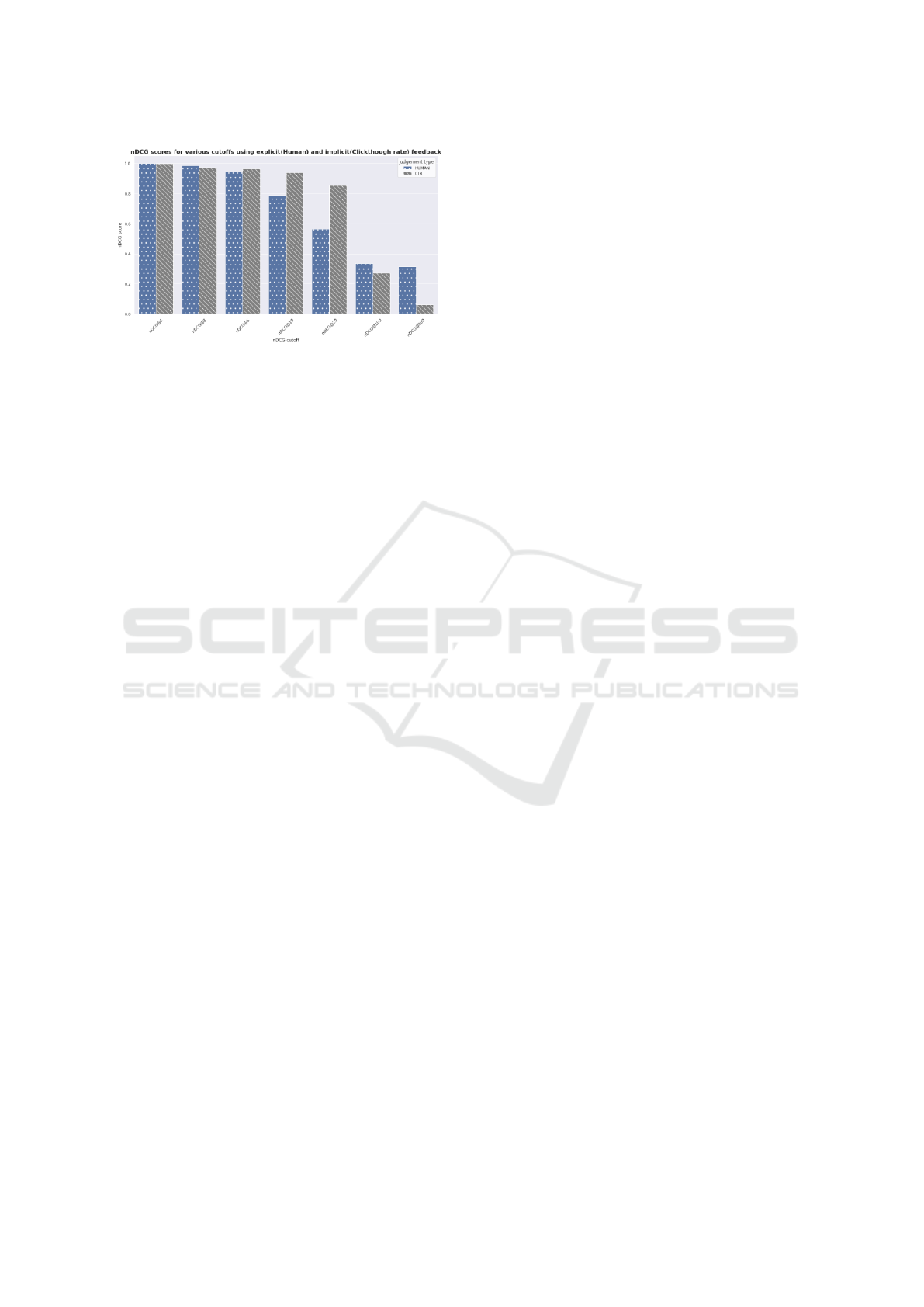
Figure 4: A bar chart showing the performance differences
between explicit and implicit feedback for various nDCG
cutoffs. For nDCG@3, the human relevance judgements are
just 1.6% higher than those recorded using click-through
feedback. For nDCG@5, nDCG@10 and nDCG@20, the
use of CTR as ground truth achieves higher scores than hu-
man judgements.
Future work may include mitigation of the identi-
fied bias in both approaches, e.g. by applying an in-
verse propensity score or introducing more diversity
to annotator selection.
