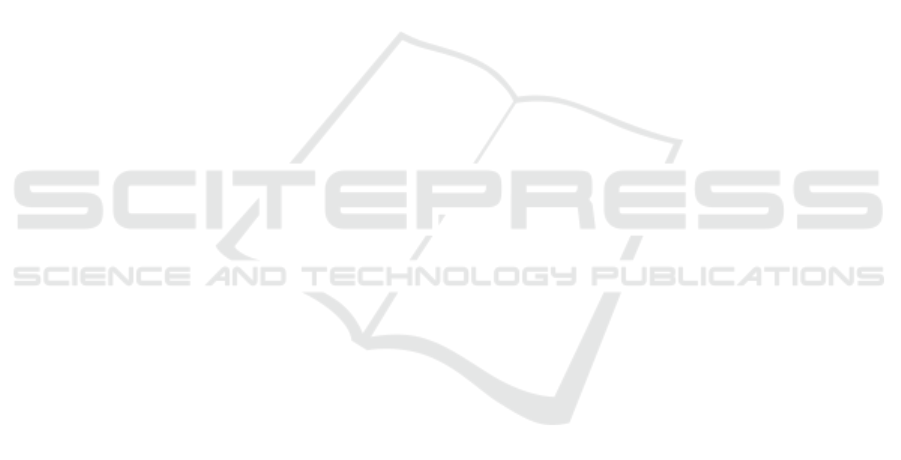
Gu, Y., Tinn, R., Cheng, H., Lucas, M., Usuyama,
N., Liu, X., Naumann, T., Gao, J., and Poon, H.
(2022). Domain-specific language model pretrain-
ing for biomedical natural language processing. ACM
Transactions on Computing for Healthcare, 3(1):1–
23.
Hanahan, D. and Weinberg, R. A. (2000). The hallmarks of
cancer. Cell, 100(1):57–70.
Hanna, J., Joseph, E., Brochhausen, M., and Hogan, W.
(2013). Building a drug ontology based on rxnorm
and other sources. Journal of biomedical semantics,
4:44.
Hertling, S., Portisch, J., and Paulheim, H. (2021). Match-
ing with transformers in melt.
Jin, Q., Dhingra, B., Liu, Z., Cohen, W., and Lu, X. (2019).
PubMedQA: A dataset for biomedical research ques-
tion answering. In Proceedings of (EMNLP-IJCNLP),
pages 2567–2577.
Johnson, A. E., Pollard, T. J., Shen, L., Lehman, L.-w. H.,
Feng, M., Ghassemi, M., Moody, B., Szolovits, P.,
Anthony Celi, L., and Mark, R. G. (2016). MIMIC-
III, a freely accessible critical care database. Scientific
data, 3(1):1–9.
Kanakarajan, K. r., Kundumani, B., and Sankarasubbu, M.
(2021). BioELECTRA: Pretrained biomedical text en-
coder using discriminators. In Proceedings of the 20th
Workshop on Biomedical Language Processing, pages
143–154, Online. Association for Computational Lin-
guistics.
Kass-Hout, T. A., Xu, Z., Mohebbi, M., Nelsen, H., Baker,
A., Levine, J., Johanson, E., and Bright, R. A. (2016).
Openfda: an innovative platform providing access
to a wealth of fda’s publicly available data. Jour-
nal of the American Medical Informatics Association,
23(3):596–600.
Kolyvakis, P., Kalousis, A., and Kiritsis, D. (2018).
Deepalignment: Unsupervised ontology matching
with refined word vectors. In Proceedings of NAACL-
HLT, 787–798., pages 787–798.
Lee, J., Yoon, W., Kim, S., Kim, D., Kim, S., So, C. H.,
and Kang, J. (2020). BioBERT: a pre-trained biomed-
ical language representation model for biomedical text
mining. Bioinformatics, 36(4):1234–1240.
