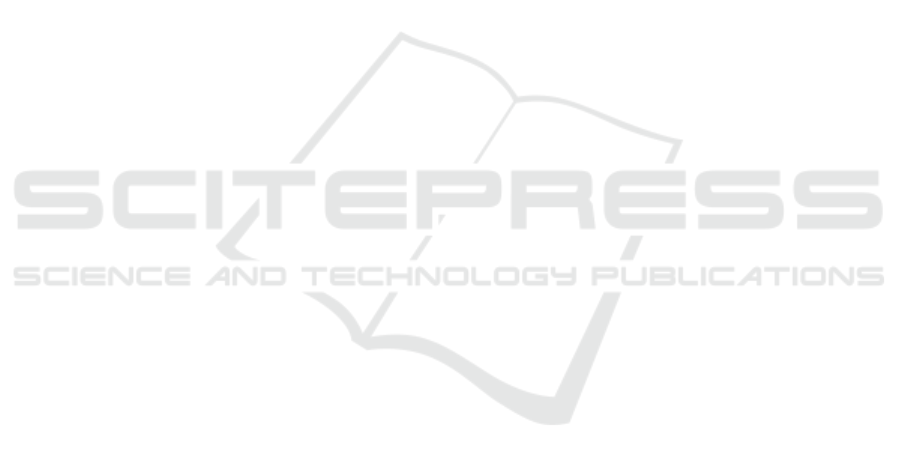
VirusViz: comparative analysis and effective visual-
ization of viral nucleotide and amino acid variants.
Nucleic Acids Research, 49(15):e90–e90.
Bernasconi, A., Mari, L., Casagrandi, R., and Ceri, S.
(2021b). Data-driven analysis of amino acid change
dynamics timely reveals SARS-CoV-2 variant emer-
gence. Scientific Reports, 11(1):21068.
Booch, G., Rumbaugh, J., and Jackobson, I. (1997). The
Unified Modeling Language User Guide. Addison-
Wesley Object Technology Series – Pearson Educa-
tion (US).
Borgo, S. and Masolo, C. (2009). Handbook on Ontologies.
Springer Verlag.
Fabio, I., Amaral, G. C., Griffo, C., Bai
˜
ao, F., and Guiz-
zardi, G. (2021). “What exactly is a lockdown?”: to-
wards an ontology-based modeling of lockdown inter-
ventions during the COVID-19 pandemic. In ONTO-
BRAS, pages 151–165.
Garc
´
ıa S, A., Bernasconi, A., Guizzardi, G., Pastor, O.,
Storey, V. C., and Costa, M. (2022a). An Initial Empir-
ical Assessment of an Ontological Model of the Hu-
man Genome. In Advances in Conceptual Modeling,
pages 55–65, Cham. Springer International Publish-
ing.
Garc
´
ıa S., A., Bernasconi, A., Guizzardi, G., Pastor, O.,
Storey, V. C., and Panach, I. (2023). Assessing the
value of ontologically unpacking a conceptual model
for human genomics. Information Systems, page
102242.
Garc
´
ıa S, A., Guizzardi, G., Pastor, O., Storey, V. C., and
Bernasconi, A. (2022b). An ontological characteri-
zation of a conceptual model of the human genome.
In Intelligent Information Systems: CAiSE Forum
2022, Leuven, Belgium, June 6–10, 2022, Proceed-
ings, pages 27–35. Springer.
Guizzardi, G. (2005). Ontological foundations for struc-
tural conceptual models. CTIT, Centre for Telematics
and Information Technology, Twente, Netherlands.
Guizzardi, G., Bernasconi, A., Pastor, O., and Storey, V. C.
(2021). Ontological unpacking as explanation: the
case of the viral conceptual model. In Conceptual
Modeling: 40th International Conference, ER 2021,
Virtual Event, October 18–21, 2021, Proceedings 40,
pages 356–366. Springer.
Guizzardi, G. and Guarino, N. (2023). Semantics, ontology
and explanation. arXiv preprint arXiv:2304.11124.
Guizzardi, G. and Wagner, G. (2004). A unified founda-
tional ontology and some applications of it in business
modeling. In Proceedings of the Open InterOp Work-
shop on Enterprise Modelling and Ontologies for In-
teroperability, co-located with CAiSE’04 Conference.
