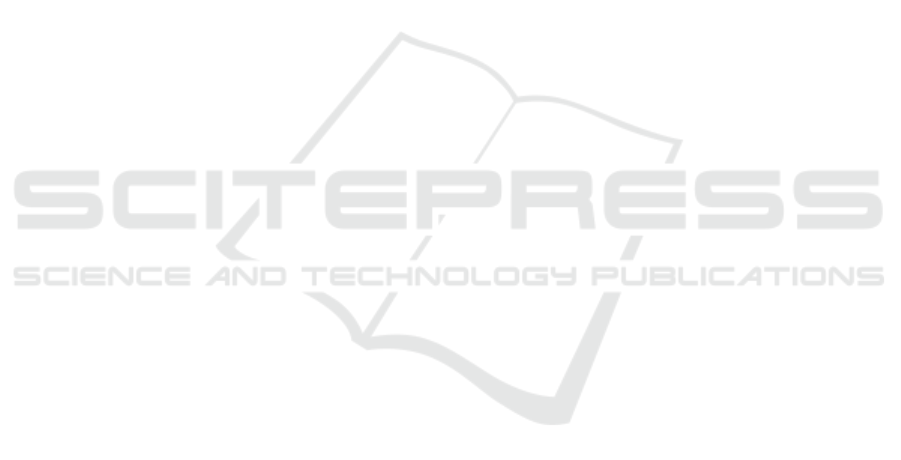
Denholm, P. and Hand, M. (2011). Grid flexibility and stor-
age required to achieve very high penetration of vari-
able renewable electricity. Energy Policy, 39(3):1817–
1830.
Derbel, B., Pruvost, G., Liefooghe, A., Verel, S., and
Zhang, Q. (2023). Walsh-based surrogate-assisted
multi-objective combinatorial optimization: A fine-
grained analysis for pseudo-boolean functions. Ap-
plied Soft Computing, 136:110061.
Drouet, V., Do, J.-M., and Verel, S. (2020a). Optimization
of load-follow operations of a 1300mw pressurized
water reactor using evolutionnary algorithms. In In-
ternational Conference on Physics of Reactors: Tran-
sition to a Scalable Nuclear Future (PHYSOR 2020),
volume 247, pages 1–8/11001.
Drouet, V., Verel, S., and Do, J.-M. (2020b). Surrogate-
assisted asynchronous multiobjective algorithm for
nuclear power plant operations. In Proceedings of the
2020 Genetic and Evolutionary Computation Confer-
ence, GECCO ’20, page 1073–1081, New York, NY,
USA. Association for Computing Machinery.
GENCI (2023). http://www.genci.fr/.
Grard, H. (2014). Physique, fonctionnement et s
ˆ
uret
´
e des
REP : Le r
´
eacteur en production. EDP Sciences.
Jenkins, J., Zhou, Z., Ponciroli, R., Vilim, R., Ganda, F., de
Sisternes, F., and Botterud, A. (2018). The benefits
of nuclear flexibility in power system operations with
renewable energy. Applied Energy, 222:872–884.
Ju, Y., Wang, J., Ge, F., Lin, Y., Dong, M., Li, D., Shi,
K., and Zhang, H. (2019). Unit commitment accom-
modating large scale green power. Applied Sciences,
9(8).
Lepr
ˆ
etre, F., Verel, S., Fonlupt, C., and Marion, V. (2019).
Walsh functions as surrogate model for pseudo-
boolean optimization problems. In The Genetic
and Evolutionary Computation Conference (GECCO
2019), Proceedings of the Genetic and Evolution-
ary Computation Conference, pages 303–311, Prague,
Czech Republic. ACM.
L
´
opez-Ib
´
a
˜
nez, M., Paquete, L., and St
¨
utzle, T. (2010).
Exploratory analysis of stochastic local search al-
gorithms in biobjective optimization. In Bartz-
Beielstein, T., Chiarandini, M., Paquete, L., and
Preuss, M., editors, Experimental Methods for the
Analysis of Optimization Algorithms, pages 209–222.
Springer, Berlin, Germany.
Mahfudin, I., Ardiyanto, I., and Cahyadi, A. I. (2022).
Auto-tuning pid controller for nuscale nuclear reactor
using point reactor kinetics model simulator. In 2022
9th International Conference on Information Tech-
nology, Computer, and Electrical Engineering (ICI-
TACEE), pages 7–12.
Muniglia, M., Do, J.-M., Jean-Charles, L. P., Grard, H.,
Verel, S., and David, S. (2016). A multi-physics
pwr model for the load following. In Interna-
tional Congress on Advances in Nuclear Power Plants
(ICAPP).
Muniglia, M., Verel, S., Le Pallec, J.-C., and Do, J.-M.
(2018). A fitness landscape view on the tuning of
an asynchronous master-worker ea for nuclear reactor
design. In Artificial Evolution, pages 30–46. Springer
International Publishing.
O’Donnell, R. (2021). Analysis of boolean functions.
Pedregosa, F., Varoquaux, G., Gramfort, A., Michel, V.,
Thirion, B., Grisel, O., Blondel, M., Prettenhofer,
P., Weiss, R., Dubourg, V., Vanderplas, J., Passos,
A., Cournapeau, D., Brucher, M., Perrot, M., and
Duchesnay, E. (2011). Scikit-learn: Machine learning
in Python. Journal of Machine Learning Research,
12:2825–2830.
Prieto, J. and G
´
omez, J. (2020). Hybrid adaptive evolution-
ary algorithm for multi-objective optimization. ArXiv,
abs/2004.13925.
Qi, Y., Ma, X., Liu, F., Jiao, L., Sun, J., and Wu, J. (2014).
MOEA/D with Adaptive Weight Adjustment. Evolu-
tionary Computation, 22(2):231–264.
Rafiei, M., Ansarifar, G., Hadad, K., and Mohammadi, M.
(2021). Load-following control of a nuclear reactor
using optimized fopid controller based on the two-
point fractional neutron kinetics model considering re-
activity feedback effects. Progress in Nuclear Energy,
141:103936.
Shim, V. A., Tan, K. C., and Tan, K. K. (2012). A hybrid
adaptive evolutionary algorithm in the domination-
based and decomposition-based frameworks of multi-
objective optimization. In 2012 IEEE Congress on
Evolutionary Computation, pages 1–8.
Verel, S., Derbel, B., Liefooghe, A., Aguirre, H., and
Tanaka, K. (2018). A surrogate model based on walsh
decomposition for pseudo-boolean functions. In Par-
allel Problem Solving from Nature – PPSN XV, pages
181–193, Cham. Springer International Publishing.
Walsh, J. L. (1923). A closed set of normal orthogonal func-
tions. American Journal of Mathematics, 45:5–24.
Xu, Y., Wang, Z., Sun, W., Chen, S., Wu, Y., and Zhao, B.
(2011). Unit commitment model considering nuclear
power plant load following. APAP 2011 - Proceed-
ings: 2011 International Conference on Advanced
Power System Automation and Protection, 3.
Zeng, W., Jiang, Q., Xie, J., and Yu, T. (2020). A functional
variable universe fuzzy pid controller for load follow-
ing operation of pwr with the multiple model. Annals
of Nuclear Energy, 140:107174.
Zhang, Q. and Li, H. (2007). Moea/d: A multiob-
jective evolutionary algorithm based on decomposi-
tion. IEEE Transactions on evolutionary computation,
11(6):712–731.
MOEA/D with Adaptive Mutation Operator Based on Walsh Decomposition: Application to Nuclear Reactor Control Optimization
85