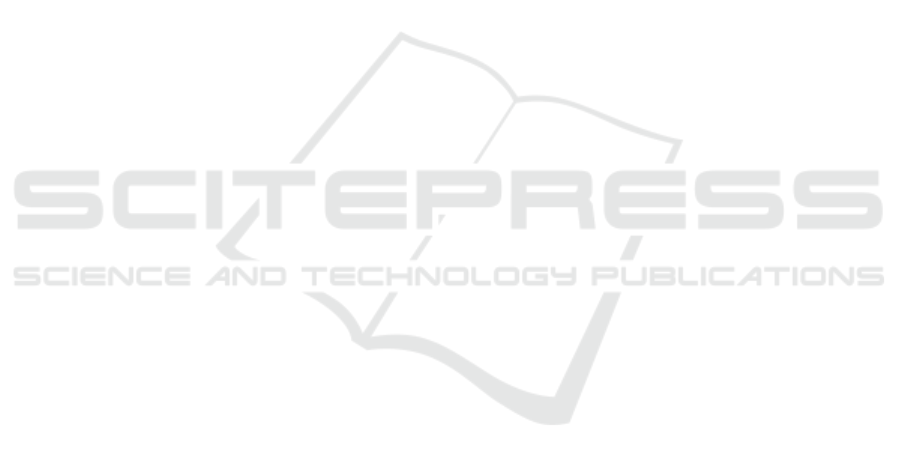
Fanaee-T, H. and Gama, J. (2014). Event labeling combin-
ing ensemble detectors and background knowledge.
Progress in Artificial Intelligence, 2:113–127.
Ghaffari, H. R. (2021). Speeding up the testing and train-
ing time for the support vector machines with minimal
effect on the performance. The Journal of Supercom-
puting, 77(10):11390–11409.
Haeri, H., Beal, C. E., and Jerath, K. (2020). Near-
optimal moving average estimation at characteristic
timescales: An allan variance approach. IEEE Con-
trol Systems Letters, 5(5):1531–1536.
Haeri, H., Beal, C. E., and Jerath, K. (2021). Near-
optimal moving average estimation at characteristic
timescales: An allan variance approach. IEEE Con-
trol Systems Letters, 5(5):1531–1536.
Haeri, H., Soleimani, B., and Jerath, K. (2022). Optimal
moving average estimation of noisy random walks us-
ing allan variance-informed window length. In 2022
American Control Conference (ACC), pages 1646–
1651. IEEE.
Hasan, M. M., Popp, J., and Ol
´
ah, J. (2020). Current land-
scape and influence of big data on finance. Journal of
Big Data, 7(1):1–17.
Hasanin, T., Khoshgoftaar, T. M., Leevy, J. L., and Bauder,
R. A. (2019). Severely imbalanced big data chal-
lenges: investigating data sampling approaches. Jour-
nal of Big Data, 6(1):1–25.
Hogue, J. (2019). Metro Interstate Traffic Vol-
ume. UCI Machine Learning Repository. DOI:
https://doi.org/10.24432/C5X60B.
Holst, A. (2021). Amount of data created, consumed, and
stored 2010-2025. Technology & Telecommunications
Retrieved, pages 06–29.
Hsieh, C.-J., Chang, K.-W., Lin, C.-J., Keerthi, S. S., and
Sundararajan, S. (2008). A dual coordinate descent
method for large-scale linear svm. In Proceedings of
the 25th international conference on Machine learn-
ing, pages 408–415.
Jerath, K., Brennan, S., and Lagoa, C. (2018). Bridging the
gap between sensor noise modeling and sensor char-
acterization. Measurement, 116:350–366.
Jinpeng Wang, A., Qinghong Lin, K., Junhao Zhang, D.,
Weixian Lei, S., and Shou, M. Z. (2023). Too large;
data reduction for vision-language pre-training. arXiv
e-prints, pages arXiv–2305.
Kim, C. D., Jeong, J., and Kim, G. (2020). Imbalanced con-
tinual learning with partitioning reservoir sampling. In
Computer Vision–ECCV 2020: 16th European Con-
ference, Glasgow, UK, August 23–28, 2020, Proceed-
ings, Part XIII 16, pages 411–428. Springer.