He, Y., Yu, H., Ong, E., Wang, Y., Liu, Y., Huffman, A.,
Huang, H.-h., Beverley, J., Hur, J., Yang, X., et al.
(2020). CIDO, a community-based ontology for coro-
navirus disease knowledge and data integration, shar-
ing, and analysis. Scientific data, 7:181.
Lauring, A. S., Frydman, J., and Andino, R. (2013). The
role of mutational robustness in RNA virus evolution.
Nature Reviews Microbiology, 11(5):327–336.
Mattenberger, F., Vila-Nistal, M., and Geller, R. (2021). In-
creased rna virus population diversity improves adapt-
ability. Scientific Reports, 11:6824.
Niles, I. and Pease, A. (2001). Towards a standard upper on-
tology. In Proceedings of the international conference
on Formal Ontology in Information Systems-Volume
2001, pages 2–9.
Poli, R., Healy, M., and Kameas, A. (2010). Theory
and applications of ontology: Computer applications.
Springer.
Romanenko, E., Calvanese, D., and Guizzardi, G. (2022).
Towards pragmatic explanations for domain ontolo-
gies. In Knowledge Engineering and Knowledge
Management: 23rd International Conference, EKAW
2022, Bolzano, Italy, September 26–29, 2022, Pro-
ceedings, pages 201–208. Springer.
Serna Garc
´
ıa, G., Al Khalaf, R., Invernici, F., Ceri, S., and
Bernasconi, A. (2023a). Coveffect: interactive sys-
tem for mining the effects of sars-cov-2 mutations
and variants based on deep learning. GigaScience,
12:giad036.
Serna Garc
´
ıa, G., Al Khalaf, R., Invernici, F., Ceri,
S., and Bernasconi, A. (2023b). Supporting
data for ”coveffect: Interactive system for min-
ing the effects of sars-cov-2 mutations and vari-
ants based on deep learning”, GigaScience Database.
http://dx.doi.org/10.5524/102386. Last accessed: Aug
2023.
Smith, B., Ashburner, M., Rosse, C., Bard, J., Bug, W.,
Ceusters, W., Goldberg, L. J., Eilbeck, K., Ireland, A.,
Mungall, C. J., et al. (2007). The OBO Foundry: coor-
dinated evolution of ontologies to support biomedical
data integration. Nature biotechnology, 25(11):1251–
1255.
Tanaka, H., Hirayama, A., Nagai, H., Shirai, C., Taka-
hashi, Y., Shinomiya, H., Taniguchi, C., and Ogata, T.
(2021). Increased transmissibility of the SARS-CoV-2
Alpha variant in a Japanese population. International
journal of environmental research and public health,
18(15):7752.
Verdonck, M. and Gailly, F. (2016). Insights on the use
and application of ontology and conceptual modeling
languages in ontology-driven conceptual modeling.
In Conceptual Modeling: 35th International Confer-
ence, ER 2016, Gifu, Japan, November 14-17, 2016,
Proceedings 35, pages 83–97. Springer.
Verdonck, M., Gailly, F., Pergl, R., Guizzardi, G., Mar-
tins, B., and Pastor, O. (2019). Comparing traditional
conceptual modeling with ontology-driven conceptual
modeling: An empirical study. Information Systems,
81:92–103.
Wang, L. L., Lo, K., Chandrasekhar, Y., Reas, R., Yang,
J., Eide, D., Funk, K., Kinney, R., Liu, Z., Merrill,
W., et al. (2020). CORD-19: The COVID-19 Open
Research Dataset. ArXiv.
Wheelock, E. F. and Toy, S. T. (1973). Participation of lym-
phocytes in viral infections. Advances in immunology,
16:123–184.
World Health Organization (2023). Statement on
the fifteenth meeting of the IHR (2005) Emer-
gency Committee on the COVID-19 pan-
demic. https://www.who.int/news/item/05-05-
2023-statement-on-the-fifteenth-meeting-of-the-
international-health-regulations. Last accessed: Aug
2023.
KEOD 2023 - 15th International Conference on Knowledge Engineering and Ontology Development
72