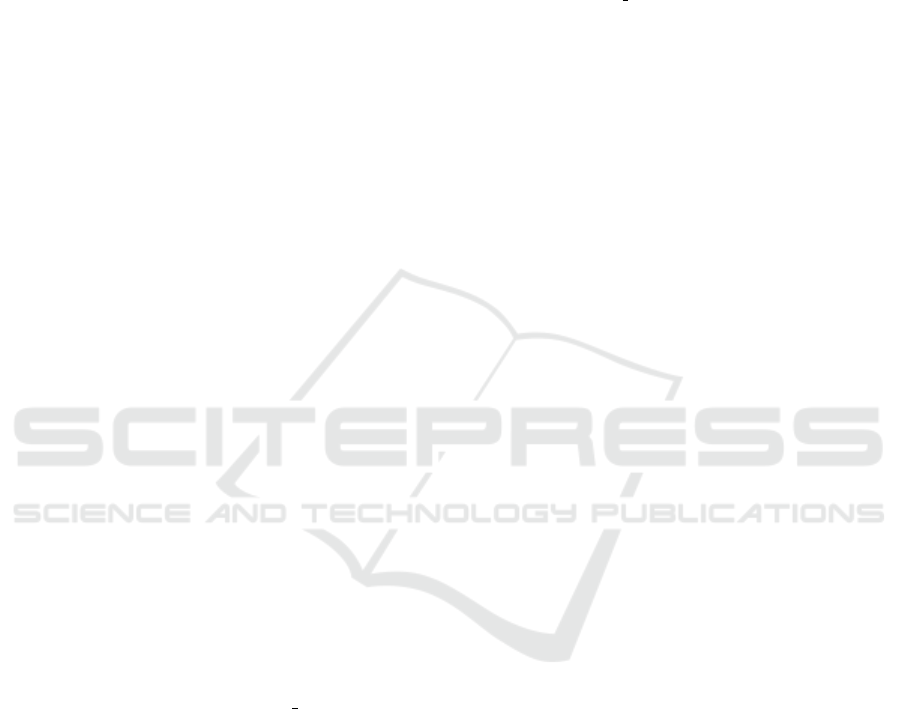
ACKNOWLEDGEMENTS
The research reported in this paper has been sup-
ported by the German Research Foundation (DFG)
funded projects NFDI2/12020 for AB and NK, and
467401796 for MH, and by the Grant Agency of
the Czech Technical University in Prague, grant No.
SGS20/208/OHK3/3T/18 for LK.
Computational resources were provided by the e-
INFRA CZ project (ID:90254), supported by the Min-
istry of Education, Youth and Sports of the Czech
Republic and by the ELIXIR-CZ project (ID:90255),
part of the international ELIXIR infrastructure.
REFERENCES
Chen, W., Zhang, M., and Qin, B. (2011). Coreference
resolution system using maximum entropy classifier.
In Proceedings of the Fifteenth Conference on Com-
putational Natural Language Learning: Shared Task,
CONLL Shared Task ’11, page 127–130, USA. Asso-
ciation for Computational Linguistics.
Damodaran, P. (2021). Parrot: Paraphrase generation for
nlu. 2023-02-11.
Devlin, J., Chang, M.-W., Lee, K., and Toutanova, K.
(2019). BERT: Pre-training of deep bidirectional
transformers for language understanding. In Pro-
ceedings of the 2019 Conference of the North Amer-
ican Chapter of the Association for Computational
Linguistics: Human Language Technologies, Volume
1 (Long and Short Papers), pages 4171–4186, Min-
neapolis, Minnesota. Association for Computational
Linguistics.
Garanina, N. O., Sidorova, E. A., and Seryi, A. S. (2018).
Multiagent approach to coreference resolution based
on the multifactor similarity in ontology population.
Programming and Computer Software, 44(1):23–34.
Huggingface (2019a). Bart paraphrase model (large). 2023-
02-11.
Huggingface (2019b). tuner007/pegasus paraphrase. 2023-
02-11.
Lee, K., He, L., and Zettlemoyer, L. (2018). Higher-order
coreference resolution with coarse-to-fine inference.
In Proceedings of the 2018 Conference of the North
American Chapter of the Association for Computa-
tional Linguistics: Human Language Technologies,
Volume 2 (Short Papers), pages 687–692, New Or-
leans, Louisiana. Association for Computational Lin-
guistics.
Lewis, M., Liu, Y., Goyal, N., Ghazvininejad, M., Mo-
hamed, A., Levy, O., Stoyanov, V., and Zettlemoyer,
L. (2019). Bart: Denoising sequence-to-sequence pre-
training for natural language generation, translation,
and comprehension.
Lu, K., Grover, A., Abbeel, P., and Mordatch, I. (2021).
Pretrained transformers as universal computation en-
gines. CoRR, abs/2103.05247.
Nov
´
ak, M. (2017). Coreference resolution system not only
for czech. In Proceedings of the 17th conference ITAT
2017: Slovensko
ˇ
cesk
´
y NLP workshop (SloNLP 2017),
pages 193–200, Praha, Czechia. CreateSpace Inde-
pendent Publishing Platform.
Ramsrigouthamg, H. (2022a). Ramsrigouthamg/t5-large-
paraphraser-diverse-high-quality. 2023-02-11.
Ramsrigouthamg, H. (2022b). Ram-
srigouthamg/t5 paraphraser. 2023-02-11.
Reimers, N. and Gurevych, I. (2019). Sentence-bert: Sen-
tence embeddings using siamese bert-networks. In
Proceedings of the 2019 Conference on Empirical
Methods in Natural Language Processing. Associa-
tion for Computational Linguistics.
Salim, M. N. and Mustafa, B. S. (2021). Uttokb: a model for
semantic relation extraction from unstructured text.
In 2021 5th International Symposium on Multidis-
ciplinary Studies and Innovative Technologies (ISM-
SIT), pages 591–595.
Thakur, N., Reimers, N., Daxenberger, J., and Gurevych,
I. (2021). Augmented SBERT: Data augmentation
method for improving bi-encoders for pairwise sen-
tence scoring tasks. In Proceedings of the 2021 Con-
ference of the North American Chapter of the Associa-
tion for Computational Linguistics: Human Language
Technologies, pages 296–310, Online. Association for
Computational Linguistics.
Trieu, H.-L., Duong Nguyen, A.-K., Nguyen, N., Miwa,
M., Takamura, H., and Ananiadou, S. (2019). Coref-
erence resolution in full text articles with BERT and
syntax-based mention filtering. In Proceedings of the
5th Workshop on BioNLP Open Shared Tasks, pages
196–205, Hong Kong, China. Association for Com-
putational Linguistics.
Valurank, H. (2022). Valurank/t5-paraphraser. 2023-02-11.
Vamsi, H. (2019). Paraphrase-generation. 2023-02-11.
Vorobev, V. and Kuznetsov, M. (2023). A paraphrasing
model based on chatgpt paraphrases. In A paraphras-
ing model based on ChatGPT paraphrases.
Yang, Y., Zhang, Y., Tar, C., and Baldridge, J. (2019).
PAWS-X: A Cross-lingual Adversarial Dataset for
Paraphrase Identification. In Proc. of EMNLP.
Zhang, J., Zhao, Y., Saleh, M., and Liu, P. J. (2019a). Pe-
gasus: Pre-training with extracted gap-sentences for
abstractive summarization.
Zhang, Y., Baldridge, J., and He, L. (2019b). PAWS: Para-
phrase Adversaries from Word Scrambling. In Proc.
of NAACL.
Using Paraphrasers to Detect Duplicities in Ontologies
49