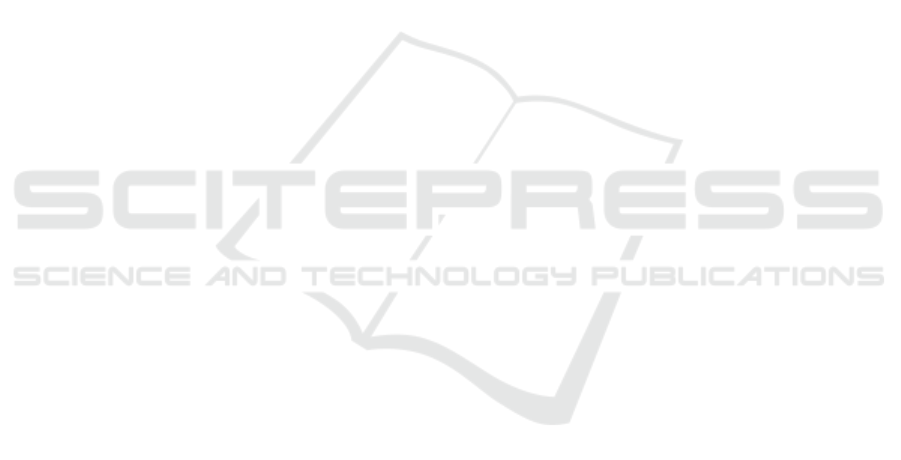
tion. In Proc. Neural Information Processing Systems,
NeurIPS ’11. Curran Associates, Inc.
Boreiko, V., Augustin, M., Croce, F., Berens, P., and Hein,
M. (2022). Sparse visual counterfactual explanations
in image space. In Proc. DAGM German Conference
on Pattern Recognition, GCPR ’22, pages 133–148.
Springer International Publishing.
Castelvecchi, D. (2016). Can we open the black box of AI?
Nature News, 538(7623):20–23.
Chen, I. Y., Pierson, E., Rose, S., Joshi, S., Ferryman, K.,
and Ghassemi, M. (2021). Ethical machine learning
in healthcare. Annual Review of Biomedical Data Sci-
ence, 4:123–144.
Chen, L., Yan, X., Xiao, J., Zhang, H., Pu, S., and Zhuang,
Y. (2020). Counterfactual samples synthesizing for
robust visual question answering. In Proc. IEEE/CVF
Conference on Computer Vision and Pattern Recogni-
tion, pages 10797–10806. IEEE.
Gomez, O., Holter, S., Yuan, J., and Bertini, E. (2020).
ViCE: Visual counterfactual explanations for machine
learning models. In Proc. 25th International Con-
ference on Intelligent User Interfaces, IUI ’20, pages
531–535. ACM.
Goyal, Y., Khot, T., Summers-Stay, D., Batra, D., and
Parikh, D. (2017). Making the V in VQA matter: Ele-
vating the role of image understanding in visual ques-
tion answering. In Proc. IEEE/CVF Conference on
Computer Vision and Pattern Recognition, CVPR ’17,
pages 6325–6334. IEEE.
Goyal, Y., Wu, Z., Ernst, J., Batra, D., Parikh, D., and
Lee, S. (2019). Counterfactual visual explanations.
In Proc. 36th International Conference on Machine
Learning, PMLR ’19, pages 2376–2384. PMLR.
He, K., Zhang, X., Ren, S., and Sun, J. (2016). Deep
residual learning for image recognition. In Proc. Con-
ference on Computer Vision and Pattern Recognition,
CVPR ’16, pages 770–778. IEEE.
Hendricks, L. A., Akata, Z., Rohrbach, M., Donahue, J.,
Schiele, B., and Darrell, T. (2016). Generating visual
explanations. In Proc. European Conference on Com-
puter Vision, ECCV ’16, pages 3–19.
Mothilal, R. K., Sharma, A., and Tan, C. (2020). Explaining
machine learning classifiers through diverse counter-
factual explanations. In Proc. Conference on Fairness,
Accountability, and Transparency, FAT* ’20, pages
607–617. ACM.
