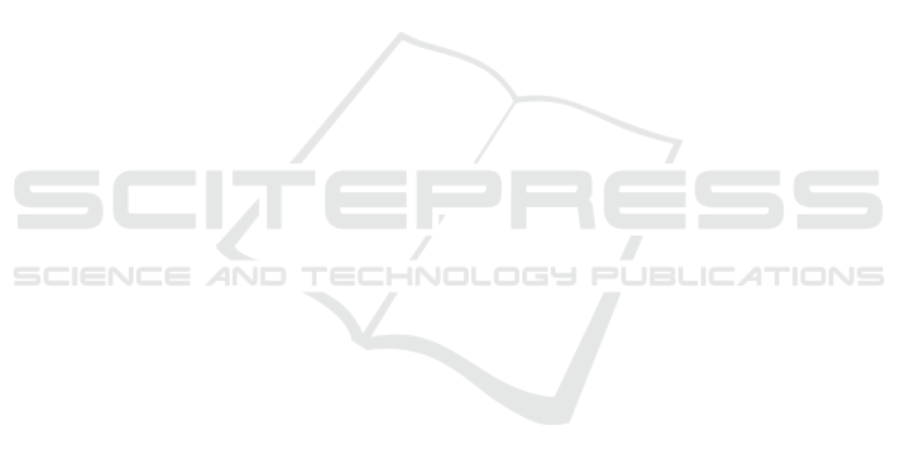
paradigms and data structures, volume 1611, pages
586–606. Spie.
Breiman, L. (2001). Random forests. Machine learning,
45:5–32.
Chabanet, S., Bril El-Haouzi, H., Morin, M., Gaudreault,
J., and Thomas, P. (2023). Toward digital twins for
sawmill production planning and control: benefits, op-
portunities, and challenges. International Journal of
Production Research, 61(7):2190–2213.
Chabanet, S., Chazelle, V., Thomas, P., and El-Haouzi,
H. B. (2021a). Dissimilarity to class medoids as fea-
tures for 3d point cloud classification. In Advances
in Production Management Systems. Artificial Intelli-
gence for Sustainable and Resilient Production Sys-
tems: IFIP WG 5.7 International Conference, APMS
2021, Nantes, France, September 5–9, 2021, Proceed-
ings, Part III, pages 573–581. Springer.
Chabanet, S., Thomas, P., and El-Haouzi, H. B. (2021b).
Medoid-based mlp: an application to wood sawing
simulator metamodeling. In 13th International Con-
ference on Neural Computation Theory and Applica-
tions, NCTA 2021.
Duin, R. and P˛ekalska, E. (2009). The dissimilarity repre-
sentation for pattern recognition: a tutorial. Technical
Report.
Dyer, M. and Frieze, A. (1985). A simple heuristic for
the p-centre problem. Operations Research Letters,
3(6):285–288.
Goulet, P. (2006). Optitek: User’s manual.
Jain, B. J. and Spiegel, S. (2015). Time series classification
in dissimilarity spaces. In AALTD@ PKDD/ECML.
Kar, P. and Jain, P. (2011). Similarity-based learning via
data driven embeddings. Advances in neural informa-
tion processing systems, 24.
Livi, L., Rizzi, A., and Sadeghian, A. (2014). Optimized
dissimilarity space embedding for labeled graphs. In-
formation Sciences, 266:47–64.
Luo, C., Li, Y., and Chung, S. M. (2009). Text document
clustering based on neighbors. Data & Knowledge
Engineering, 68(11):1271–1288.
Maranzana, F. E. (1963). On the location of supply points to
minimize transportation costs. IBM Systems Journal,
2(2):129–135.
Martineau, V., Morin, M., Gaudreault, J., Thomas, P., and
El-Haouzi, H. B. (2021). Neural network architectures
and feature extraction for lumber production predic-
tion. In The 34th Canadian Conference on Artificial
Intelligence.
Morin, M., Gaudreault, J., Brotherton, E., Paradis, F., Rol-
land, A., Wery, J., and Laviolette, F. (2020). Machine
learning-based models of sawmills for better wood al-
location planning. International Journal of Produc-
tion Economics, 222:107508.
Morin, M., Paradis, F., Rolland, A., Wery, J., Laviolette,
F., and Laviolette, F. (2015). Machine learning-based
metamodels for sawing simulation. In 2015 Win-
ter Simulation Conference (WSC), pages 2160–2171.
IEEE.
Morneau-Pereira, M., Arabi, M., Gaudreault, J., Nourelfath,
M., and Ouhimmou, M. (2014). An optimization and
simulation framework for integrated tactical planning
of wood harvesting operations, wood allocation and
lumber production. In MOSIM 2014, 10eme Con-
férence Francophone de Modélisation, Optimisation
et Simulation.
Müller, M. (2007). Dynamic time warping. Information
retrieval for music and motion, pages 69–84.
Munoz, A. and de Diego, I. M. n. (2006). From indefinite
to positive semi-definite matrices. In Structural, Syn-
tactic, and Statistical Pattern Recognition: Joint IAPR
International Workshops, SSPR 2006 and SPR 2006,
Hong Kong, China, August 17-19, 2006. Proceedings,
pages 764–772. Springer.
Ong, C. S., Mary, X., Canu, S., and Smola, A. J. (2004).
Learning with non-positive kernels. In Proceedings of
the twenty-first international conference on Machine
learning, page 81.
P˛ekalska, E., Duin, R. P., and Paclík, P. (2006). Prototype
selection for dissimilarity-based classifiers. Pattern
Recognition, 39(2):189–208.
Qi, C. R., Su, H., Mo, K., and Guibas, L. J. (2017). Point-
net: Deep learning on point sets for 3d classification
and segmentation. In Proceedings of the IEEE con-
ference on computer vision and pattern recognition,
pages 652–660.
Sarle, W. S. (1991). Finding groups in data: An introduction
to cluster analysis.
Schleif, F.-M. and Tino, P. (2015). Indefinite prox-
imity learning: A review. Neural Computation,
27(10):2039–2096.
Schubert, E. and Rousseeuw, P. J. (2021). Fast and eager
k-medoids clustering: O (k) runtime improvement of
the pam, clara, and clarans algorithms. Information
Systems, 101:101804.
Selma, C., Bril El Haouzi, H., Thomas, P., Gaudreault,
J., and Morin, M. (2018). An iterative closest point
method for measuring the level of similarity of 3d
log scans in wood industry. Service Orientation in
Holonic and Multi-Agent Manufacturing: Proceed-
ings of SOHOMA 2017, pages 433–444.
Wery, J., Gaudreault, J., Thomas, A., and Marier, P. (2018).
Simulation-optimisation based framework for sales
and operations planning taking into account new prod-
ucts opportunities in a co-production context. Com-
puters in industry, 94:41–51.
NCTA 2023 - 15th International Conference on Neural Computation Theory and Applications
486