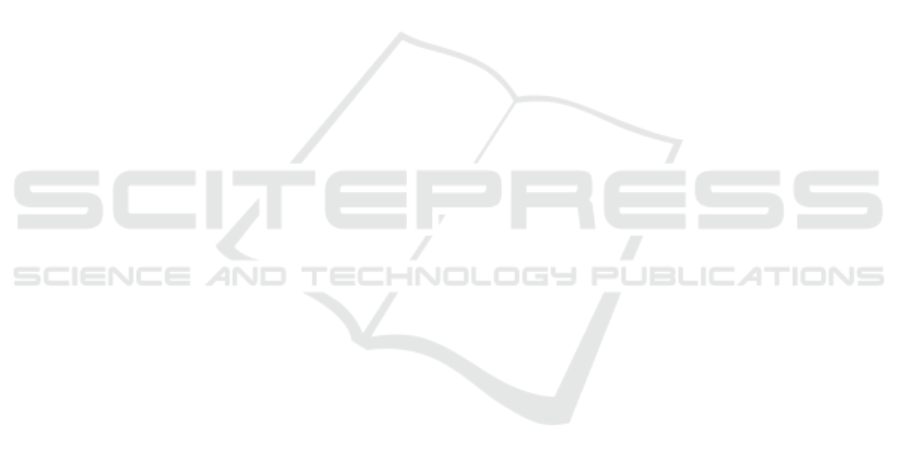
Berger, C., Blauth, R., Boger, D., Bolster, C., Burchill, G.,
DuMouchel, W., Pouliot, F., Richter, R., Rubinoff, A.,
Shen, D., Timko, M., and Walden, D. (1993). The de-
sire for continuous and graphical analysis. In Berger,
C., Blauth, R., Boger, D., Bolster, C., Burchill, G.,
DuMouchel, W., Pouliot, F., Richter, R., Rubinoff, A.,
Shen, D., Timko, M., and Walden, D., editors, Kano’s
methods for understanding customer-defined quality,
volume 2.4, pages 17–20. Center for Quality Manage-
ment Journal.
BVDW (2017). Digital Trends Umfrage zu digitalen
Sprachassistenten (Digital Trends survey on digital
voice assistants).
Chaouali, W., Yahia, I. B., Lunardo, R., and Triki, A.
(2019). Reconsidering the “what is beautiful is good”
effect. International Journal of Bank Marketing.
Chen, C.-C. and Chuang, M.-C. (2008). Integrating the
kano model into a robust design approach to enhance
customer satisfaction with product design. Interna-
tional Journal of Production Economics, 114(2):667–
681. Special Section on Logistics Management in
Fashion Retail Supply Chains.
Czaja, S. J., Charness, N., Fisk, A. D., Hertzog, C., Nair,
S. N., Rogers, W. A., and Sharit, J. (2006). Factors
predicting the use of technology: findings from the
center for research and education on aging and tech-
nology enhancement (CREATE). Psychology and ag-
ing, 21(2):333–352.
DuMouchel, B. (1993). Thoughts on graphical and con-
tinuous analysis for kano’s methods. In Berger, C.,
Blauth, R., Boger, D., Bolster, C., Burchill, G., Du-
Mouchel, W., Pouliot, F., Richter, R., Rubinoff, A.,
Shen, D., Timko, M., and Walden, D., editors, Kano’s
methods for understanding customer-defined quality,
volume 2.4, pages 20–23. Center for Quality Manage-
ment Journal.
Erikson, E. H. (1994). Identity: Youth and Crisis. Norton
& Company.
Fong, D. (1996). Using the self-stated importance question-
naire to interpret kano questionnaire results. Center
for Quality Management Journal, 5:21–23.
Haimes, P. (2021). Beyond beauty: Towards a deeper under-
standing of aesthetics in hci. In Extended Abstracts of
the 2021 CHI Conference on Human Factors in Com-
puting Systems, CHI EA ’21, New York, NY, USA.
Association for Computing Machinery.