Kim, T. and Jerath, K. (2022). Congestion-aware coop-
erative adaptive cruise control for mitigation of self-
organized traffic jams. IEEE Transactions on Intelli-
gent Transportation Systems, 23(7):6621–6632.
Koggalage, R. and Halgamuge, S. (2004). Reducing the
number of training samples for fast support vector ma-
chine classification. Neural Information Processing-
Letters and Reviews, 2(3):57–65.
Lv, Y., Duan, Y., Kang, W., Li, Z., and Wang, F.-Y. (2015).
Traffic flow prediction with big data: A deep learn-
ing approach. IEEE Transactions on Intelligent Trans-
portation Systems, 16(2):865–873.
Maddipatla, S. P., Haeri, H., Jerath, K., and Brennan, S.
(2021). Fast allan variance (favar) and dynamic fast
allan variance (d-favar) algorithms for both regularly
and irregularly sampled data. IFAC-PapersOnLine,
54(20):26–31.
Maddipatla, S. P., Pakala, R., Haeri, H., Chen, C., Jerath,
K., and Brennan, S. (2023). Using databases to imple-
ment algorithms: Estimation of allan variance using
b+-tree data structure. In Proc. of the Modeling, Esti-
mation, and Control Conf. 2023, Lake Tahoe, NV.
Mahmud, M. S., Huang, J. Z., Salloum, S., Emara, T. Z.,
and Sadatdiynov, K. (2020). A survey of data par-
titioning and sampling methods to support big data
analysis. Big Data Mining and Analytics, 3(2).
Nash, W., Sellers, T., Talbot, S., Cawthorn, A., and Ford, W.
(1995). Abalone. UCI Machine Learning Repository.
DOI: https://doi.org/10.24432/C55C7W.
Oates, T. and Jensen, D. (1997). The effects of training
set size on decision tree complexity. In Sixth Interna-
tional Workshop on Artificial Intelligence and Statis-
tics, pages 379–390. PMLR.
Osuna, E. and Girosi, F. (1998). Reducing the run-time
complexity of support vector machines. In Interna-
tional Conference on Pattern Recognition (submitted).
Pace, R. K. and Barry, R. (1997). Sparse spatial autoregres-
sions. Statistics & Probability Letters, 33(3):291–297.
Peng, Y., Lu, Y.-T., and Chen, Z.-G. (2021). An improved
error-based pruning algorithm of decision trees on
large data sets. In 2021 IEEE 6th International Conf.
on Big Data Analytics (ICBDA), pages 33–37. IEEE.
Raghupathi, W. and Raghupathi, V. (2014). Big data an-
alytics in healthcare: promise and potential. Health
information science and systems, 2:1–10.
Sahatiya, P. (2018). Big data analytics on social media data:
a literature review. International Research Journal of
Engineering and Technology, 5(2):189–192.
Sandhu, A. K. (2021). Big data with cloud computing: Dis-
cussions and challenges. Big Data Mining and Ana-
lytics, 5(1):32–40.
Santana, A., Inoue, S., Murakami, K., Iizaka, T., and Mat-
sui, T. (2020). Clustering-based data reduction ap-
proach to speed up svm in classification and regres-
sion tasks. In 33rd International Conference on In-
dustrial, Engineering and Other Applications of Ap-
plied Intelligent Systems, IEA/AIE 2020, Kitakyushu,
Japan, pages 478–488. Springer.
Sinanaj, L. (2021). Allan Variance-based Granulation Tech-
nique for Large Temporal Databases. PhD thesis,
University of Massachusetts Lowell.
Sinanaj, L., Haeri, H., Maddipatla, S. P., Gao, L., Pakala,
R., Kathiriya, N., Beal, C., Brennan, S., Chen, C.,
and Jerath, K. (2022). Granulation of large temporal
databases: An allan variance approach. SN Computer
Science, 4(1):7.
ur Rehman, M. H., Liew, C. S., Abbas, A., Jayaraman, P. P.,
Wah, T. Y., and Khan, S. U. (2016). Big data reduction
methods: a survey. Data Science and Engineering,
1:265–284.
KMIS 2023 - 15th International Conference on Knowledge Management and Information Systems
38