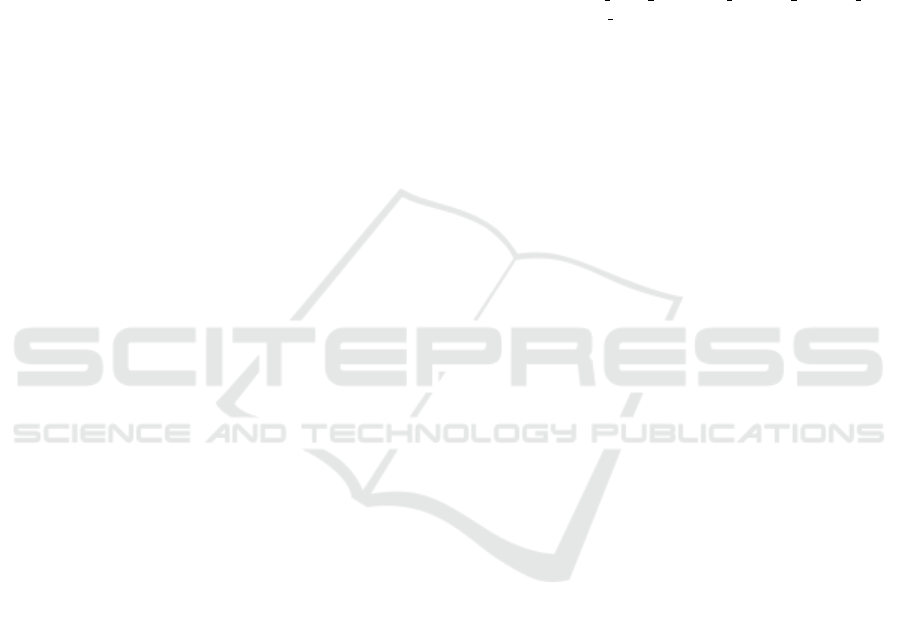
techniques in advanced persistent threat attribution
and detection. In Thomas, C., editor, Data Mining,
chapter 5. IntechOpen, Rijeka.
Chen, P., Desmet, L., and Huygens, C. (2014). A study
on advanced persistent threats. In De Decker, B.
and Z
´
uquete, A., editors, Communications and Mul-
timedia Security, pages 63–72, Berlin, Heidelberg.
Springer Berlin Heidelberg.
Chen, T. and Guestrin, C. (2016). Xgboost: A scalable
tree boosting system. In Proceedings of the 22nd
ACM SIGKDD International Conference on Knowl-
edge Discovery and Data Mining, KDD ’16, page
785–794, New York, NY, USA. Association for Com-
puting Machinery.
D’Agostino, J. and Kul, G. (2021). Toward pinpointing
data leakage from advanced persistent threats. In
2021 7th IEEE Intl Conference on Big Data Security
on Cloud (BigDataSecurity), IEEE Intl Conference
on High Performance and Smart Computing, (HPSC)
and IEEE Intl Conference on Intelligent Data and Se-
curity (IDS), pages 157–162.
Edgar, T. W. and Manz, D. O. (2017). Chapter 11 - applied
experimentation. In Edgar, T. W. and Manz, D. O.,
editors, Research Methods for Cyber Security, pages
271–297. Syngress.
Eric, C., Liam, O., and Nicolas, F. (2012). W32.duqu: The
precursor to the next stuxnet. In USENIX Workshop
on Large-Scale Exploits and Emergent Threats.
Ghafir, I., Hammoudeh, M., Prenosil, V., Han, L., Hegarty,
R., Rabie, K., and Aparicio-Navarro, F. J. (2018). De-
tection of advanced persistent threat using machine-
learning correlation analysis. Future Generation
Computer Systems, 89:349–359.
Irshad, H., Ciocarlie, G., Gehani, A., Yegneswaran, V., Lee,
K. H., Patel, J., Jha, S., Kwon, Y., Xu, D., and Zhang,
X. (2021). Trace: Enterprise-wide provenance track-
ing for real-time apt detection. IEEE Transactions on
Information Forensics and Security, 16:4363–4376.
King, J., Bendiab, G., Savage, N., and Shiaeles, S. (2021).
Data exfiltration: Methods and detection countermea-
sures. In 2021 IEEE International Conference on Cy-
ber Security and Resilience (CSR), pages 442–447.