Enterprise content is diverse and different for ev-
ery organisation. The generalisability of the ENTRP-
SRCH dataset is therefore limited. However, since
click-through feedback is cheap and abundant com-
pared to human relevance judgements, our (correla-
tion and ranking performance) findings for our organ-
isation may present a crucial cost-saving opportunity
to other organisations considering which type of feed-
back approach they should adopt for learning to rank
in the context of Enterprise Search.
ACKNOWLEDGEMENTS
This research was conducted with the financial sup-
port of Science Foundation Ireland under Grant
Agreement No. 13/RC/2106 P2 at the ADAPT SFI
Research Centre at Trinity College Dublin. ADAPT,
the SFI Research Centre for AI-Driven Digital Con-
tent Technology, is funded by Science Foundation Ire-
land through the SFI Research Centres Programme.
REFERENCES
Abrol, M., Latarche, N., Mahadevan, U., Mao, J., Mukher-
jee, R., Raghavan, P., Tourn, M., Wang, J., and Zhang,
G. (2001). Navigating Large-Scale Semi-Structured
Data in Business Portals. In International Conference
on Very Large Data Bases, VLDB ’01, page 663–666,
San Francisco, CA, USA. Morgan Kaufmann Publish-
ers Inc.
Ai, Q., Mao, J., Liu, Y., and Croft, W. B. (2018). Unbiased
Learning to Rank: Theory and Practice. In Proceed-
ings of the 2018 ACM SIGIR International Conference
on Theory of Information Retrieval, ICTIR ’18, page
1–2, New York, NY, USA. Association for Computing
Machinery.
Akoglu, H. (2018). User’s guide to correlation coefficients.
Turkish Journal of Emergency Medicine, 18(3):91.
Bentley, J. (2011). Mind the Enterprise Search Gap: Smart-
logic Sponsor MindMetre Research Report.
Białecki, A., Muir, R., Ingersoll, G., and Imagination, L.
(2012). Apache lucene 4. In SIGIR 2012 workshop on
open source information retrieval, page 17.
Chapelle, O. (2011). Yahoo! Learning to Rank Challenge
Overview.
Cleverley, P. H. and Burnett, S. (2019). Enterprise search
and discovery capability: The factors and generative
mechanisms for user satisfaction:. Journal of Infor-
mation Science, 45(1):29–52.
Craswell, N., Cambridge, M., and Soboroff, I. (2005).
Overview of the TREC-2005 Enterprise Track. In
TREC 2005 conference notebook, pages 199–205.
Dowsett, C. (2018). It’s Time to Talk About Organizational
Bias in Data Use.
Fagin, R., Kumar, R., McCurley, K. S., Novak, J., Sivaku-
mar, D., Tomlin, J. A., and Williamson, D. P. (2003).
Searching the workplace web. Proceedings of the 12th
International Conference on World Wide Web, WWW
2003, pages 366–375.
Hawking, D. (2004). Challenges in Enterprise Search. In
Proceedings of the 15th Australasian Database Con-
ference - Volume 27, ADC ’04, page 15–24, AUS.
Australian Computer Society, Inc.
Jawaheer, G., Szomszor, M., and Kostkova, P. (2010). Com-
parison of implicit and explicit feedback from an on-
line music recommendation service. Information Het-
erogeneity and Fusion in Recommender Systems, Het-
Rec 2010, pages 47–51.
Joachims, T. (2002). Optimizing search engines using click-
through data. In Proceedings of the ACM SIGKDD In-
ternational Conference on Knowledge Discovery and
Data Mining.
Kelly, D. and Teevan, J. (2003). Implicit feedback for infer-
ring user preference. ACM SIGIR Forum, 37(2):18–
28.
Kim, Y., Son, S. W., and Jeong, H. (2010). LinkRank:
Finding communities in directed networks. Physi-
cal Review E - Statistical, Nonlinear, and Soft Matter
Physics, 81(1).
Kruschwitz, U. and Hull, C. (2017). Searching the Enter-
prise. Foundations and Trends® in Information Re-
trieval, 11(1):1–142.
Li, H. (2011). A Short Introduction to Learning to Rank.
IEICE Transactions, 94-D:1854–1862.
Liu, T.-Y., Xu, J., Qin, T., Xiong, W., and Li, H. (2007).
LETOR: Benchmark Datasets for Learning to Rank.
SIGIR 2007 Workshop on Learning to Rank for Infor-
mation Retrieval, 1(Lr4ir):3–10.
Lykke, M., Bygholm, A., Søndergaard, L. B., and Bystr
¨
om,
K. (2021). The role of historical and contextual
KDIR 2023 - 15th International Conference on Knowledge Discovery and Information Retrieval
246