Pearl, J. (2000). Causality: Models, Reasoning and Infer-
ence. Cambridge University Press.
Pearson, K. (1896). VII. Mathematical contributions to the
theory of evolution.—III. Regression, heredity, and
panmixia. Philosophical Transactions of the Royal
Society of London. Series A, containing papers of a
mathematical or physical character, 187:253–318.
Petsiuk, V., Jain, R., Manjunatha, V., Morariu, V. I., Mehra,
A., Ordonez, V., and Saenko, K. (2021). Black-box
explanation of object detectors via saliency maps. In
Proc. IEEE/CVF Conference on Computer Vision and
Pattern Recognition, CVPR ’21, pages 11438–11447.
IEEE.
Rosenfeld, A. (2021). Better metrics for evaluating ex-
plainable artificial intelligence. In Proc. 20th Interna-
tional Conference on Autonomous Agents and Multia-
gent Systems, AAMAS ’21, pages 45–50. IFAAMAS.
Rudin, C. (2019). Stop explaining black box machine learn-
ing models for high stakes decisions and use inter-
pretable models instead. Nature Machine Intelligence,
1(5):206–215.
Schwarting, W., Alonso-Mora, J., and Rus, D. (2018). Plan-
ning and decision-making for autonomous vehicles.
Annual Review of Control, Robotics, and Autonomous
Systems, 1:187–210.
Simonyan, K., Vedaldi, A., and Zisserman, A. (2014).
Deep inside convolutional networks: Visualising im-
age classification models and saliency maps. In Proc.
2nd International Conference on Learning Represen-
tations – Workshops Track, ICLR ’14.
Simonyan, K. and Zisserman, A. (2015). Very deep convo-
lutional networks for large-scale image recognition. In
Proc. 3rd International Conference on Learning Rep-
resentations, ICLR ’15, pages 1–14. Computational
and Biological Learning Society.
Spearman, C. E. (1904). The proof and measurement of
association between two things. American Journal of
Psychology, 15(1):72–101.
Vandenhende, S., Mahajan, D., Radenovic, F., and Ghadi-
yaram, D. (2022). Making heads or tails: Towards se-
mantically consistent visual counterfactuals. In Proc.
European Conference on Computer Vision, ECCV
’22, pages 261–279. Springer Nature Switzerland.
Vilone, G. and Longo, L. (2021). Notions of explainability
and evaluation approaches for explainable artificial in-
telligence. Information Fusion, 76:89–106.
Wachter, S., Mittelstadt, B., and Russell, C. (2018). Coun-
terfactual explanations without opening the black box:
Automated decisions and the GDPR. Harvard Journal
of Law & Technology, 31(2):841–887.
Wachter, S., Mittelstadt, B., and Russell, C. (2020). Bias
preservation in machine learning: The legality of fair-
ness metrics under EU non-discrimination law. West
Virginia Law Review, 123(3):735–790.
Wah, C., Branson, S., Welinder, P., Perona, P., and Be-
longie, S. (2011). The Caltech-UCSD Birds-200-2011
dataset. Technical Report CNS-TR-2011-001, Cali-
fornia Institute of Technology.
Yun, S., Han, D., Oh, S. J., Chun, S., Choe, J., and Yoo,
Y. (2019). CutMix: Regularization strategy to train
strong classifiers with localizable features. In Proc.
IEEE/CVF International Conference on Computer Vi-
sion, (ICCV ’19, pages 6022–6031. IEEE.
Zhang, B., Anderljung, M., Kahn, L., Dreksler, N.,
Horowitz, M. C., and Dafoe, A. (2021). Ethics and
governance of artificial intelligence: Evidence from
a survey of machine learning researchers. Journal of
Artificial Intelligence Research, 71:591–666.
KDIR 2023 - 15th International Conference on Knowledge Discovery and Information Retrieval
74