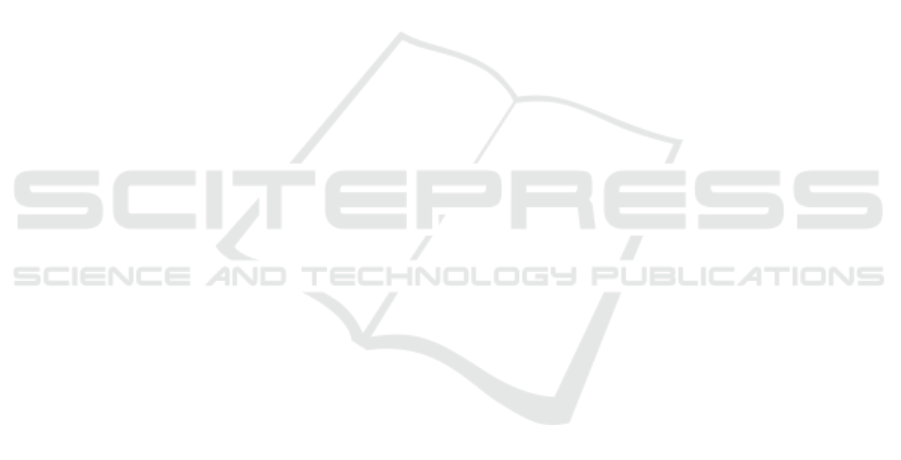
tion. IEEE Transactions on Evolutionary Computa-
tion, 19(5):630–643.
Huang, C., Li, Y., and Yao, X. (2019). A survey of
automatic parameter tuning methods for metaheuris-
tics. IEEE transactions on evolutionary computation,
24(2):201–216.
Jin, Y. (2005). A comprehensive survey of fitness approxi-
mation in evolutionary computation. Soft computing,
9(1):3–12.
Kajdanowicz, T., Plamowski, S., and Kazienko, P. (2011).
Training set selection using entropy based distance. In
2011 IEEE Jordan Conference on Applied Electrical
Engineering and Computing Technologies (AEECT),
pages 1–5. IEEE.
Kordos, M. and Blachnik, M. (2012). Instance selection
with neural networks for regression problems. In In-
ternational Conference on Artificial Neural Networks,
pages 263–270. Springer.
Kordos, M. and Łapa, K. (2018). Multi-objective evolution-
ary instance selection for regression tasks. Entropy,
20(10):746.
Koza, J. R. (1992). Genetic Programming: On the Pro-
gramming of Computers by Means of Natural Selec-
tion. MIT Press, Cambridge, MA, USA.
Kshirsagar, M., Gupt, K. K., Vaidya, G., Ryan, C., Sullivan,
J. P., and Kshirsagar, V. (2022). Insights into incor-
porating trustworthiness and ethics in ai systems with
explainable ai. International Journal of Natural Com-
puting Research (IJNCR), 11(1):1–23.
La Cava, W., Danai, K., Spector, L., Fleming, P., Wright,
A., and Lackner, M. (2016a). Automatic identification
of wind turbine models using evolutionary multiobjec-
tive optimization. Renewable Energy, 87:892–902.
La Cava, W., Spector, L., and Danai, K. (2016b). Epsilon-
lexicase selection for regression. In Proceedings of
the Genetic and Evolutionary Computation Confer-
ence 2016, pages 741–748.
Lobo, F. G. and Lima, C. F. (2007). Adaptive population
sizing schemes in genetic algorithms. Parameter set-
ting in evolutionary algorithms, 54:185–204.
Murphy, A., Youssef, A., Gupt, K. K., Raja, M. A., and
Ryan, C. (2021). Time is on the side of grammatical
evolution. In 2021 International Conference on Com-
puter Communication and Informatics (ICCCI), pages
1–7.
Nicolau, M. and Slattery, D. (2006). libGE. for version
0.27alpha1, 14 September 2006.
Olvera-L
´
opez, J. A., Carrasco-Ochoa, J. A., Mart
´
ınez-
Trinidad, J., and Kittler, J. (2010). A review of in-
stance selection methods. Artificial Intelligence Re-
view, 34(2):133–143.
O’Neill, M., Nicolau, M., and Agapitos, A. (2014). Exper-
iments in program synthesis with grammatical evo-
lution: A focus on integer sorting. In 2014 IEEE
Congress on Evolutionary Computation (CEC), pages
1504–1511. IEEE.
Ryan, C., Collins, J. J., and Neill, M. O. (1998). Gram-
matical evolution: Evolving programs for an arbitrary
language. In European Conference on Genetic Pro-
gramming, pages 83–96.
Ryan., C., Kshirsagar., M., Gupt., K. K., Rosenbauer.,
L., and Sullivan., J. (2021). Hierarchical Cluster-
ing Driven Test Case Selection in Digital Circuits.
In Proceedings of the 16th International Conference
on Software Technologies - ICSOFT, pages 589–596.
SciTePress.
Ryan, C., Tetteh, M. K., and Dias, D. M. (2020). Be-
havioural modelling of digital circuits in system ver-
ilog using grammatical evolution. In IJCCI, pages 28–
39.
Schmidt, M. D. and Lipson, H. (2007). Coevolving fitness
models for accelerating evolution and reducing evalu-
ations. In Genetic Programming Theory and Practice
IV, pages 113–130. Springer.
Slowik, A. and Kwasnicka, H. (2020). Evolutionary al-
gorithms and their applications to engineering prob-
lems. Neural Computing and Applications, 32:12363–
12379.
Son, S.-H. and Kim, J.-Y. (2006). Data reduction for
instance-based learning using entropy-based parti-
tioning. In International Conference on Computa-
tional Science and Its Applications, pages 590–599.
Springer.
Streichert, F., Ulmer, H., and Zell, A. (2005). Parallelization
of multi-objective evolutionary algorithms using clus-
tering algorithms. In Evolutionary Multi-Criterion
Optimization: Third International Conference, EMO
2005, Guanajuato, Mexico, March 9-11, 2005. Pro-
ceedings 3, pages 92–107. Springer.
Traiola, M., Virazel, A., Girard, P., Barbareschi, M., and
Bosio, A. (2018). Testing approximate digital cir-
cuits: Challenges and opportunities. In 2018 IEEE
19th Latin-American Test Symposium (LATS), pages
1–6. IEEE.
Youssef, A., Gupt, K. K., Raja, M. A., Murphy, A., and
Ryan, C. (2021). Evolutionary computing based anal-
ysis of diversity in grammatical evolution. In 2021 In-
ternational Conference on Artificial Intelligence and
Smart Systems (ICAIS), pages 1688–1693.
Zhang, H. and Zhou, A. (2021). Rl-gep: symbolic regres-
sion via gene expression programming and reinforce-
ment learning. In 2021 International Joint Conference
on Neural Networks (IJCNN), pages 1–8. IEEE.
Adaptive Case Selection for Symbolic Regression in Grammatical Evolution
205