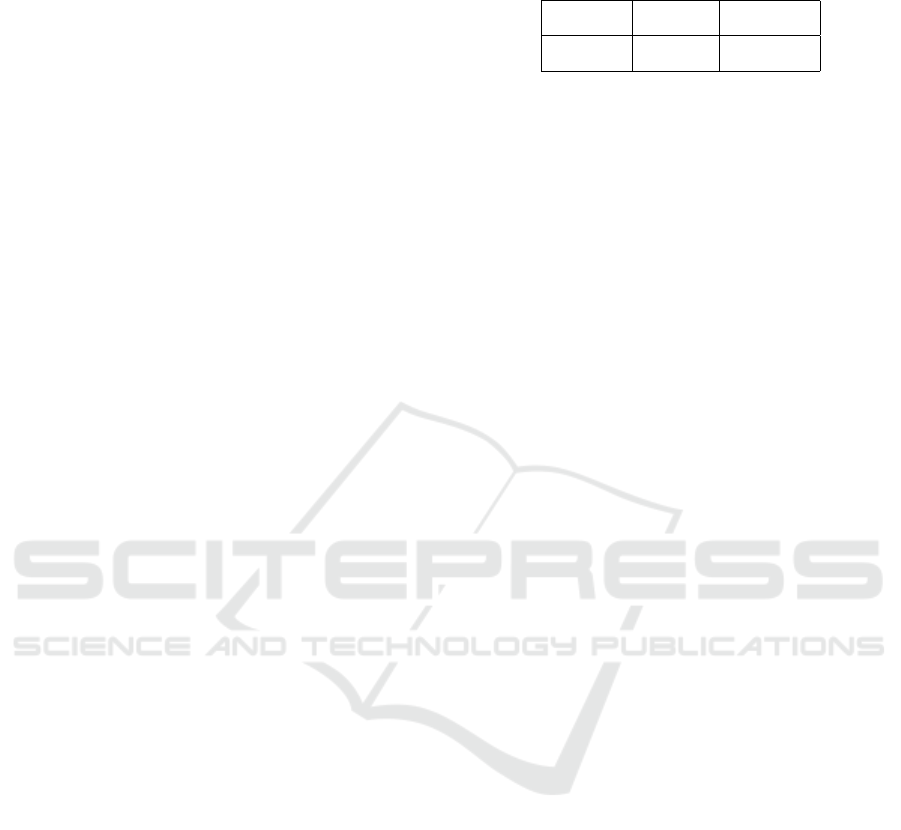
set known as Type-2 fuzzy set (T2 FSs) was intro-
duced by Zadeh in 1975 (Zadeh, 1975), where in this
set, an additional dimension is associated with uncer-
tainty about the degree of membership. For example,
consider the room temperature; whether a tempera-
ture of 27 degrees Celsius belongs to this set or not
may have a degree of membership of 0.9 with a cer-
tainty of 0.5 and a membership of 0.8 with a certainty
of 0.6. Such a problem can be modelled using type-2
fuzzy sets (T2FSs). It is useful when existing uncer-
tain information determines a set’s precise and exact
membership function. In most cases, however, pro-
viding crisp numbers, for example, using the Likert
scale to assess something whether to determine the
level of certainty or measure a degree of belonging to
the set, is problematic (i.e. there could exist uncer-
tainties about them), and thus it is more meaningful
to provide intervals (Wu and Mendel, 2007). There-
fore, the type-2 fuzzy set theory provides a valuable
account of how uncertainties should be handled in
the decision-making process when uncertainty about
words is present.
Multi-criteria decision analysis problems are cat-
egorised as one of the decision-making issues which
received considerable critical attention. It is a prob-
lem which concern finding the most desirable alter-
native(s) from a set of pre-determined alternatives,
A = A
1
, A
2
, ··· , A
n
concerning the decision informa-
tion about criteria weights and criteria values pro-
vided by a group of decision-makers (DMs), DM =
DM
1
, DM
2
, · ·· , DM
m
. However, a significant prob-
lem with dealing with humans as decision-makers is
that they exhibit variation in their decision (Garibaldi
and Ozen, 2007). In order to design an intelligent
decision-making method, such variations should be
considered, especially in the initial of the system it-
self. In other words, the construction of a system
should be aimed to better resemble human reason-
ing in conjunction with using approximate informa-
tion and uncertainty to reach a decision.
In the general framework of fuzzy multi-criteria
decision analysis (MCDA), there exists a technique to
assign a linguistic label (e.g., Very Good, Very Poor,
Fair, etc.) with fuzzy membership functions (MFs)
to represent the performance of each alternative con-
cerning each criterion. For example, in one of the
techniques, known as the Fuzzy Technique for Or-
der Preference by Similarity to Ideal Solution (Fuzzy
TOPSIS) (Chen, 2000), the performance of each alter-
native is evaluated against each criterion using the nu-
merical scale, which then mapped into the fuzzy MFs
with associated parameter (Table 1). The evaluation
from the decision maker is mapped using a fuzzy set
to enhance pre-screening evaluations, where the value
Table 1: Linguistic scale for rating of alternatives in Fuzzy
TOPSIS method.
Poor (P) Fair (F) Good (G)
(0,0,5) (0,5,10) (5,10,10)
of positive rating performance, for example, ‘Good’,
can be approximated in a range of value, for example,
5 − 10.
However, the conventional FTOPSIS used Type-
1 fuzzy sets (T1FSs), characterised by precise mem-
bership functions in the range [0,1], resulting in the
uncertainty disappearing once they have been cho-
sen. In addition, humans as decision-makers exhibit
dynamic behaviour, which causes dynamic variation
in the decision-making process (Ozen and Garibaldi,
2004). Various frameworks based on fuzzy sets have
recently been suggested to model uncertainty. The
main challenge in constructing the model is the gener-
ation of the fuzzy MFs (C. Wagner, 2009; Mendel and
Wu, 2007). In the MCDA framework, this will affect
the overall ranking result at the end of the model. Ad-
ditionally, a lack of investigations has been observed
in the literature on how to construct the MFs and spec-
ify the parameter of MFs in MCDA paradigm. Thus,
in this study, a series of experiments were carried
out by introducing several different levels of small
changes (i.e., uncertainty) in the MFs associated with
the linguistic labels. The purpose of doing this is to
explore any relationship between the amount of un-
certainty (i.e., small changes level) introduced in MFs
and to observe changes in overall decision support
output. In addition, this experiment will lead towards
a proposal of a novel and direct technique to generate
Type-2 MFs for providing a better and more accurate
model of uncertainty based on MCDA technique. Ad-
ditionally, the output results remain in the same form
of information which is in a range of values (i.e., in-
terval form). This type of output result is the main dif-
ference as opposed to the standard MCDA technique,
where in the classical one, it provides output results in
a crisp rank. Thus, this novel technique is interesting
when the input and output of the information are in
the same form. Furthermore, it can minimise the po-
tential for loss of information during the process by
mapping all the information directly to fuzzy sets.
The paper is structured as follows: Section 2
briefly revises the fundamental concepts of fuzzy set
theory and the MCDA method. Section 3 presents the
experimental procedure implementing fuzzy TOPSIS
method. Section 4 provides a discussion of experi-
ment result in the comparison context. Finally, Sec-
tion 5 gives conclusions with suggestions of future
work.
FCTA 2023 - 15th International Conference on Fuzzy Computation Theory and Applications
388