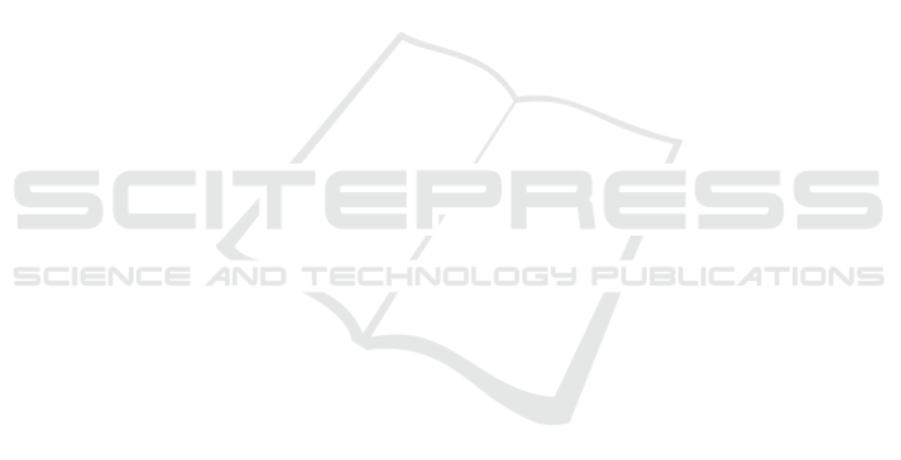
REFERENCES
Baker, K. (2021). Solutions of dc opf are never ac feasible.
In Proceedings of the Twelfth ACM International Con-
ference on Future Energy Systems, pages 264–268.
Bertsekas, D. (2016). Nonlinear Programming, volume 4.
Athena Scientific.
Capitanescu, F. (2011). Assessing reactive power re-
serves with respect to operating constraints and volt-
age stability. IEEE Transactions on Power Systems,
26(4):2224–2234.
Carpentier, J. (1962). Contribution to the economic dis-
patch problem. Bulletin de la Societe Francoise des
Electriciens, 3(8):431–447.
Dener, A., Miller, M. A., Churchill, R. M., Munson, T., and
Chang, C.-S. (2020). Training neural networks under
physical constraints using a stochastic augmented la-
grangian approach. arXiv preprint arXiv:2009.07330.
Djeumou, F., Neary, C., Goubault, E., Putot, S., and Topcu,
U. (2022). Neural networks with physics-informed
architectures and constraints for dynamical systems
modeling. In Learning for Dynamics and Control
Conference, pages 263–277. PMLR.
Falconer, T. and Mones, L. (2022). Leveraging power grid
topology in machine learning assisted optimal power
flow.
Fioretto, F., Mak, T. W., and Van Hentenryck, P. (2020).
Predicting ac optimal power flows: Combining deep
learning and lagrangian dual methods. In Proceedings
of the AAAI conference on artificial intelligence, vol-
ume 34, pages 630–637.
Huang, W., Pan, X., Chen, M., and Low, S. H. (2021).
Deepopf-v: Solving ac-opf problems efficiently. IEEE
Transactions on Power Systems, 37(1):800–803.
Kingma, D. P. and Ba, J. (2017). Adam: A method for
stochastic optimization.
Lavaei, J. and Low, S. H. (2011). Zero duality gap in
optimal power flow problem. IEEE Transactions on
Power Systems, 27(1):92–107.
Low, S. H. (2014). Convex relaxation of optimal power
flow—part i: Formulations and equivalence. IEEE
Transactions on Control of Network Systems, 1(1):15–
27.
Lu, L., Pestourie, R., Yao, W., Wang, Z., Verdugo, F.,
and Johnson, S. G. (2021). Physics-informed neu-
ral networks with hard constraints for inverse design.
SIAM Journal on Scientific Computing, 43(6):B1105–
B1132.
Pan, X., Chen, M., Zhao, T., and Low, S. H. (2022). Deep-
opf: A feasibility-optimized deep neural network ap-
proach for ac optimal power flow problems.
Pan, X., Zhao, T., and Chen, M. (2020). Deepopf: Deep
neural network for dc optimal power flow.
Skolfield, J. K. and Escobedo, A. R. (2022). Operations
research in optimal power flow: A guide to recent and
emerging methodologies and applications. European
Journal of Operational Research, 300(2):387–404.
Tang, Y., Dvijotham, K., and Low, S. (2017). Real-time op-
timal power flow. IEEE Transactions on Smart Grid,
8(6):2963–2973.
Wang, H., Murillo-Sanchez, C. E., Zimmerman, R. D., and
Thomas, R. J. (2007). On computational issues of
market-based optimal power flow. IEEE Transactions
on Power Systems, 22(3):1185–1193.
Zhang, L. and Zhang, B. (2022). Learning to solve the ac
optimal power flow via a lagrangian approach. In 2022
North American Power Symposium (NAPS), pages 1–
6. IEEE.
Zhou, F., Anderson, J., and Low, S. H. (2020). The optimal
power flow operator: Theory and computation.
Zimmerman, R. D., Murillo-S
´
anchez, C. E., and Thomas,
R. J. (2011). Matpower: Steady-state operations, plan-
ning, and analysis tools for power systems research
and education. IEEE Transactions on Power Systems,
26(1):12–19.
ICINCO 2023 - 20th International Conference on Informatics in Control, Automation and Robotics
220