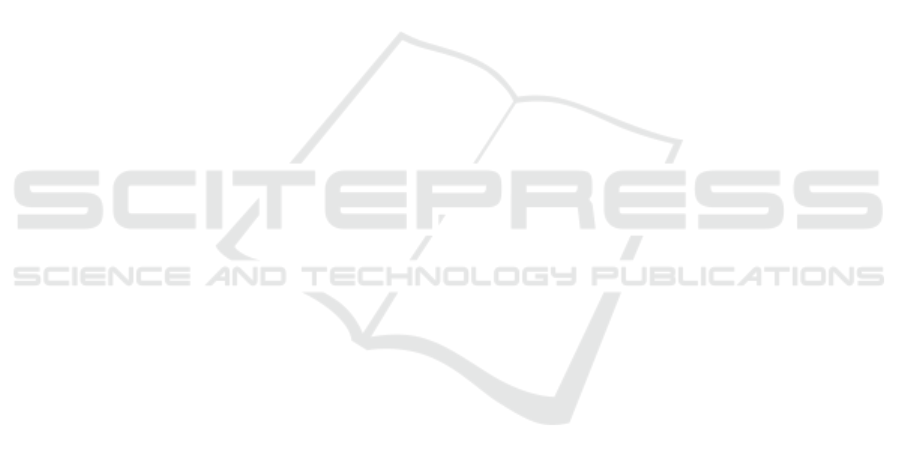
state-of-the-art superpixel methods. IEEE transac-
tions on pattern analysis and machine intelligence, 34.
Achat, S., Marzat, J., and Moras, J. (2022). Path planning
incorporating semantic information for autonomous
robot navigation. In 19th International Conference
on Informatics in Control, Automation and Robotics
(ICINCO), Lisbon, Portugal.
Bartolomei, L., Teixeira, L., and Chli, M. (2020).
Perception-aware path planning for uavs using seman-
tic segmentation. In IEEE/RSJ International Confer-
ence on Intelligent Robots and Systems (IROS), pages
5808–5815.
Behley, J., Garbade, M., Milioto, A., Quenzel, J., Behnke,
S., Stachniss, C., and Gall, J. (2019). SemanticKITTI:
A Dataset for Semantic Scene Understanding of Li-
DAR Sequences. In IEEE/CVF International Conf. on
Computer Vision (ICCV).
Bouman, A., Ginting, M. F., Alatur, N., Palieri, M., Fan,
D. D., Touma, T., Pailevanian, T., Kim, S.-K., Otsu,
K., Burdick, J., and Agha-Mohammadi, A.-a. (2020).
Autonomous Spot: Long-range autonomous explo-
ration of extreme environments with legged locomo-
tion. In IEEE/RSJ International Conference on Intel-
ligent Robots and Systems (IROS), pages 2518–2525.
Chen, X., Li, S., Mersch, B., Wiesmann, L., Gall, J., Behley,
J., and Stachniss, C. (2021). Moving Object Seg-
mentation in 3D LiDAR Data: A Learning-based Ap-
proach Exploiting Sequential Data. IEEE Robotics
and Automation Letters (RA-L), 6:6529–6536.
Cheng, Q., Zeller, N., and Cremers, D. (2022). Vision-
based large-scale 3D semantic mapping for au-
tonomous driving applications. arXiv preprint
arXiv:2203.01087.
Crespo, J., Castillo, J. C., Mozos, O. M., and Barber, R.
(2020). Semantic information for robot navigation: A
survey. Applied Sciences, 10(2).
Dosovitskiy, A., Ros, G., Codevilla, F., Lopez, A., and
Koltun, V. (2017). CARLA: An open urban driving
simulator. In Conference on Robot Learning, pages
1–16.
Ewen, P., Li, A., Chen, Y., Hong, S., and Vasudevan, R.
(2022). These maps are made for walking: Real-time
terrain property estimation for mobile robots. IEEE
Robotics and Automation Letters.
Grinvald, M., Furrer, F., Novkovic, T., Chung, J. J., Ca-
dena, C., Siegwart, R., and Nieto, J. (2019). Volumet-
ric Instance-Aware Semantic Mapping and 3D Object
Discovery. IEEE Robotics and Automation Letters,
4(3):3037–3044.