Jenkins, A., Eslambolchilar, P., Lindsay, S., Hare, M.,
Thornton, I., and Tales, A. (2016). Attitudes to-
wards attention and aging:: What differences between
younger and older adults tell us about mobile technol-
ogy design. International Journal of Mobile Human
Computer Interaction, 8:47–68.
Kano, N., Seraku, N., Takahashi, F., and Tsuji, S.-i. (1984).
————— (attractive quality and must-be quality).
Journal of the Japanese Society for Quality Control,
31(4):147–156.
Klein, A. M., Deutschl
¨
ander, J., K
¨
olln, K., Rauschenberger,
M., and Escalona, M. J. (2023a). Exploring the Con-
text of Use for Voice User Interfaces: Toward Context-
dependent UX Quality Testing. Journal of Software:
Evolution and Process. [Accepted].
Klein, A. M., Hinderks, A., Rauschenberger, M., and
Thomaschewski, J. (2020a). Exploring voice assistant
risks and potential with technology-based users. In
Proceedings of the 16th International Conference on
Web Information Systems and Technologies - Volume
1: WEBIST,, pages 147–154. SciTePress.
Klein, A. M., Hinderks, A., Schrepp, M., and
Thomaschewski, J. (2020b). Construction of
ueq+ scales for voice quality. In Proceedings of the
Conference on Mensch Und Computer, pages 1–5.
Association for Computing Machinery.
Klein, A. M., Hinderks, A., Schrepp, M., and
Thomaschewski, J. (2020c). Measuring user ex-
perience quality of voice assistants. In 2020 15th
Iberian Conference on Information Systems and
Technologies (CISTI), pages 1–4. IEEEE.
Klein, A. M., K
¨
olln, K., Deutschl
¨
ander, J., and Rauschen-
berger, M. (2023b). Design and Evaluation of Voice
User Interfaces: What should one consider? In
Design, Operation and Evaluation of Mobile Com-
munications: 4th International Conference, MOBILE
2023, Held as Part of the 25th HCI International Con-
ference, HCII 2023, Copenhagen, Denmark, July 23-
28, 2023, Proceedings, pages 167–190.
Klein, A. M., Rauschenberger, M., Thomaschweski, J., and
Escalona, M. J. (2021). Comparing voice assistant
risks and potential with technology-based users: A
study from germany and spain. Journal of Web En-
gineering, 20 − 7.
K
¨
olln, K., Deutschl
¨
ander, J., Klein, A., Rauschenberger,
M., and Winter, D. (2022a). Identifying user expe-
rience aspects for voice user interfaces with inten-
sive users. In Proceedings of the 18th International
Conference on Web Information Systems and Tech-
nologies, pages 385–393. SCITEPRESS - Science and
Technology Publications.
K
¨
olln, K., Deutschl
¨
ander, J., Klein, A. M., Rauschenberger,
M., and Winter, D. (2022b). Protocol for identifying
user experience aspects for voice user interfaces with
intensive users.
K
¨
olln, K., Klein, A. M., Deutschl
¨
ander, J., Winter, D., and
Rauschenberger, M. (2023a). Categorizing ux aspects
for voice user interfaces using the kano model. In
Marchiori, M., Dom
´
ınguez Mayo, F. J., and Filipe, J.,
editors, Web Information Systems and Technologies,
pages 209–228. Springer Nature Switzerland.
K
¨
olln, K., Klein, A. M., Deutschl
¨
ander, J., Winter, D., and
Rauschenberger, M. (2023b). Protocol for categoriz-
ing ux aspects for voice user interfaces using the kano
model.
Lee, C. and Coughlin, J. (2014). Perspective: Older adults’
adoption of technology: An integrated approach to
identifying determinants and barriers. Journal of
Product Innovation Management, 32.
Lee, M. C. and Newcomb, J. F. (1997). Applying the kano
methodology to meet customer requirements: Nasa’s
microgravity science program. Quality Management
Journal, 4(3):95–106.
Lis, J. (2022). How big is the voice assistant market? Ac-
cessed 26-May-2023.
WEBIST 2023 - 19th International Conference on Web Information Systems and Technologies
338