Liu, F., Shareghi, E., Meng, Z., Basaldella, M., and Col-
lier, N. (2021). Self-alignment pretraining for biomed-
ical entity representations. In Proceedings of NAACL-
HLT, pages 4228–4238.
Lynn, S., Arze, C., Nadendla, S., Chang, Y.-W. W.,
Mazaitis, M., Felix, V., Feng, G., and Kibbe, W.
(2011). Disease ontology: A backbone for disease se-
mantic integration. Nucleic acids research, 40:D940–
6.
Mary, M., Soualmia, L., Gansel, X., Darmoni, S., Karls-
son, D., and Schulz, S. (2017). Ontological represen-
tation of laboratory test observables: Challenges and
perspectives in the snomed ct observable entity model
adoption. pages 14–23.
Miller, G. A., Beckwith, R., Fellbaum, C., Gross, D., and
Miller, K. J. (1990). Introduction to wordnet: An on-
line lexical database. International journal of lexicog-
raphy, 3(4):235–244.
Muennighoff, N., Tazi, N., Magne, L., and Reimers, N.
(2022). Mteb: Massive text embedding benchmark.
arXiv preprint arXiv:2210.07316.
Nelson, S. J., Zeng, K., Kilbourne, J., Powell, T., and
Moore, R. (2011). Normalized names for clinical
drugs: RxNorm at 6 years. Journal of the American
Medical Informatics Association, 18(4):441–448.
Nentidis, A., Bougiatiotis, K., Krithara, A., and Paliouras,
G. (2019). Results of the seventh edition of the
BioASQ challenge. In Joint European Conference
on Machine Learning and Knowledge Discovery in
Databases, pages 553–568. Springer.
Osman, I., Ben Yahia, S., and Diallo, G. (2021). Ontol-
ogy integration: Approaches and challenging issues.
Information Fusion, 71:38–63.
Peng, Y., Yan, S., and Lu, Z. (2019). Transfer learn-
ing in biomedical natural language processing: An
evaluation of BERT and ELMo on ten benchmarking
datasets. In Proceedings of the 18th BioNLP Work-
shop and Shared Task, pages 58–65, Florence, Italy.
Association for Computational Linguistics.
Portisch, J., Hladik, M., and Paulheim, H. (2022). Back-
ground knowledge in ontology matching: A survey.
Semantic Web, pages 1–55.
Reimers, N. and Gurevych, I. (2019). Sentence-BERT: Sen-
tence embeddings using Siamese BERT-networks. In
Proceedings of (EMNLP-IJCNLP), pages 3982–3992,
Hong Kong, China. Association for Computational
Linguistics.
Shvaiko, P. and Euzenat, J. (2013). Ontology matching:
State of the art and future challenges. IEEE Transac-
tions on Knowledge and Data Engineering, 25:158–
176.
Vela, J. and Gracia, J. (2022). Cross-lingual ontology
matching with cider-lm: results for oaei 2022.
Wang, K., Reimers, N., and Gurevych, I. (2021). Tsdae:
Using transformer-based sequential denoising auto-
encoderfor unsupervised sentence embedding learn-
ing. In Findings of the Association for Computational
Linguistics: EMNLP 2021, pages 671–688.
Wang, W., Wei, F., Dong, L., Bao, H., Yang, N., and Zhou,
M. (2020). Minilm: Deep self-attention distillation for
task-agnostic compression of pre-trained transform-
ers. Advances in Neural Information Processing Sys-
tems, 33:5776–5788.
Wu, J., Lv, J., Guo, H., and Ma, S. (2020). Daeom: A
deep attentional embedding approach for biomedical
ontology matching. Applied Sciences, 10(21).
Zimmermann, A. and Euzenat, J. (2006). Three semantics
for distributed systems and their relations with align-
ment composition. In The Semantic Web - ISWC 2006,
pages 16–29, Berlin, Heidelberg. Springer Berlin Hei-
delberg.
KEOD 2023 - 15th International Conference on Knowledge Engineering and Ontology Development
84