Grinvald, M., Tombari, F., Siegwart, R., and Nieto, J.
(2021). TSDF++: A Multi-Object Formulation for
Dynamic Object Tracking and Reconstruction. In
2021 IEEE International Conference on Robotics and
Automation (ICRA), pages 14192–14198.
He, K., Gkioxari, G., Dollar, P., and Girshick, R. (2020).
Mask R-CNN. IEEE Transactions on Pattern Analysis
and Machine Intelligence, 42(2):386–397.
Hornung, A., Wurm, K. M., Bennewitz, M., Stachniss,
C., and Burgard, W. (2013). OctoMap: An effi-
cient probabilistic 3D mapping framework based on
octrees. Autonomous Robots. Software available at
http://octomap.github.com.
Hua, B.-S., Pham, Q.-H., Nguyen, D. T., Tran, M.-K., Yu,
L.-F., and Yeung, S.-K. (2016). SceneNN: A scene
meshes dataset with aNNotations. In Fourth Interna-
tional Conference on 3D Vision (3DV), pages 92–101.
Hughes, N., Chang, Y., and Carlone, L. (2022). Hydra: A
real-time spatial perception system for 3D scene graph
construction and optimization. In Robotics: Science
and Systems (RSS).
Jiang, P., Osteen, P., Wigness, M., and Saripalli, S. (2021).
RELLIS-3D dataset: Data, benchmarks and analysis.
In IEEE international Conference on Robotics and
Automation (ICRA), pages 1110–1116.
Kantaros, Y., Kalluraya, S., Jin, Q., and Pappas, G. J.
(2022). Perception-based temporal logic planning
in uncertain semantic maps. IEEE Transactions on
Robotics, 38(4):2536–2556.
Kazhdan, M., Bolitho, M., and Hoppe, H. (2006). Pois-
son surface reconstruction. In Proceedings of the
fourth Eurographics symposium on Geometry pro-
cessing, volume 7, page 0.
Krstini
´
c, D., Braovi
´
c, M.,
ˇ
Seri
´
c, L., and Bo
ˇ
zi
´
c-
ˇ
Stuli
´
c, D.
(2020). Multi-label classifier performance evaluation
with confusion matrix. Computer Science & Informa-
tion Technology, 1.
Lambert, J., Liu, Z., Sener, O., Hays, J., and Koltun, V.
(2020). MSeg: A composite dataset for multi-domain
semantic segmentation. In Computer Vision and Pat-
tern Recognition (CVPR).
Martins, R., Bersan, D., Campos, M. F., and Nascimento,
E. R. (2020). Extending maps with semantic and
contextual object information for robot navigation:
a learning-based framework using visual and depth
cues. Journal of Intelligent & Robotic Systems,
99(3):555–569.
Maturana, D. (2022). Semantic Mapping for Autonomous
Navigation and Exploration. PhD thesis, Carnegie
Mellon University.
McCormac, J., Handa, A., Davison, A., and Leutenegger,
S. (2017). SemanticFusion: Dense 3D semantic map-
ping with convolutional neural networks. In IEEE In-
ternational Conference on Robotics and automation
(ICRA), pages 4628–4635.
M
¨
uller, M. G., Durner, M., Gawel, A., St
¨
urzl, W., Triebel,
R., and Siegwart, R. (2021). A Photorealistic Terrain
Simulation Pipeline for Unstructured Outdoor Envi-
ronments. In IEEE/RSJ International Conference on
Intelligent Robots and Systems.
Nießner, M., Zollh
¨
ofer, M., Izadi, S., and Stamminger,
M. (2013). Real-time 3D reconstruction at scale us-
ing voxel hashing. ACM Transactions on Graphics
(TOG), 32.
Oleynikova, H., Taylor, Z., Fehr, M., Siegwart, R., and Ni-
eto, J. (2017). Voxblox: Incremental 3d euclidean
signed distance fields for on-board mav planning.
In IEEE/RSJ International Conference on Intelligent
Robots and Systems (IROS).
Ono, M., Fuchs, T. J., Steffy, A., Maimone, M., and Yen,
J. (2015). Risk-aware planetary rover operation: Au-
SMaNa: Semantic Mapping and Navigation Architecture for Autonomous Robots
463