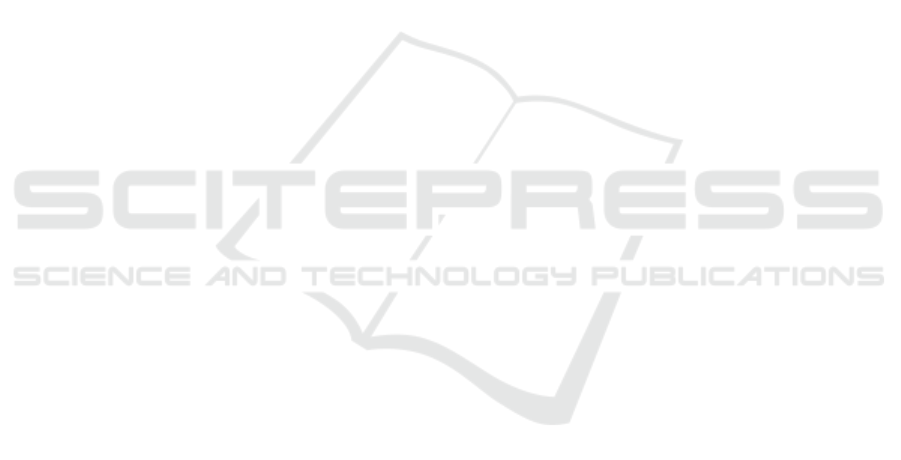
the Eleventh ACM Conference on Recommender Sys-
tems, pages 79–83.
Alshammari, M., Nasraoui, O., and Abdollahi, B. (2018).
A semantically aware explainable recommender sys-
tem using asymmetric matrix factorization. In KDIR,
pages 266–271.
Alshammari, M., Nasraoui, O., and Sanders, S. (2019).
Mining semantic knowledge graphs to add explain-
ability to black box recommender systems. IEEE Ac-
cess, 7:110563–110579.
Alshammari, M. S. (2019). An explainable recommender
system based on semantically-aware matrix factoriza-
tion.
Arrieta, A. B., D
´
ıaz-Rodr
´
ıguez, N., Del Ser, J., Bennetot,
A., Tabik, S., Barbado, A., Garc
´
ıa, S., Gil-L
´
opez, S.,
Molina, D., Benjamins, R., et al. (2020). Explainable
artificial intelligence (xai): Concepts, taxonomies, op-
portunities and challenges toward responsible ai. In-
formation fusion, 58:82–115.
Bilgic, M. and Mooney, R. J. (2005). Explaining recom-
mendations: Satisfaction vs. promotion. In Beyond
Personalization Workshop, IUI, volume 5, page 153.
Ge, L., Gao, J., Yu, X., Fan, W., and Zhang, A. (2012). Es-
timating local information trustworthiness via multi-
source joint matrix factorization. In 2012 IEEE 12th
International Conference on Data Mining, pages 876–
881. IEEE.
Ghassemi, M., Oakden-Rayner, L., and Beam, A. L. (2021).
The false hope of current approaches to explainable
artificial intelligence in health care. The Lancet Digi-
tal Health, 3(11):e745–e750.
Herlocker, J. L., Konstan, J. A., and Riedl, J. (2000). Ex-
plaining collaborative filtering recommendations. In
Proceedings of the 2000 ACM conference on Com-
puter supported cooperative work, pages 241–250.
Herlocker, J. L., Konstan, J. A., Terveen, L. G., and Riedl,
J. T. (2004). Evaluating collaborative filtering recom-
mender systems. ACM Transactions on Information
Systems (TOIS), 22(1):5–53.
J
¨
arvelin, K. and Kek
¨
al
¨
ainen, J. (2017). Ir evaluation meth-
ods for retrieving highly relevant documents. In ACM
SIGIR Forum, volume 51, pages 243–250. ACM New
York, NY, USA.
Koren, Y., Bell, R., and Volinsky, C. (2009). Matrix factor-
ization techniques for recommender systems. Com-
puter, 42(8):30–37.
Lundberg, S. M. and Lee, S.-I. (2017). A unified approach
to interpreting model predictions. In Advances in neu-
ral information processing systems, pages 4765–4774.
McNee, S. M., Riedl, J., and Konstan, J. A. (2006). Being
accurate is not enough: how accuracy metrics have
hurt recommender systems. In CHI’06 extended ab-
stracts on Human factors in computing systems, pages
1097–1101.
Nguyen, J. and Zhu, M. (2013). Content-boosted matrix
factorization techniques for recommender systems.
Statistical Analysis and Data Mining: The ASA Data
Science Journal, 6(4):286–301.
Peake, G. and Wang, J. (2018). Explanation mining: Post
hoc interpretability of latent factor models for rec-
ommendation systems. In Proceedings of the 24th
ACM SIGKDD International Conference on Knowl-
edge Discovery & Data Mining, pages 2060–2069.
Ribeiro, M. T., Singh, S., and Guestrin, C. (2016). Model-
agnostic interpretability of machine learning. arXiv
preprint arXiv:1606.05386.
Ricci, G., de Gemmis, M., and Semeraro, G. (2012). Matrix
and tensor factorization techniques applied to recom-
mender systems: a survey. Matrix, 1(01).
Rudin, C. (2019). Stop explaining black box machine learn-
ing models for high stakes decisions and use inter-
pretable models instead. Nature machine intelligence,
1(5):206–215.
Seton, O., Haghighi, P. S., Alshammari, M., and Nasraoui,
O. (2021). Tag-boosted explainable matrix factoriza-
tion methods for multi-style explanations.
Tintarev, N. and Masthoff, J. (2007). A survey of explana-
tions in recommender systems. In 2007 IEEE 23rd in-
ternational conference on data engineering workshop,
pages 801–810. IEEE.
Tintarev, N. and Masthoff, J. (2015). Explaining recom-
mendations: Design and evaluation. In Recommender
systems handbook, pages 353–382. Springer.
Vig, J., Sen, S., and Riedl, J. (2009). Tagsplanations: ex-
plaining recommendations using tags. In Proceed-
ings of the 14th international conference on Intelligent
user interfaces, pages 47–56.
Enhancing Explainable Matrix Factorization with Tags for Multi-Style Explanations
85