Kostas, K. (2018). Anomaly Detection in Networks Using
Machine Learning. Master’s thesis, University of Es-
sex, Colchester, UK.
Lal, A., Prasad, A., Kumar, A., and Kumar, S. (2022).
Data exfiltration: Preventive and detective counter-
measures. In Proceedings of the International Con-
ference on Innovative Computing & Communication
(ICICC) 2022.
Mamun, M. and Shi, K. (2021). Deeptaskapt: Insider apt
detection using task-tree based deep learning. In 2021
IEEE 20th International Conference on Trust, Secu-
rity and Privacy in Computing and Communications
(TrustCom), pages 693–700.
Mandiant (2014). APT1 — Exposing One of China’s
Cyber Espionage Units — mandiant.com.
https://www.mandiant.com/resources/reports/
apt1-exposing-one-chinas-cyber-espionage-units.
[Accessed 18-April-2023].
Mengqi, Z., Yang, L., Guangxi, Y., Bo, L., and Weiping, W.
(2022). Detecting dns over https based data exfiltra-
tion. Computer Networks, 209:108919.
Moghaddam, A. K. and Zincir-Heywood, N. (2020). Ex-
ploring data leakage in encrypted payload using su-
pervised machine learning. Proceedings of the 15th
International Conference on Availability, Reliability
and Security.
NSA, CISA, FBI, and NCSC (2021). Russian gru
conducting global brute force campaign to com-
promise enterprise and cloud environments.
https://media.defense.gov/2021/Jul/01/2002753896/
-1/-1/1/CSA
GRU GLOBAL BRUTE FORCE
CAMPAIGN UOO158036-21.PDF. [Accessed
15-April-2023].
Sabir, B., Ullah, F., Babar, M. A., and Gaire, R. (2021).
Machine learning for detecting data exfiltration: A re-
view. ACM Comput. Surv., 54(3).
Sabir, B., Ullah, F., Babar, M. A., and Gaire, R. (2022). Ma-
chine Learning for Detecting Data Exfiltration. ACM
Computing Surveys, 54(3):1–47.
Sowmya, M., Kritshekhar, J., Abdulhakim, S., Garima, A.,
Yuli, D., Ankur, C., and Dijiang, H. (2023). Unrav-
eled — a semi-synthetic dataset for advanced persis-
tent threats. Computer Networks, 227:109688.
Stojanovi
´
c, B., Hofer-Schmitz, K., and Kleb, U. (2020).
Apt datasets and attack modeling for automated de-
tection methods: A review. Computers & Security,
92:101734.
Thongsuwan, S., Jaiyen, S., Padcharoen, A., and Agarwal,
P. (2021). Convxgb: A new deep learning model for
classification problems based on cnn and xgboost. Nu-
clear Engineering and Technology, 53(2):522–531.
Veena, R. C. and Brahmananda, S. H. (2022). A frame-
work for apt detection based on host destination
and packet—analysis. In Smys, S., Bestak, R.,
Palanisamy, R., and Kotuliak, I., editors, Computer
Networks and Inventive Communication Technolo-
gies, pages 833–840, Singapore. Springer Singapore.
Xianrui, M. and Joan, F. (2020). Privacy-preserving xg-
boost inference. In NeurIPS 2020 Workshop on Pri-
vacy Preserving Machine Learning.
Zebin, T., Rezvy, S., and Luo, Y. (2022). An explain-
able ai-based intrusion detection system for dns over
https (doh) attacks. IEEE Transactions on Information
Forensics and Security, 17:2339–2349.
Zimba, A., Chen, H., Wang, Z., and Chishimba, M.
(2020). Modeling and detection of the multi-stages
of Advanced Persistent Threats attacks based on
semi-supervised learning and complex networks char-
acteristics. Future Generation Computer Systems,
106:501–517.
Zou, Q., Singhal, A., Sun, X., and Liu, P. (2020). Auto-
matic recognition of advanced persistent threat tactics
for enterprise security. In Proceedings of the Sixth In-
ternational Workshop on Security and Privacy Ana-
lytics, IWSPA ’20, page 43–52, New York, NY, USA.
Association for Computing Machinery.
New Perspectives on Data Exfiltration Detection for Advanced Persistent Threats Based on Ensemble Deep Learning Tree
285