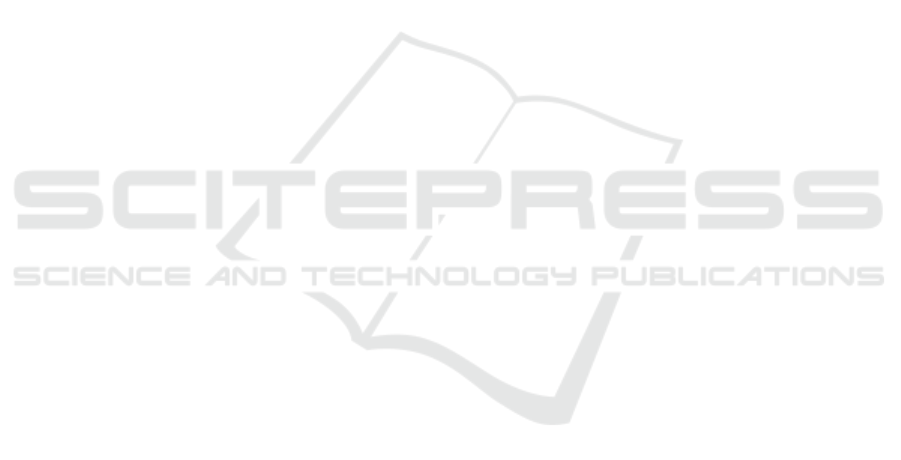
elitist evolutionary algorithm was able to find good re-
source allocations parameters within 150 generations
(Table1). For MOEA, upon using population size of
26, 50 and 100 for generation number 39, 75 and 150
respectively as hyper parameters for our MOEA (see
Table 2), we found that within around 25 generations
the fitness values converge to 98%. These sets of solu-
tions can now be used for allocating resources on Ar-
leigh Bruke Destroyer ship for maximization of area
of coverage and risk minimization (meaning overall
higher strength of coverage). We have calculated av-
erage of best, mean and and worst fitness across 30
random seeds for each generations and noticed that
the mean fitness value starts from 40% fitness value
and reaches a value of 80% within less than 10 gen-
erations and by 25 generations it finds the optimum
set of solutions. Maximum fitness starts somewhere
around 70% and reaches 96% fitness values within
less than 10 generations.
6 CONCLUSION
We formulated two novel resource allocation (RA)
problems for navy ships. The generalized version
of RA focuses on maximization of area of coverage
around ships and finds solutions having 98% area cov-
erage within a given circular boundary. The second
version of the RA problem was defined considering a
DDG-51 ship’s dimensions and ranges of its available
resources. We found that our optimization approach
was able to find solutions representing the location
and orientation of the resources ensuring 98% risk
minimization and area of coverage. Our novel visual
analytic algorithm is capable of generating visualiza-
tion of combined intensity of coverage also known
as heatmaps around DDG-51 at any point of time.
Moreover, tuning the GA for incorporating data based
on location of multiple ships in real time, can lead
to resource allocation in real time and parallel pro-
cessing can help with generating faster outputs. This
opens doors for future works related to integrating
dynamic threatmaps with our multi-objective evolu-
tionary algorithm to enhance the level of protection
around ships in multi-agent scenarios (MAS).
ACKNOWLEDGMENTS
This work has been supported by the Office of Naval
Research award N00014-21-1-2234.
REFERENCES
Ai, B., Li, B., Gao, S., Xu, J., and Shang, H. (2019). An in-
telligent decision algorithm for the generation of mar-
itime search and rescue emergency response plans.
IEEE Access, 7:155835–155850.
Benaskeur, A., Bossé, É., and Blodgett, D. (2007). Com-
bat resource allocation planning in naval engage-
ments. Technical report, DEFENCE RESEARCH
AND DEVELOPMENT CANADA VALCARTIER
(QUEBEC).
Cao, X., Zhang, H., and Peng, M. (2022). Collaborative
multiple access and energy-efficient resource alloca-
tion in distributed maritime wireless networks. China
Communications, 19(4):137–153.
Carlson, L., Navalta, D., Nicolescu, M., Nicolescu, M.,
and Woodward, G. (2019). Multinomial hmms for in-
tent recognition in maritime domains. In Proceedings
of the 18th International Conference on Autonomous
Agents and MultiAgent Systems, pages 1856–1858.
Cleveland, W. S. and McGill, M. (1988). Dynamic graphics
for statistics wadsworth & brooks. Pacific Grove, CA.
Davis, A., Vincent, C., Otenti, N., and Parolin, A. (2016).
Aerial precision 3-d ground surveillance and local-
ization using a network of inexpensive, disposable,
image-based sensors. In 2016 IEEE Symposium on
Technologies for Homeland Security (HST), pages 1–
6. IEEE.
Dkhil, H., Yassine, A., and Chabchoub, H. (2018). Multi-
objective optimization of the integrated problem of lo-
cation assignment and straddle carrier scheduling in
maritime container terminal at import. Journal of the
Operational Research Society, 69(2):247–269.
Dridi, O., Krichen, S., and Guitouni, A. (2012). A multi-
objective optimization approach for resource assign-
ment and task scheduling problem: Application to
maritime domain awareness. In 2012 IEEE Congress
on Evolutionary Computation, pages 1–8. IEEE.
Eshelman, L. J. (1991). The chc adaptive search algorithm:
How to have safe search when engaging in nontradi-
tional genetic recombination. In Foundations of ge-
netic algorithms, volume 1, pages 265–283. Elsevier.
Goldberg, D. E. (1987). Simple genetic algorithms and the
minimal, deceptive problem. Genetic algorithms and
simulated annealing, pages 74–88.
Grefenstette, J. J. (1993). Genetic algorithms and machine
learning. In Proceedings of the sixth annual confer-
ence on Computational learning theory, pages 3–4.
Guo, Y., Ye, Y., Yang, Q., and Yang, K. (2019). A multi-
objective inlp model of sustainable resource allocation
for long-range maritime search and rescue. Sustain-
ability, 11(3):929.
Hassan, S. S., Park, S.-B., Huh, E.-N., and Hong, C. S.
(2023). Seamless and intelligent resource allocation
in 6g maritime networks framework via deep rein-
forcement learning. In 2023 International Conference
on Information Networking (ICOIN), pages 505–510.
IEEE.
Hattaway, S. B. (2008). Adapting the dynamic allocation
of fires and sensors (dafs) model for use in maritime
ICINCO 2023 - 20th International Conference on Informatics in Control, Automation and Robotics
62