. ACM Computing Surveys, 54(10):215:1–
35.
Churchill, R., Singh, L., and Kirov, C. (2018). A temporal
topic model for noisy mediums. In Proc. 22nd Pacific-
Asia Conference on Knowledge Discovery and Data
Mining, PAKDD ’18, pages 42–53. Springer.
Clark, K. and Manning, C. D. (2016). Deep reinforce-
ment learning for mention-ranking coreference mod-
els. In Proc. SIGDAT Conference on Empirical Meth-
ods on Natural Language Processing, EMNLP ’16,
pages 2256–2262. ACL.
de Rivero, M., Tirado, C., and Ugarte, W. (2021). For-
malStyler: GPT based model for formal style transfer
based on formality and meaning preservation. In Proc.
13th International Conference on Knowledge Discov-
ery and Information Retrieval, KDIR ’21, pages 48–
56. SciTePress.
Finkel, J. R., Grenager, T., and Manning, C. D. (2005).
Incorporating non-local information into information
extraction systems by Gibbs sampling. In Proc. 43rd
Annual Meeting of the Association for Computational
Linguistics, ACL ’05, pages 363–370. ACL.
Floridi, L. and Chiriatti, M. (2020). GPT-3: Its nature,
scope, limits, and consequences. Springer Minds and
Machines, 30:681–694.
Gehrmann, S., Strobelt, H., and Rush, A. M. (2019). GLTR:
Statistical detection and visualization of generated
text. In Proc. 57th Annual Meeting of the Associa-
tion for Computational Linguistics: System Demon-
strations, ACL ’19, pages 111–116. ACL.
Houvardas, J. and Stamatatos, E. (2006). N-gram feature
selection for authorship identification. In Proc. 12th
International Conference on Artificial Intelligence:
Methodols, Systems, and Applications, AIMSA ’06,
pages 77–86. Springer.
Ippolito, D., Duckworth, D., Callison-Burch, C., and Eck,
D. (2019). Automatic detection of generated text is
easiest when humans are fooled. In Proc. 58th An-
nual Meeting of the Association for Computational
Linguistics, ACL ’19, pages 1808–1822. Association
for Computational Linguistics.
Jan Hendrik Kirchner, Lama Ahmad, S. A. . J. L. (2023).
OpenAI AI classifier. https://openai.com/blog/ne
w-ai-classifier-for-indicating-ai-written-text. last
Accessed: 2023-03.
Lund, B. D. and Wang, T. (2023). Chatting about ChatGPT:
how may AI and GPT impact academia and libraries?
Library Hi Tech News, 40(3):26–29.
Mohiuddin, T., Joty, S., and Nguyen, D. T. (2018). Coher-
ence modeling of asynchronous conversations: A neu-
ral entity grid approach. In Proc. 56th Annual Meet-
ing of the Association for Computational Linguistics
– Volume 1: Long Papers, ACL ’18, pages 558–568.
ACL.
Nachar, N. (2008). The Mann-Whitney U: A test for assess-
ing whether two independent samples come from the
same distribution. Tutorials in Quantitative Methods
for Psychology, 4(1):13–20.
OpenAI (2023). GPT-4 technical report. arXiv CoRR,
cs.CL. arXiv:2303.08774v3.
Posadas-Dur
´
an, J.-P., Sidorov, G., G
´
omez-Adorno, H.,
Batyrshin, I., Mirasol-M
´
elendez, E., Posadas-Dur
´
an,
G., and Chanona-Hern
´
andez, L. (2017). Algorithm for
extraction of subtrees of a sentence dependency parse
tree. Acta Polytechnica Hungarica, 14(3):79–98.
Radford, A., Wu, J., Child, R., Luan, D., Amodei, D.,
Sutskever, I., et al. (2019). Language models are un-
supervised multitask learners. Technical report, Ope-
nAI.
R
´
ıos-Toledo, G., Posadas-Dur
´
an, J. P. F., Sidorov, G., and
Castro-S
´
anchez, N. A. (2022). Detection of changes
in literary writing style using n-grams as style mark-
ers and supervised machine learning. PLOS ONE,
17(7):1–24.
Rudin, C. (2019). Stop explaining black box machine learn-
ing models for high stakes decisions and use inter-
pretable models instead. Nature Machine Intelligence,
1(5):206–215.
Shu, K., Sliva, A., Wang, S., Tang, J., and Liu, H. (2017).
Fake news detection on social media: A data mining
perspective. ACM SIGKDD Explorations Newsletter,
19(1):22–36.
Zellers, R., Holtzman, A., Rashkin, H., Bisk, Y., Farhadi,
A., Roesner, F., and Choi, Y. (2019). Defending
against neural fake news. NeurIPS ’19. Curran As-
sociates, Inc.
Zhang, C. and Wang, J. (2022). Tag-Set-Sequence learn-
ing for generating question-answer pairs. In Proc.
14th International Conference on Knowledge Discov-
ery and Information Retrieval, KDIR ’22, pages 138–
147. SciTePress.
Zhang, X. and Ghorbani, A. A. (2020). An overview of on-
line fake news: Characterization, detection, and dis-
cussion. Elsevier Information Processing & Manage-
ment, 57(2):102025:1–26.
APPENDIX
Expert Study Design
The expert study with 13 participants was conducted
using Google Forms
9
to collect the given answers and
guide the participants through the process. The partic-
ipants were observed by an instructor in person during
the time of the study to capture direct comments on
the components. Before the study, participants were
told that the efficiency of the tool was to be evaluated
and the background in explainable AI was not men-
tioned explicitly. During the session, instructors min-
imized their communication with the participants, ex-
9
https://docs.google.com/forms/d/1-
dLyWXKx01stPUcldRJ35dovfQmpuRyxcdtIqSj6bjk/prefill
UNCOVER: Identifying AI Generated News Articles by Linguistic Analysis and Visualization
49