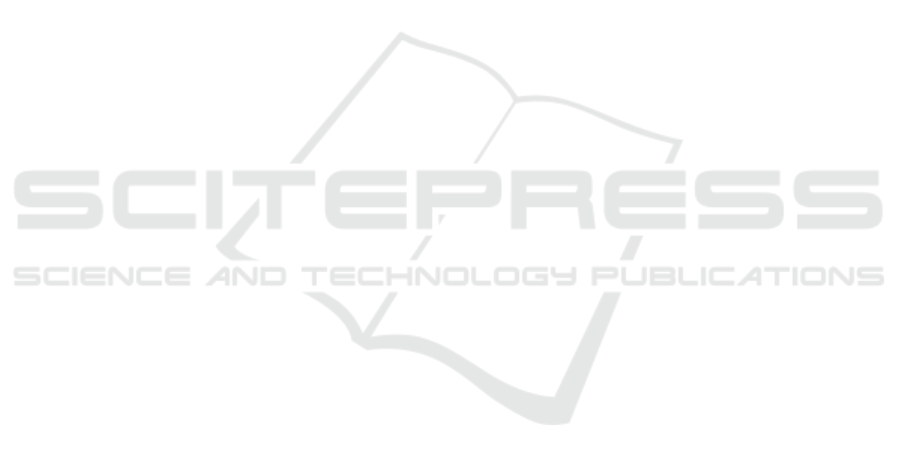
would allow for a more realistic simulation with ma-
jor impact specifically on the aerial environment,
where the 2D representation used in this work is a
major simplification. Another necessary step is the
deployment of the solution to real robots and real
environments, optimization and study of the chal-
lenges associated with it. The biggest difficulty for
the controller appeared to be on the aerial environ-
ment, specifically the wind gusts, that the controller
had difficulty in compensating. Future work could
also reside in optimizing this controller for better re-
sults in the different environments. For example, giv-
ing the controller access to a sensor that detects wind
gusts could help the robot compensate them and boost
the performance on the aerial environment.
ACKNOWLEDGMENTS
This work was partly funded through national funds
by FCT Fundac¸
˜
ao para a Ci
ˆ
encia e Tecnologia, I.P.
under projects UIDBEEA500082020 (Instituto de
Telecomunicac¸
˜
oes) and UIDB044662020 (ISTAR).
REFERENCES
Ahmad, A., Nascimento, T., Conceicao, A. G. S., Moreira,
A. P., and Lima, P. (2013). Perception-driven multi-
robot formation control. In Proceedings - IEEE In-
ternational Conference on Robotics and Automation,
pages 1851–1856.
Angelo Cangelosi, Domenico Parisi (1994). The touch sen-
sitive behavior of caenorhabditis elegans: A simula-
tion approach using neural networks. Technical Re-
port May, Institute of Psychology C.N.R. - Rome.
Chen, J. and Wermter, S. (1998). Continuous Time Re-
current Neural Networks for Grammatical Induction.
In International Conference on Artificial Neural Net-
works,1998, pages 381–386.
Cheviron, T., Plestan, F., and Chriette, A. (2009). A robust
guidance and control scheme of an autonomous scale
helicopter in presence of wind gusts. International
Journal of Control, 82(12):2206–2220.
Cliff, D., Husbands, P., and Harvey, I. (1993). Evolving
visually guided robots. In Proceedings of the Second
International Conference on Simulation of Adaptive
Behavior (SAB), pages 374–383.
Duarte, M., Costa, V., Gomes, J., Rodrigues, T., Silva, F.,
Oliveira, S. M., and Christensen, A. L. (2016). Evolu-
tion of collective behaviors for a real swarm of aquatic
surface robots. PLoS ONE, 11(3):1–25.
Duarte, M., Oliveira, S., and Christensen, A. L. (2012).
Hierarchical evolution of robotic controllers for com-
plex tasks. In 2012 IEEE International Conference on
Development and Learning and Epigenetic Robotics,
ICDL 2012.
Duarte, M., Silva, F., Rodrigues, T., Oliveira, S. M., and
Christensen, A. L. (2014). {JBotEvolver}: A versatile
simulation platform for evolutionary robotics. In Pro-
ceedings of the International Conference on the Syn-
thesis & Simulation of Living Systems (ALIFE), pages
210–211.
Eaton, M. (2007). Evolutionary humanoid robotics: past,
present and future. Lecture Notes in Computer Sci-
ence, 4850:42.
Fitzpatrick, P. M. (2003). Perception and perspective in
robotics. In Proceedings of the 25th Annual Confer-
ence of the Cognitive Science Society.
Hartland, C. and Bred
`
eche, N. (2006). Evolution-
ary robotics, anticipation and the reality gap. In
2006 IEEE International Conference on Robotics and
Biomimetics, ROBIO 2006, pages 1640–1645.
Jakobi, N., Husbands, P., and Harvey, I. (1995). Noise and
the Reality Gap: The Use of Simulation in Evolution-
ary Robotics. Lecture Notes in Computer Science,
929:704–720.
Jim, K., Giles, C. L., and Horne, B. G. (1995). Effects of
Noise on Convergence and Generalization in Recur-
rent Networks. In Advances in Neural Information
Processing Systems (NIPS) 7, page 649.
Kim, S.-W., Qin, B., Chong, Z. J., Shen, X., Liu, W., Ang,
M. H., Frazzoli, E., and Rus, D. (2015). Multivehicle
cooperative driving using cooperative perception: De-
sign and experimental validation. IEEE Transactions
on Intelligent Transportation Systems, 16.
Le, Q. V., Saxena, A., and Ng, A. Y. (2010). Active Percep-
tion : Interactive Manipulation for Improving Object
Detection. Technical report, Stanford.
Leonard, F., Martini, A., and Abba, G. (2012). Robust non-
linear controls of model-scale helicopters under lat-
eral and vertical wind gusts. In IEEE Transactions on
Control Systems Technology, pages 154–163.
Lewis, M. A., Fagg, A. H., and Solidum, A. (1992). Ge-
netic Programming Approach to the Construction of a
Neural Network for Control of a Walking Robot. In In
IEEE International Conference on Robotics and Au-
tomation, pages 2618–2623.
McCulloch, W. S. and Pitts, W. (1943). A logical calculus of
the ideas immanent in nervous activity. The Bulletin
of Mathematical Biophysics, 5(4):115–133.
Merino, L., Caballero, F., Mart
´
ınez-de Dios, J. R., Ferruz,
J., and Ollero, A. (2006). A cooperative perception
system for multiple UAVs: Application to automatic
detection of forest fires. Journal of Field Robotics,
23(3-4):165–184.
Pflimlin, J., Soueres, P., and Hamel, T. (2004). Hover-
ing flight stabilization in wind gusts for ducted fan
UAV. 2004 43rd IEEE Conference on Decision and
Control (CDC) (IEEE Cat. No.04CH37601), 4(Jan-
uary 2005):3491–3496.
Rodrigues, T., Duarte, M., Figueir
´
o, M., Costa, V., Oliveira,
S. M., and Christensen, A. L. (2015). Overcoming
limited onboard sensing in swarm robotics through
local communication. Lecture Notes in Computer
Science (including subseries Lecture Notes in Artifi-
ECTA 2023 - 15th International Conference on Evolutionary Computation Theory and Applications
276